How Previa Is Reimagining Healthcare
Alessandro Svensson and Sarah Johnnesson
Head of Neo4j Innovation Lab, Product Manager Doberman
18 min read
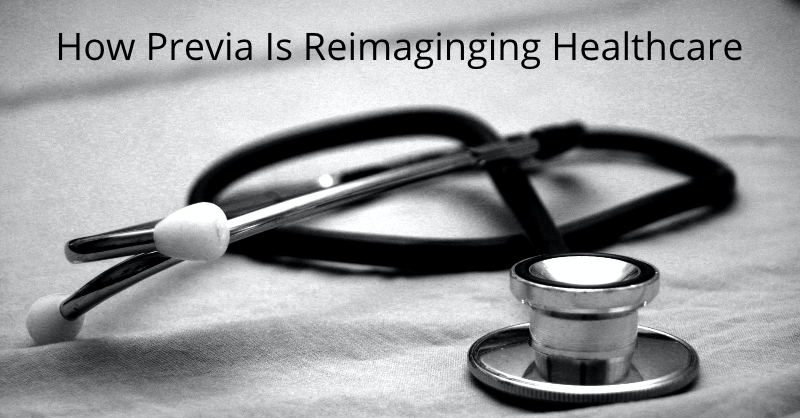
Editor’s Note: This presentation was given by Alessandro Svensson and Sarah Johnnesson at GraphConnect New York in September 2018.
Presentation Summary
Alessandro Svensson is the Head of Neo4j Innovation Labs within the U.S. He and Sarah Johnnesson of Previa discuss the impact of implementing graph thinking into the healthcare company, and the results following one year of their original Neo4j Innovation Lab
sprint in San Francisco.
Svensson first highlights what the Innovation Lab sprints include and how Neo4j encourages graph thinking for new customers. Usually, this is through generating and prototyping graph projects. Next, he highlights the expected outcomes of the sprint which is not to build perfect projects like many might expect. Instead, the expected outcome is to provide a deeper understanding of what thinking in graphs really means. The foundation for this methodology is laid out during the Innovation Lab sprint.
Companies come to adopt Neo4j by already being familiar with the open source software or the Neo4j Community Edition. Typically, when companies come to Neo4j, their developers or architects are aware that their problem could be solved by graphs. At this point, a company is ready for a a shift in mindset. They know that dealing with their processes, their data, their structures and their thinking is not yielding the results that they want. The Innovation Lab sprint aims to open thinking to what is possible when one starts thinking in graphs.
The Neo4j Innovation Lab methodology encourages customers to think about processes in a way that is connected as opposed to thinking of information as siloed and stale. Customers work on generating use cases and data modeling, they write large-scale problems on a whiteboard and then discover what pieces are significant.
Finally, at the end of the Innovation Lab, a lot of energy is put into prototyping and creating something truly usable.
Sarah Johnnesson is a long-time consultant at Previa. She recounts Previa’s Innovation Lab sprint tasked with preventing burnout. As a company that deals with the health and wellness of 1.1 million Swedes, Previa had a lot of work to do.
Previa’s work today consists of linking and capturing trends in health-related absences. This includes tracking conditions, work environments, managers and symptoms to treat existing conditions and better predict future ones.
Previa’s work since customer 360 views has come a long way since it’s initial Innovation Lab sprint in San Francisco and many companies have engaged in labs since. The typical audience for such a lab generally contains members from a range of teams and varying skill sets.
The end result is always the same. The methodology that Neo4j uses impresses customers with its speed and their ability to deeply understand complex problems in as little as a couple of days. Many companies find that they achieve more in three and a half days, using the Neo4j methodology, than they have done on their own in a year.
Full Presentation
Alessandro Svensson: My name is Alessandro Svensson. I’m the head of Neo4j Innovation Lab and I’m hosting this session with Sarah Johnnesson. There are two main topics we are going to cover in this talk.
The first thing I’m going to introduce is the Neo4j Innovation Lab, which is something we’ve been working on for just over a year. Previa was actually our first case study from this work within the Innovation Lab, starting in 2017.
Sarah is going to talk about how Previa reimagined their business, preventive care, with connected data. I’m going to talk a little bit about our methodology and the way we approach our work within the Innovation Lab.
The Innovation Lab was introduced in 2017. We did our first pilot project in June of 2017, that was the product we did together with Sarah’s team, Previa.
What We Do
Our whole purpose is to help companies accelerate innovation and transformation through graph thinking.
How We Do It
We encourage this graph thinking by generating and prototyping graph projects with our customers and prospects. These Innovation Labs are a collaborative effort.
Expected Outcome
The expected outcome of this is not to build perfect projects. You will see this when I go through the methodology. Instead, the outcome is more to provide a deep understanding of what it means to think in graphs. We want to deliver a thorough understanding of the possibilities in innovation and transformation that is enabled by adopting Neo4j. We want to inspire teams to start thinking about their data as connected.
Methodology
We host a three and a half day workshop which we call the Innovation Lab sprints.
How Companies Adopt Neo4j
First, I want to kick off my talk with how companies adopt Neo4j and where this fits in the adoption cycle. There are basically three ways that this happens. First, a company gets introduced to Neo4j.
This introduction could be facilitated by being an open source software, or being familiar with our community edition. Typically, developers or architects are working on a problem that they know graphs would be a good solution for.
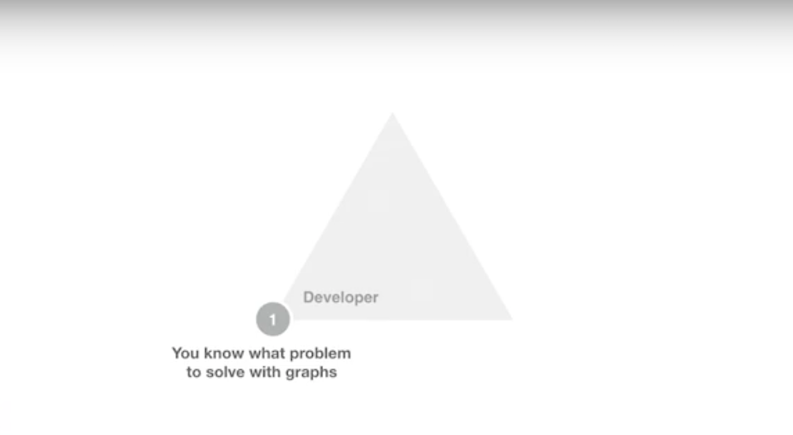
We don’t require an in-depth understanding of the technology. Problems are often very specific. Your problem could be that your relational database just doesn’t perform in the way that you want it to. Then, when you start looking at graph databases, you download the community version of Neo4j, you try it out on your own PSEs and so on. That’s how we operated for almost a decade when customers came to us with their problems.
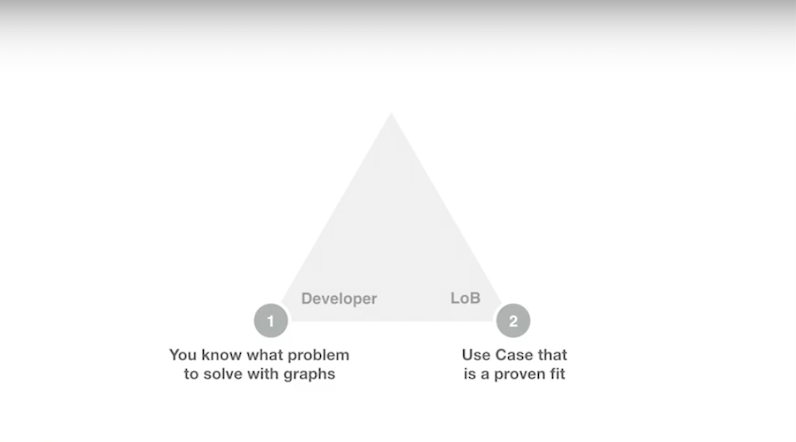
If you’re working as a risk manager, in fraud detection or in recommendations, you might have heard that graphs are a good technology to deal with area-specific challenges. There are a ton of benchmarks and white papers, making it pretty easy to get familiar with how graphs fit specific use cases.
We’ve seen graph technology evolve and gain an increasing footprint in organizations. Connected data is a strategic fit for transformation, it’s also very prevalent.
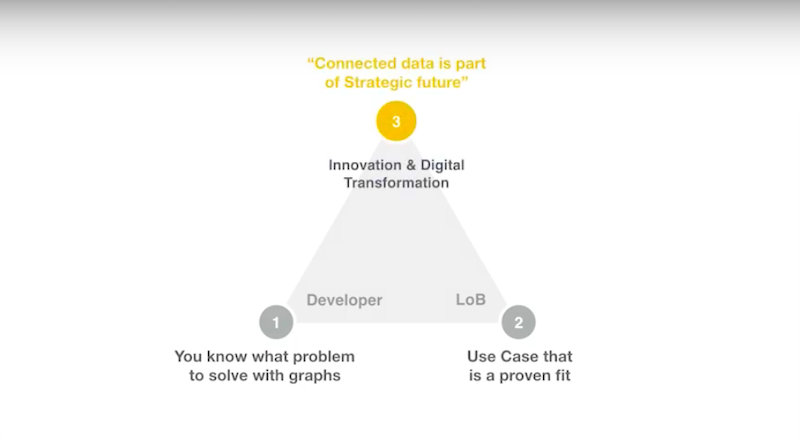
The innovation teams or leadership teams might not have a problem in mind in the first place. The problem that they do have in mind might not even fit the mold of a specific known use case. That’s the realm where the Innovation Lab works.
We don’t work so much with known problems or well-defined use cases. We actually look at the use case that we would call the digital transformation. The good thing with known use cases is, though they could be very complicated, the input and the output are still known. You know what you want to achieve within the realm of a use case.
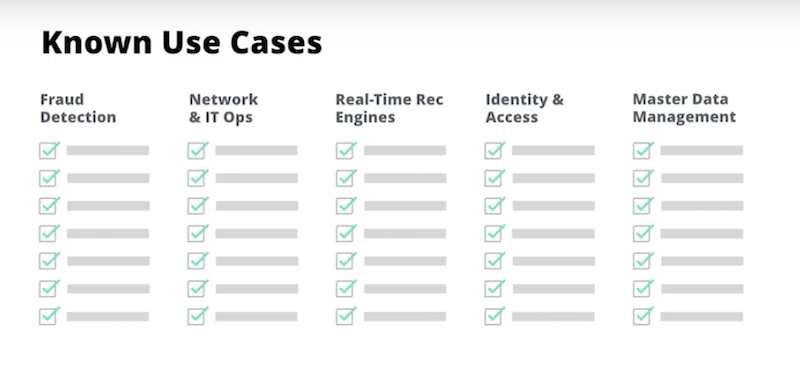
Digital transformation is different because it might be the future roadmap of a company or product.
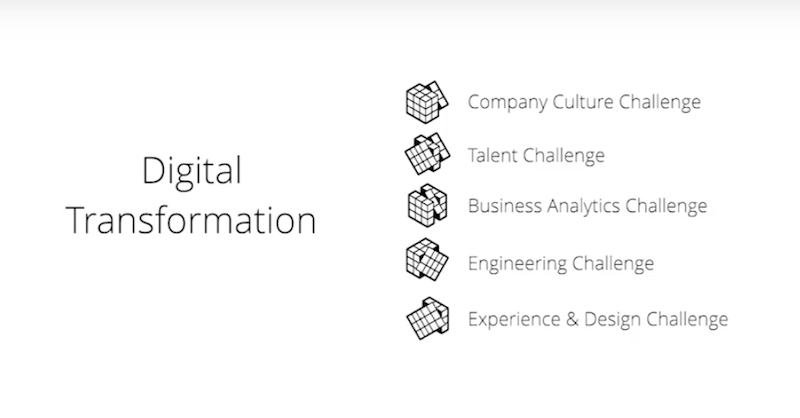
Digital transformation requires more of an inquiry and an exploration around what’s possible. If you look at digital transformation as a use case, it is not a specific set of items to check. Instead, digital transformation is a set of really large challenges.
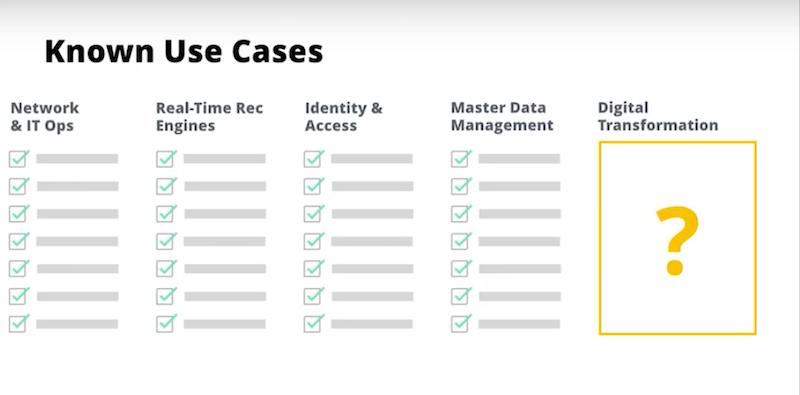
These challenges could include company culture, a talent challenge – maybe you’re not able to recruit the right people. These challenges could be in business analytics, engineering, design, experience and so on. These are sets of really complicated problems. What we explore in the Innovation Lab is that there could be a connected data narrative of all of these problems. That’s what our process is based on.
A Paradigm Shift in Mindset
In order to achieve this, the first thing we look at is this paradigm shift in mindset. Many companies that we work with are stuck in this paradigm of having their processes, their data, their structures, and their thinking based on these siloed, solution-specific tasks.
They’re so stuck in this mindset that it becomes formative. If you’ve been working in an environment that has this kind of structure underneath, it’s hard to think outside the box and have this cross-pollination.
The first thing we do is start looking to see what’s possible in the mindset. We want to see what opens up if you start relating to your data and processes in the way that stuff is actually connected. This also happens to be data modeling for the graph.
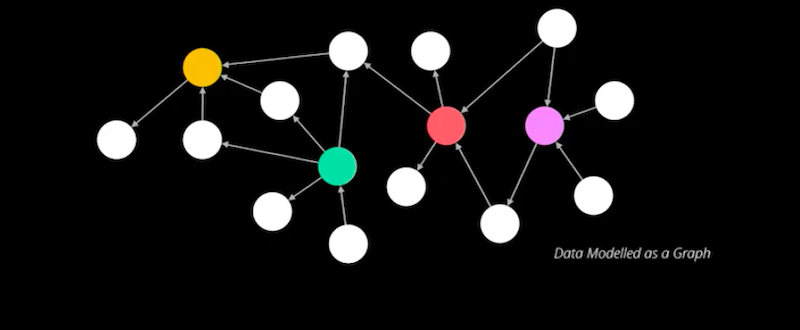
Your processes will always be mirrored in some sort of data structure. This is a very common problem. We actually use this concept as a mantra.
Whatever processes you have on stage or that you deal with day-to-day, will always be represented here.
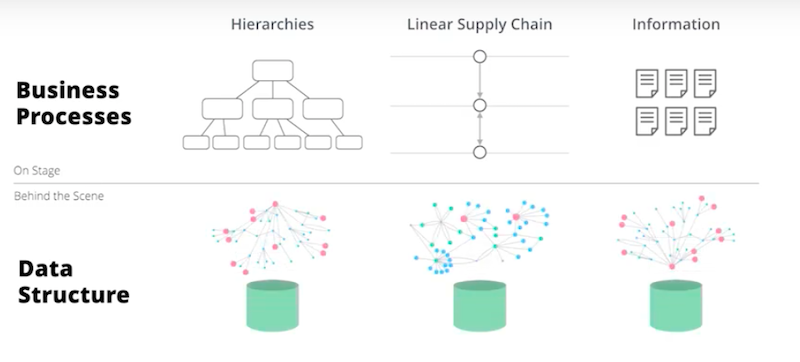
Conceptually, if you think about a process that would look like a hierarchy, like a CRM tool or a supply chain, that is very linear information that is stored in a certain way. There’s always a representation in data and this happens whether you think about it or not. What we do in the Innovation Lab is reimagine if we are able to change the underlying data structures.
Thinking about hierarchy, that could actually be represented more as an organization. Which obviously changed a lot, how you would approach a CRM problem for example.
A connected supply chain could start taking shape as a dynamic supply chain, which opens up for those types of application. There’s a lot of talk about artificial intelligence, knowledge graphs are one of the ways we think about information and storing information in a certain way.
You see, business processes and your data structure will always be in a dance and you can’t have one without the other. If you want to strategically change your processes, but you don’t change your underlying foundations, you will always operate at a deficit.
The Neo4j Innovation Lab Methodology
I’m going to talk about our methodology, and how we approach these exercises with customers. We do this to propel a way of thinking about processes. We want customers to think about processes in a way that is connected as opposed to siloed and stale.
There are three components to an Innovation Lab; the business processes, the technology and the methodology. The technology is data science which is graphs in our case. The methodology is very heavily influenced by design thinking.
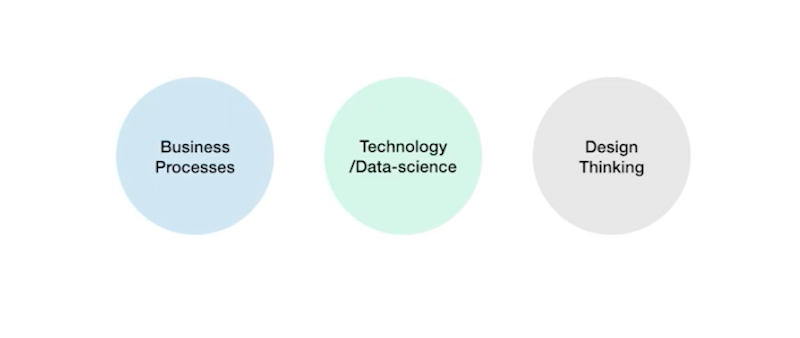
The process is input from the customer. For example, if we work with a bank, the team from that bank will be the experts in that business domain. We provide the technology, the data science and the expertise in graph. In the Innovation Lab, we think a lot about the methodology and how to wrap this around exercises that will accelerate this kind of thinking.
When we have this as a combination, like in the middle, is really where we see that we have our sweet spot.
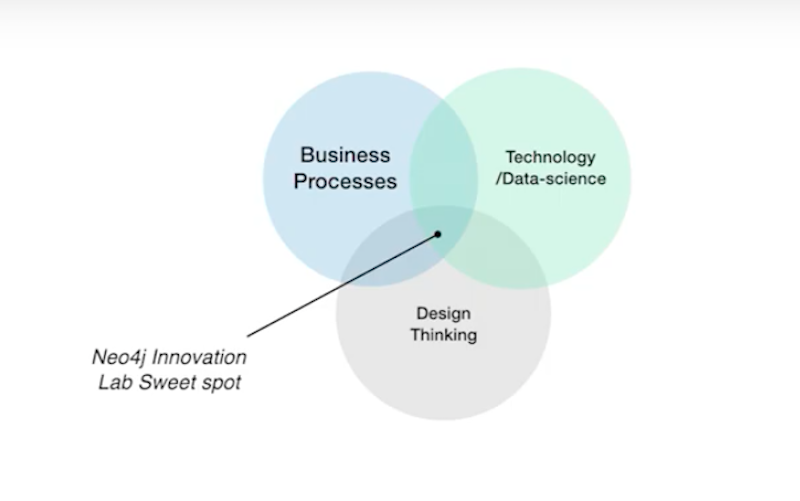
This is where we are the most effective and what it looks like as a process.
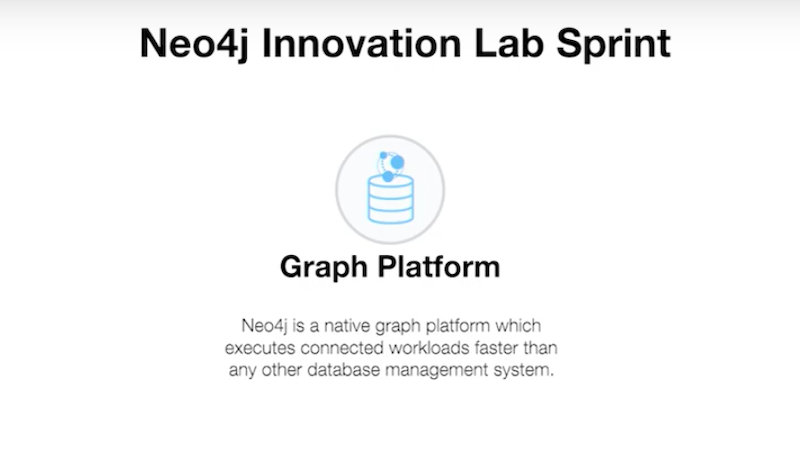
Everything that we do at Neo4j revolves around the graph platform, starting with our technology. The difference between a lab and a more technical exercise, is that we focus as much time on the graph mindset.
The third component is that we also want the customer to experience the difference with something tangible.
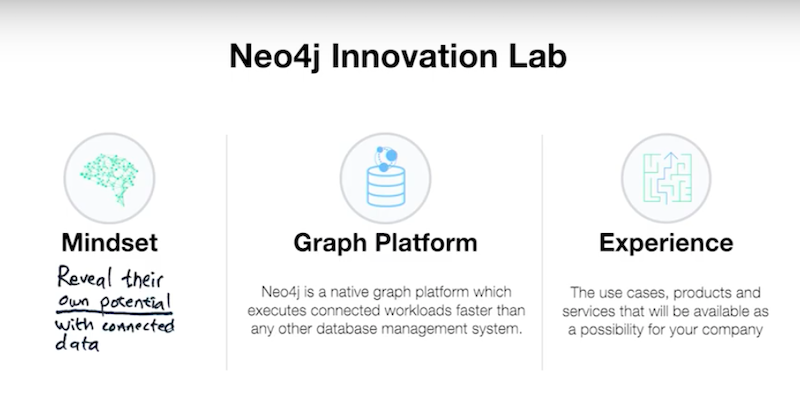
The mindset reveals your own potential with connected data. This is why we encourage users to make that jump and experience that paradigm shift in how they think.
Then, you see it materialize in Neo4j. The experience occurs through those technical exercises where we model, we ingest data and so on.
At the end we experience the difference with something tangible. This means that we build a prototype that you are able to touch, feel and interact with.
We’ve seen that when this happens in a sequence,it becomes almost cathartic. That is because you have it happen in a very short amount of time. You go from never having heard of graph technology to experiencing what’s possible within your company or team by having gone through this process.
The way we solve this is through the Innovation Sprint, which is a three and a half day exercise done with customers.
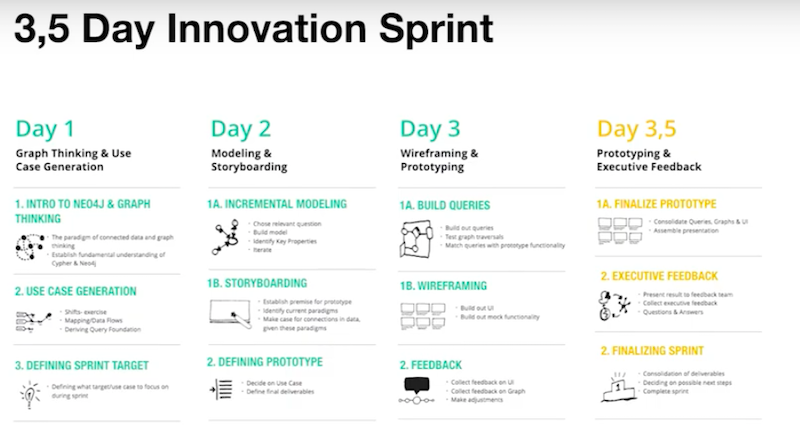
Above is the format of a pretty standard design sprint. What makes this unique is that we’re not agnostic when it comes to technology. We only focus on graphs and then that’s what we explore.
Generating Use Cases
Here’s some pictures to get a vibe for what this looks like in real life. This is a very intense session where we work for three and a half days. The first part is all about generating use cases which Neo4j based on the model to be intuitive. It’s a very analog exercise.
Data Modeling
The next part is the data modeling and this is also one of the benefits of working with Neo4j. We are able to create large-scale problems just on a whiteboard and really discover what’s significant.
We ask ourselves “What would be a significant graph problem to solve for a specific company?”
Then we begin to see what this will look like as a graph.
As these days progress we go into more detail. We start to identify data sources, ingest sample data and so on. We get to the point where we craft algorithms and pretty advanced queries.
Prototyping
At the end of the Innovation Lab, we focus a lot on prototyping. We put a lot of energy into making them beautiful and represent something that you could use.
Sarah Johnnesson from Previa has been gracious to present her result as a participant of this Innovation Lab sprint.
Previa’s Innovation Lab Sprint
Sarah Johnnesson: I’m going to talk about the Innovation Lab sprint, Previa did in San Francisco. We were together with Alessando Svensson. This project was about preventing burnout.
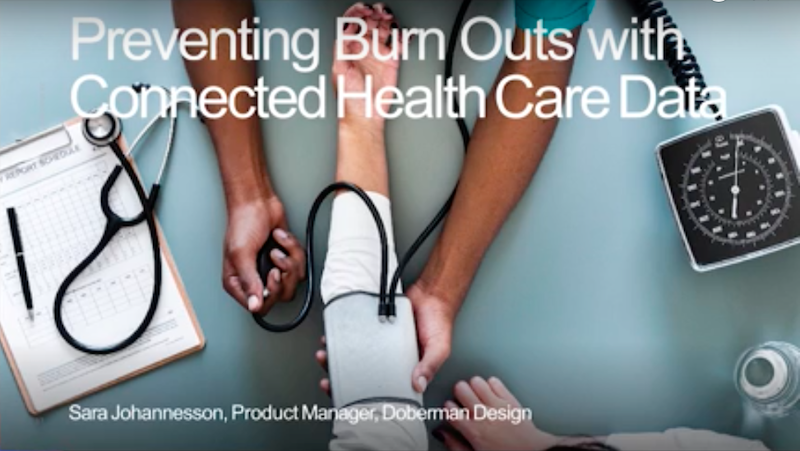
Previa is an occupational healthcare company that is legislated in Sweden. In Sweden, companies have to offer healthcare to their employees. That could be everything from preventive care, therapy, health exams and establishing a healthy work environment.
Previa is one of the largest companies in Sweden. They are also offering a service that is available to an employee whenever they are sick. They are able to call Previa directly, and tell Previa that they are ill and why they are not going to work. From there, Previa will send the message to your boss and let them know the details.
This is what our service looked like a year ago.
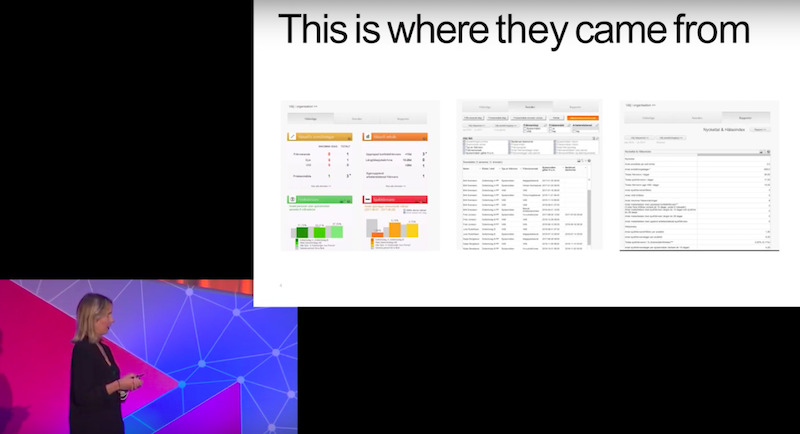
As you are able to see, it’s very messy. It’s hard to find all of the clients, and it appears to be a business to business company.
Soon all the employers were asking questions like, “You’re meeting all my employers all day, what are you able to find out about them? Why are they ill? What do I need to do?
How should I make sure that they are a healthy workforce? “
Previa was not in a good place to answer these questions because the data was not structured in such a way. This was a problem.
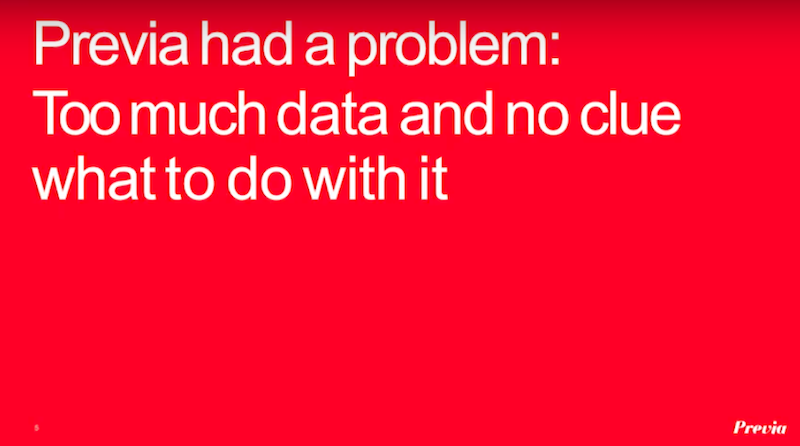
When I first met Previa, my reaction was “Oh my God, you have so much data and you’re not doing anything with it.” Previa felt they had tried a few times but they really didn’t know what to do anymore. They had so much data that it was frustrating.
Luckily, we managed to convince them that we should fly to San Francisco and do the Innovation Lab sprint with Neo4j.
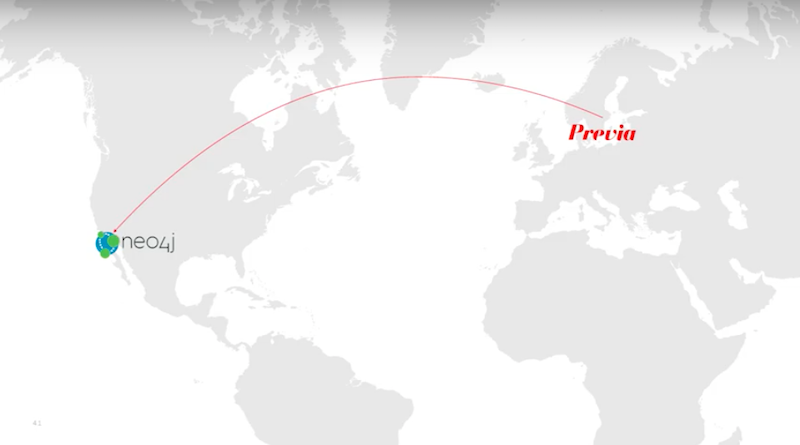
I took a few of my team members with me.
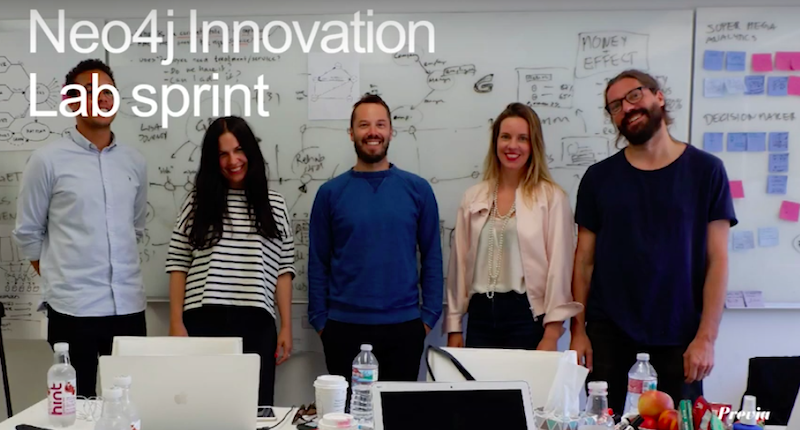
Five people were in a room for a week. We were very happy as you are able to see. It’s fun to be in San Francisco and to do the lab.
The sprint required Previa to make sure that their business case was going to be stronger and to be able to answer their client’s needs and questions. They wanted to provide the best healthcare they could and make sure that they gave the right recommendations. This was not only to their clients, but also to their own doctors, physiotherapists, therapists and psychologists.
This is where Previa was.
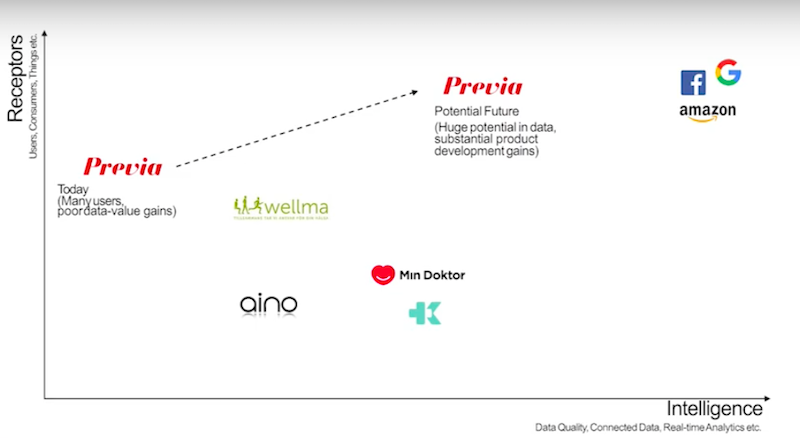
They have many many users, but the date was not intelligent. Previa needed to take a leap and make sure that they were better than all the other startups coming. Why would customers want to go to this older company when there are cool startups that understand data from the start.
We’re going to look at what kind of data we had to work with.
Sweden is a fairly small country. We are 10 million people and approximately five million people are working. Previa has 7500 companies as clients, that equals 1.1 million Swedish employees.
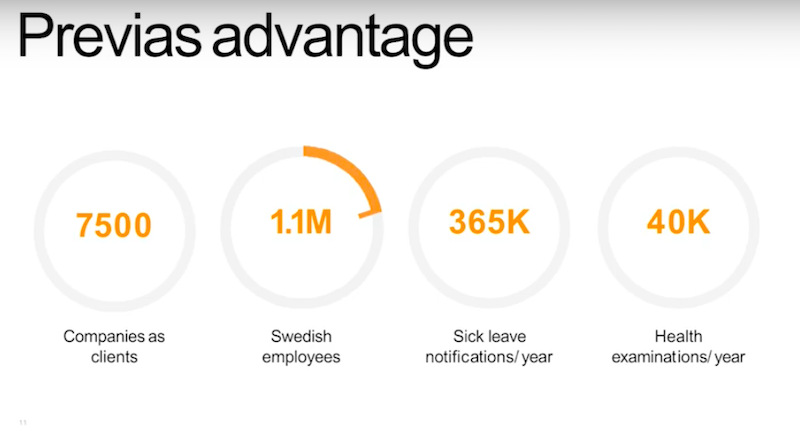
Previa has sick leave notifications. There are about 400,000 sick leave notifications per year and they’re doing 40,000 healthcare exams.
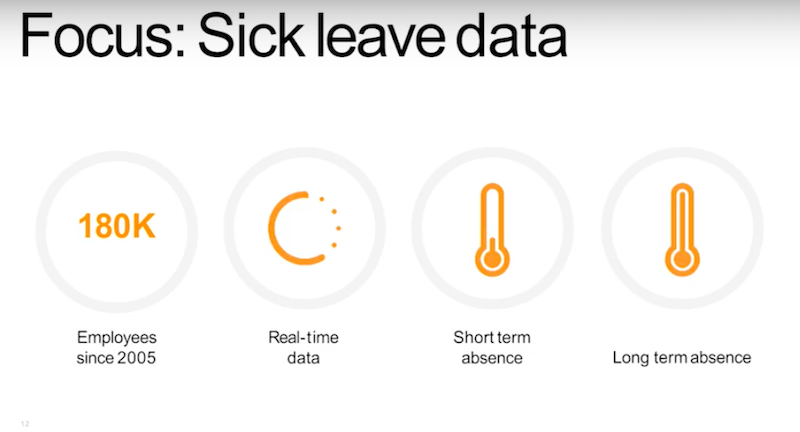
For this sprint, we had a focus on the sick leave data. It’s real-time data from 180,000 employees since 2005. The focus was on short-term and long-term absences. It was also noted if leave had something to do with the employees work.
After connecting the data and going through and understanding what Previa is and what kind of data we have, we created our whiteboard model in San Francisco.
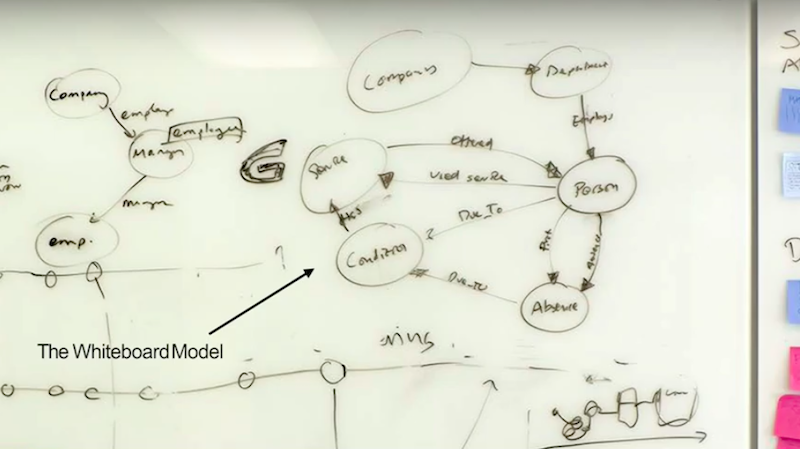
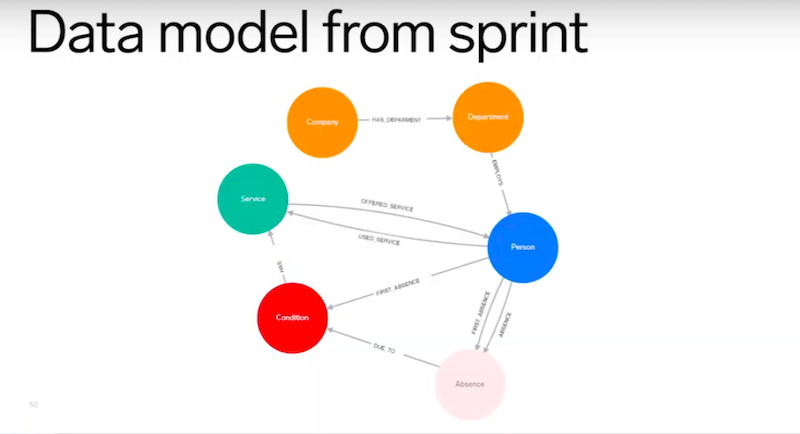
This is not far from the model that we use today.
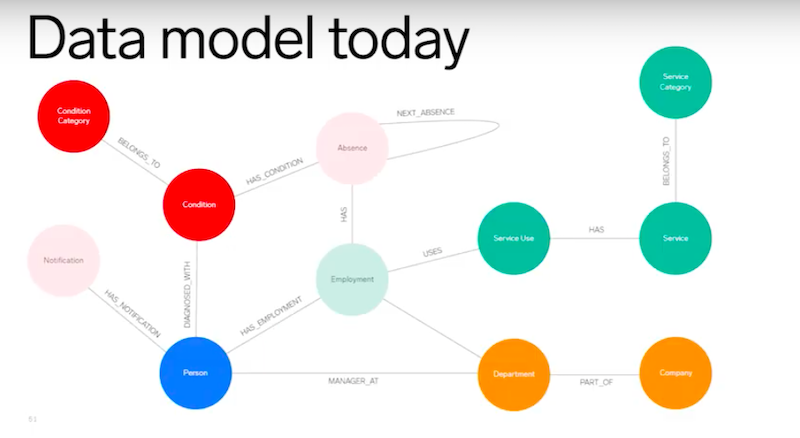
Previa’s Work Today
I’m going to walk you through a bit about what we’re actually doing.
We’re focusing on people’s individual healthcare. We have a person, that person is employed at a company and the absence is tied to the employment. Absences require a reason to be ill, so that’s why we’re tying the condition to both the absence and the person. This is done to make sure that we are able to follow the person no matter where they work. We are also able to see if the condition is tied to the place of employment.
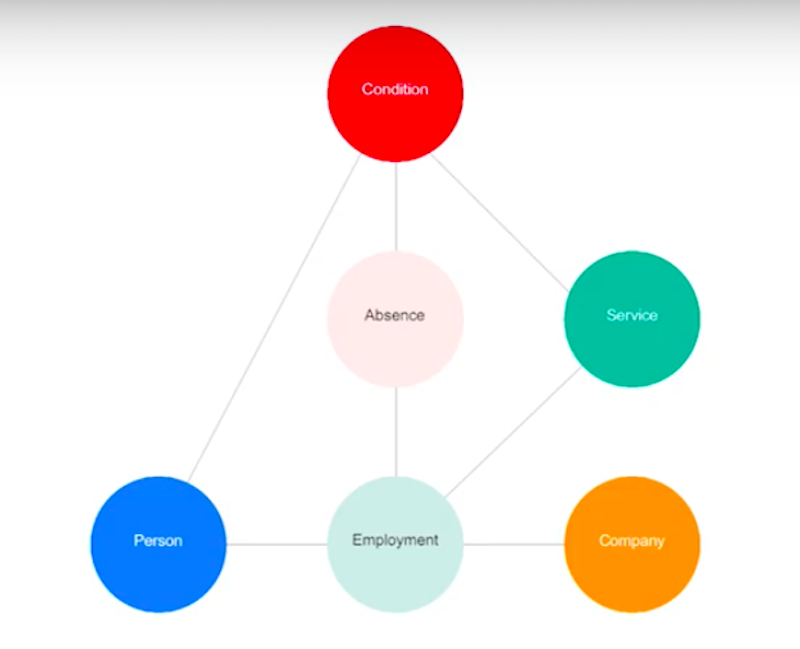
Previa is offering services to hopefully cure the condition. If a person is using that service, we also tie that to the employment. There may be a pattern related to the place of employment.
With this model, we are able to find patterns that had previously been hidden. There might be trends that we’ve known were there, but we were unable to find. With Neo4j, we are now able to do that.
I’m going to give you another example. We have a superstar skier. He is employed as a skier at the cross country team.
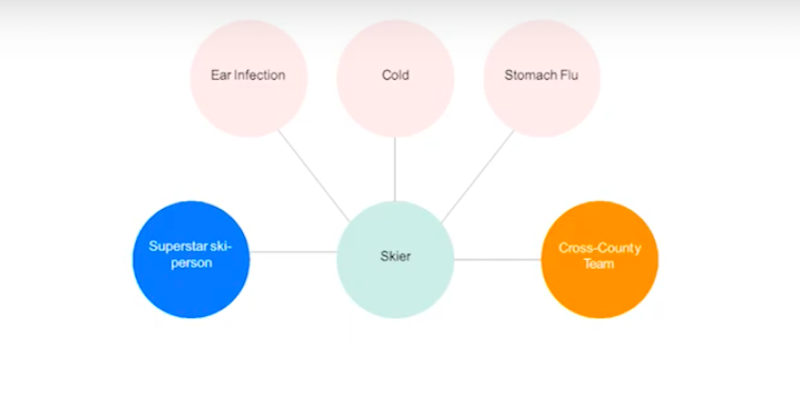
The superstar skier gets an ear infection, a cold and the stomach flu.
Next, we have a superstar politician. He is the prime minister. The superstar politician gets an ear infection, a cold and a stomach flu.
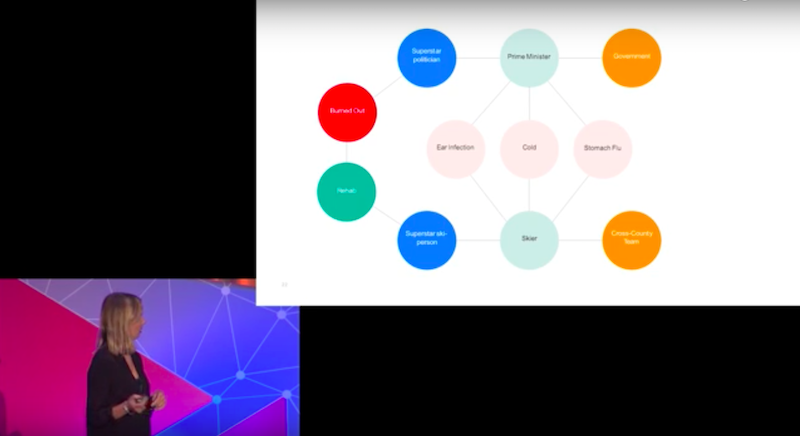
What happened to the superstar politician? He got burned out. We want to prevent our superstar skier from getting burnt out as well.
We know that our rehab is working with all three of these conditions. We are able to offer the superstar skier the preventive care we know he needs.
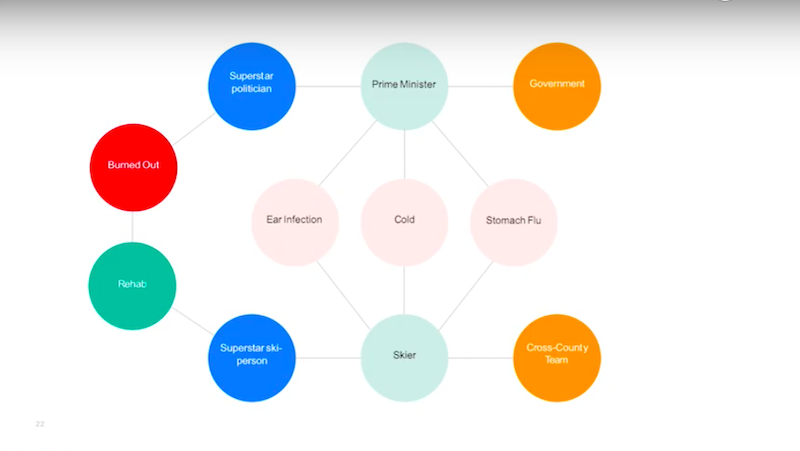
We are also able to find other patterns that don’t really have to do with the individual.
Here we have a company with two departments. We are able to see on one side that we have three employees with a lot of absences. On the other side we have employees that don’t have as many absences as the others.
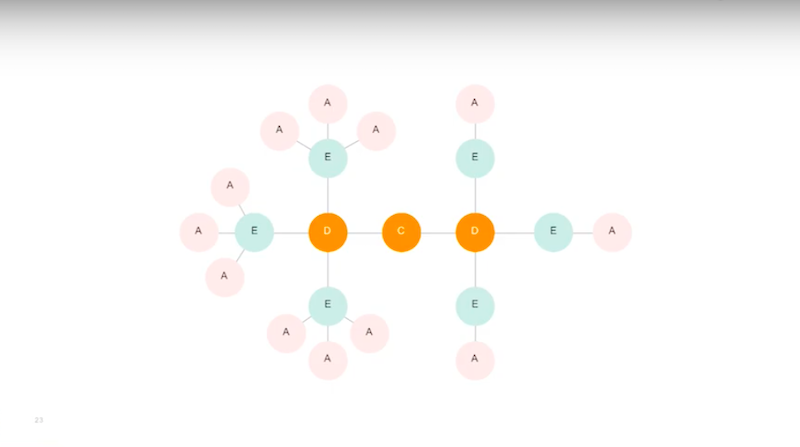
Here we are able to look for other things, maybe it’s something in the environment. What about the air? What about the ergonomics? It could be something on the structural level. These employees could have a bad boss. It is super interesting to look at those patterns that we couldn’t really find before.
We are building a new tool with Previa for a healthier work life.
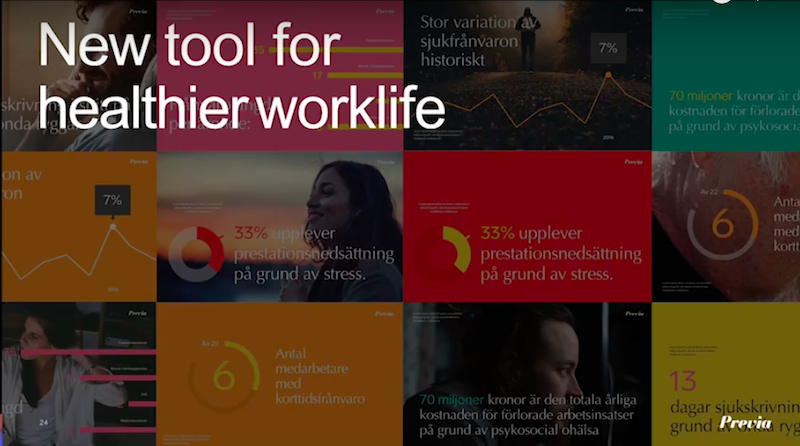
This is the prototype that we did in San Francisco.
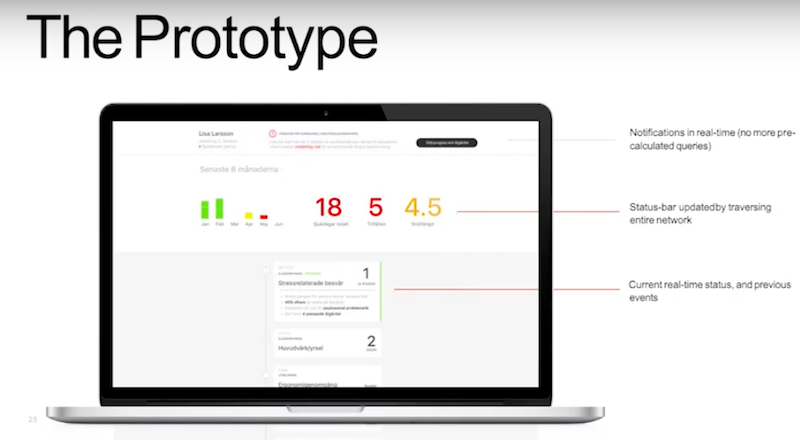
We wanted to make sure that everyone, an employee or a boss, has access to a good overview about how their workforce is doing. It also includes if there is something that you need to act on. This was a simple model of how to use the data.
This is what we have today, everything is in Swedish.
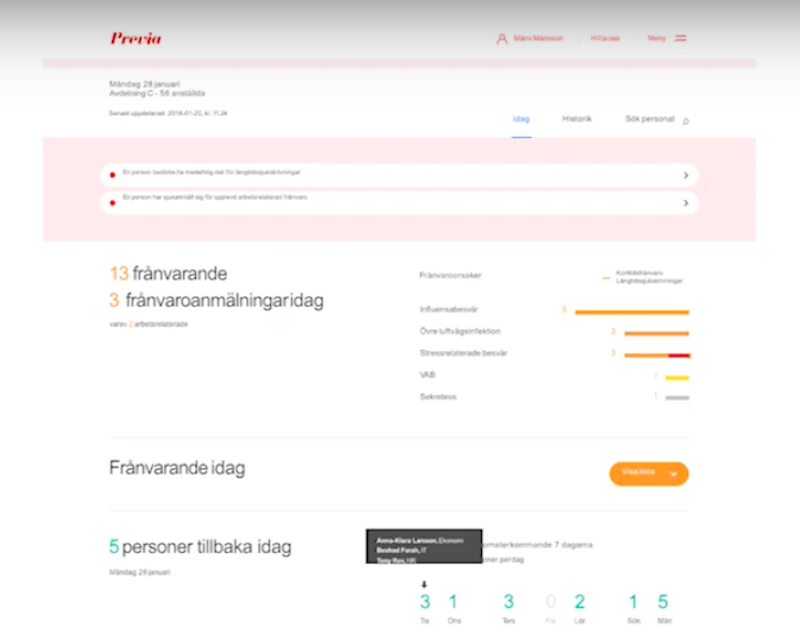
The numbers are also placeholders.
This is what I need to know as a boss. I need to know if there’s something that will require my immediate attention. If there is someone out sick, maybe I need to call in someone else to work. If someone has been out sick for three times during the last six months, that increases the risk of being long term sick listed with 50%.
This alerts me to check on that person. Maybe something is wrong and I am able to help them in some way. That’s something employers really want to do. They want to make sure that people get the help they need.
Below you are able to see absences, how many people are coming back to work and sick leave history. You are able to see if someone is using a service right now.
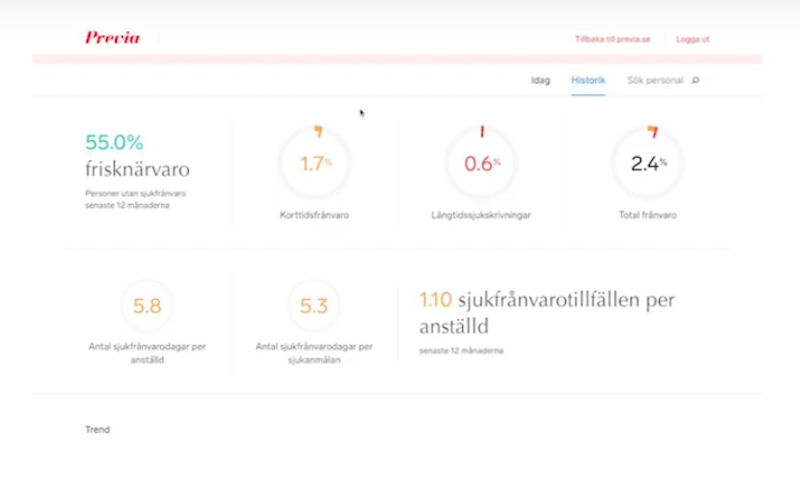
This is an overview covering the past six months over all your department. You are able to see how you’re doing and if there’s something you need to deal with on a department level.
Let’s look at the architecture. I think this is interesting because Previa is an old company where they have all the data structured in silos.
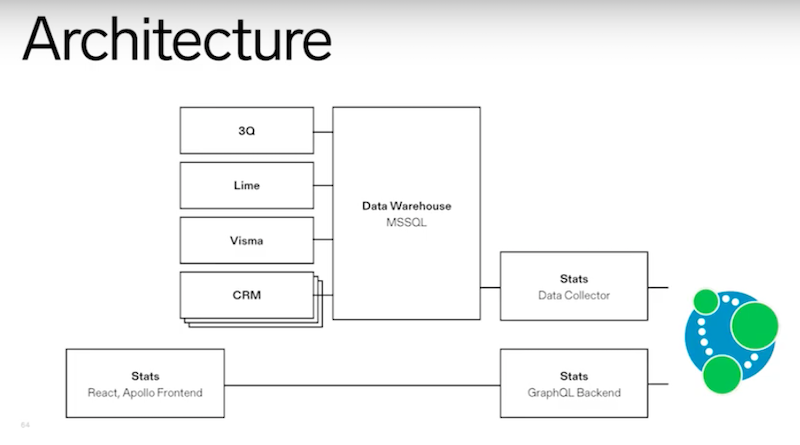
Previa is moving the data to the data warehouse so that we could build on top of that with Neo4j. That means Previa could change all their old systems, but they don’t have to do that to move forward. They’re moving forward and keeping pace.
These are some examples of data sources that we used from the start for the prototype.
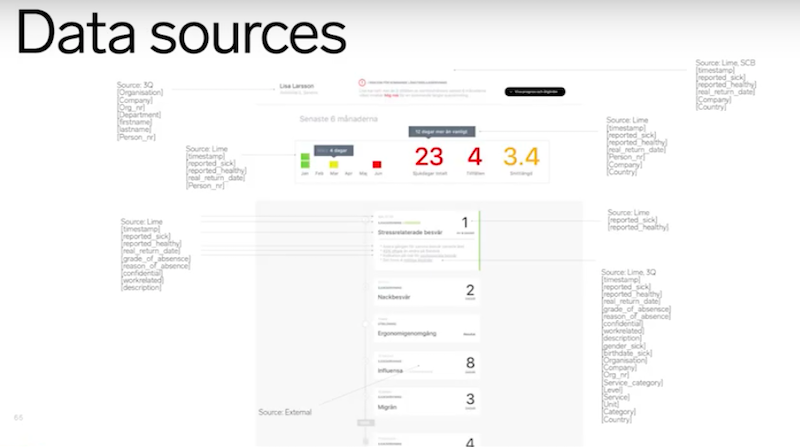
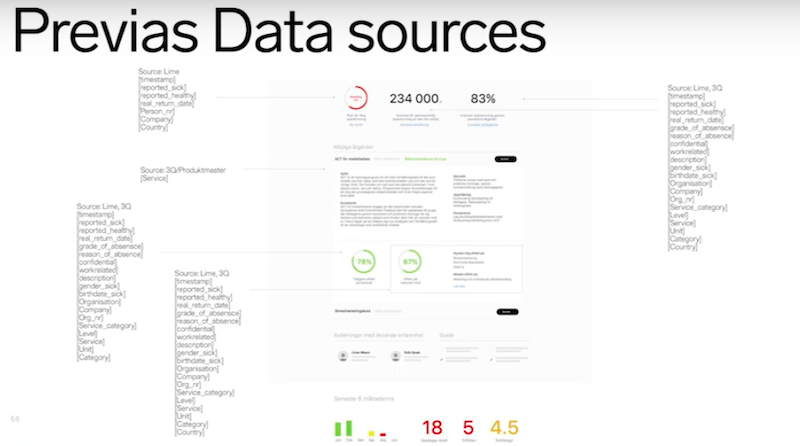
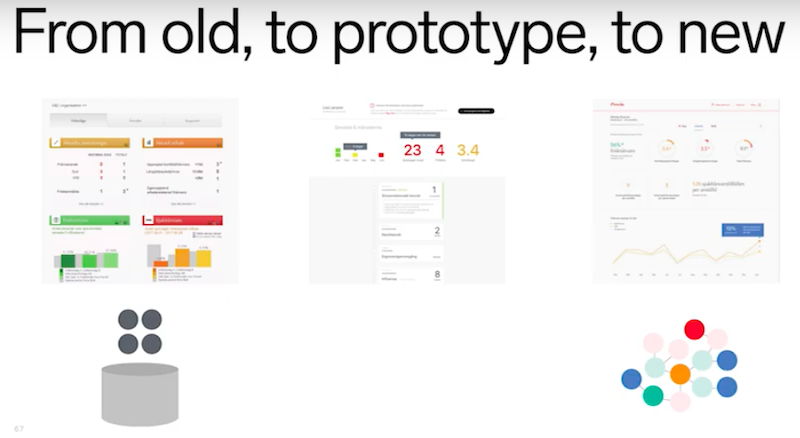
This is where we’ve taken Previa. We’ve come from the very old structure, to the prototype and then to a new tool that’s helping the Swedish workforce get healthier. We’re looking forward to finding more patterns that were previously hidden.
Some of the learnings that we had from doing the sprint was understanding how much power this prototype holds.
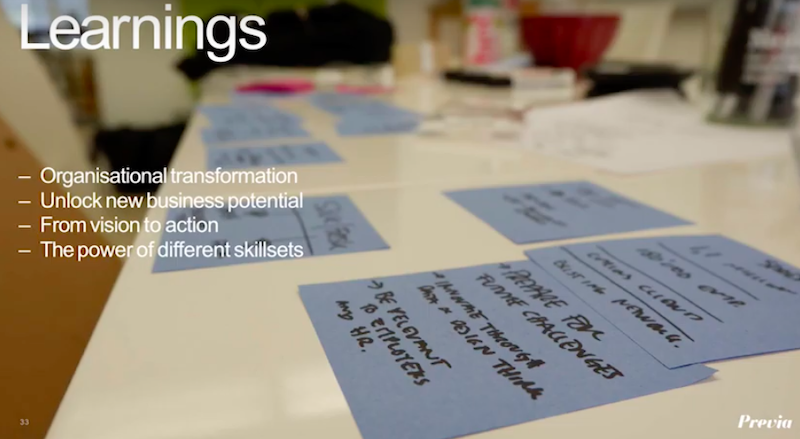
Graph technology motivated Previa to undergo actual change. We knew that we needed to change but we didn’t really know how to do it. Neo4j was a great tool for that.
Neo4j also unlocked a lot of business potential. Previa knew that we had something good, but we couldn’t really see it. When higher-ups saw the prototype, they were excited to say that we can do all of that. The expansion never stopped, which was awesome. Neo4j took us from vision to action.
It’s so easy to say that you want to do something and be visionary, but you don’t really know how. The Innovation Lab is hands-on. Another strength was the power of having different skill sets in the room. When we were in San Francisco, we had data scientists, engineers, designers, UX team members and myself. I represented Previa, because I’ve been working with them for a while.
Getting Started
Alessandro Svensson: It’s beautiful to see when companies go from the prototyping stage. As far as the Innovation Lab is concerned, our engagement stops after those three and a half days. Previa is now a year post the lab and is in production with a tool that is really changing their company.
Previa has now gone from being a very old legacy company that was under pretty huge pressure from all of the healthcare funding to a major competitor with the newer healthcare startups.
On a final note, I want to share how this works, how you could participate in this brand or how your company can engage with us in this. It’s pretty straightforward.
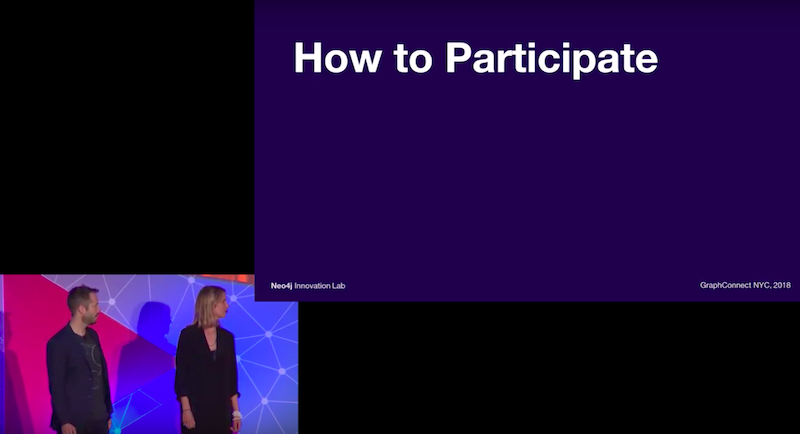
The Anatomy of an Innovation Lab
An Innovation Lab contains three parts.
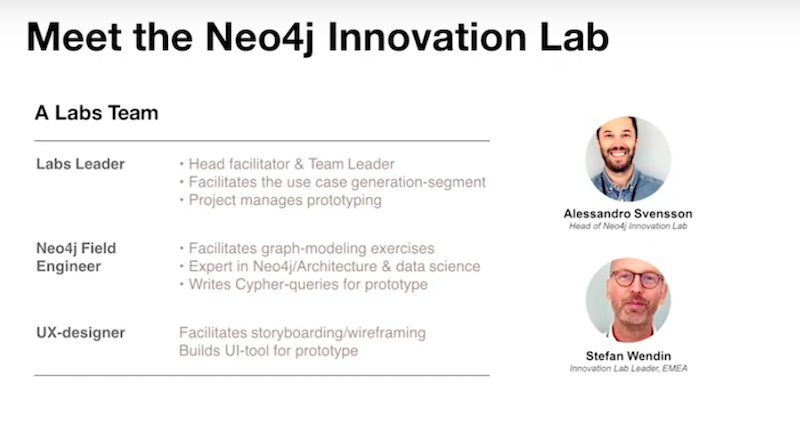
First, there is the lab leader which is a facilitator role. We always have a Neo4j field engineer present, who is skilled in both the Neo4j architecture and also data science. The Neo4j data science and UX design comes in during prototyping and wireframing.
The organization for the Innovation Lab has two heads of lab. I’m one of them, I am based in the U.S., and we have Stefan Wendin who covers Europe.
The Typical Audience
The typical audience for one of our sprints is not necessarily an innovation team.
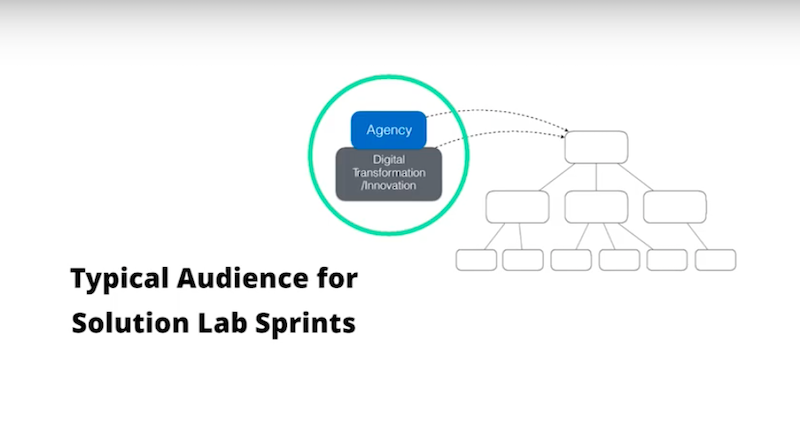
We’ve seen a lot of companies have the sort of team that sits outside the complexities of the normal day-to-day. They have a mandate to work on this kind of project and not be stopped by the slowness of their organization.
Typically, they are called like the transformation team or innovation team or it could be a specific group that is just summoned for the occasion. Often there’s also a partner agency connected to it. It could be like McKinsey to help you with types of design.
The important thing is that these teams are able to take many shapes and forms. Teams are always able to think outside the box.
Across the testimonials we’ve had, the methodology never changes. We work with heavy industry, banks, media companies, healthcare companies and so on. One of the things that stands out is the feedback concerning the speed of our processes. Customers really like that it’s fast. They get a deep understanding of their data and quickly. When data comprehension comes quickly among a room of ranging skilled professionals, the production level and the outcome soars.
That captures the benefit with this approach and this is something that we see a lot in Neo4j. One of the biggest challenges companies face, is not so much the adoption of Neo4j. That is because once you get going, it’s actually quite fast. Instead, I would say the validation of a technology is really what takes time.
We’ve seen companies spend eighteen months just validating if this tech is right for them. Depending on the disruption that goes on in your industry, eighteen months is a long time. Say you’re a retailer stuck in a legacy structure. You don’t really have eighteen months to figure out your recommendations for example.
Conclusion
I think the biggest takeaway is that we achieve more in three and a half days, having this methodology and this structure in place, than some of these companies that we work with have done in a year.
To end this session, we found this quote from Charles Eames.
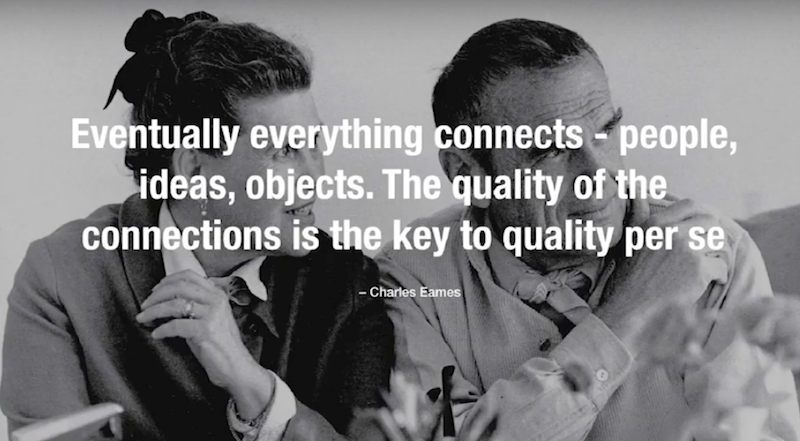
I think that everything mentioned connects people, ideas, objects. The quality of the connections is key. It’s a very nice way to frame what we try to do, teach and experience within Innovation Labs.