Book
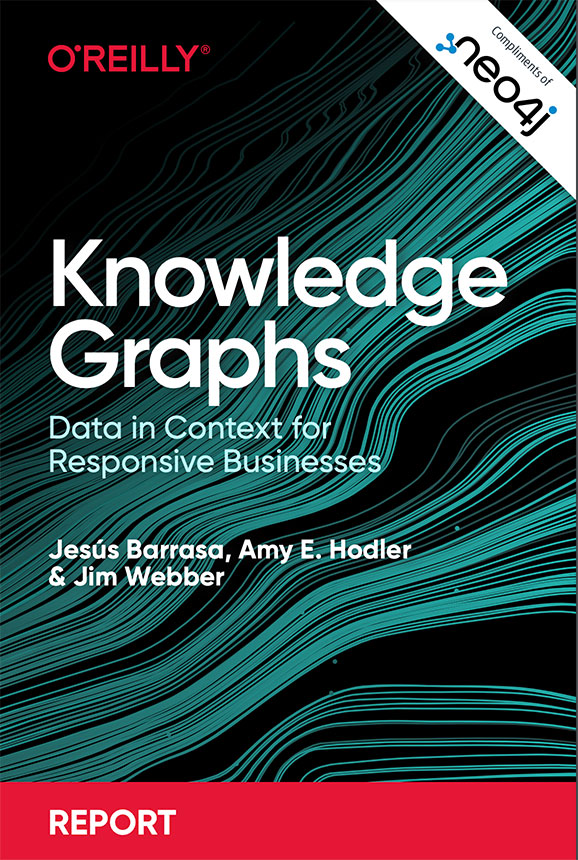
Knowledge Graphs: Data in Context for Responsive Businesses
Specs
By Jesús Barrasa, Amy E. Hodler, and Jim Webber
Publisher: O'Reilly Media
Summary
GAll (graph) data science and analytics start with a knowledge graph!
Despite their predictive power, most analytics and data science practices ignore relationships, the best predictors of behavior, because it has been historically challenging to process them at scale.
Instead of ignoring relationships, knowledge graphs incorporate them into analytics and machine learning (ML) workflows. In fact, graph analytics excel at finding the unobvious by processing patterns even when we don’t exactly know what to look for, while graph-based ML can predict how a graph might evolve.
A decisioning knowledge graph – a knowledge graph used for analytics, ML, or data science – surfaces trends in the data which can be used in several ways including:
- Human analysis for data science exploration and experimentation to answer previously intractable questions
- Graph queries, algorithms, and embeddings for better predictive feature engineering
- Further processing by downstream systems, like training ML models or knowledge graph completion, to predict missing data and relationships
- Combination with AI systems for contextual (unbiased) AI by determining your data provenance and through transparent data sourcing
In the book Knowledge Graphs: Data in Context for Responsive Businesses, we help data scientists and analysts understand what knowledge graphs are, how to build and use them for actioning and decisioning, and how they power innovations like AI, digital twins, data fabric, and more.
This book is a must-read for data professionals who truly want to understand their data – its lineage, transparency, relationships – and, through the deep context derived from a knowledge graph, build more accurate and unbiased predictions to drive intelligent business decisions.