Breaking out of Data Jail: How Arcurve Better Serves Its Clients with Neo4j Graph Data Science
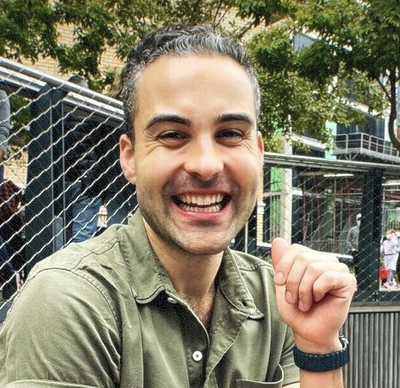
Senior Product Marketing Manager
3 min read
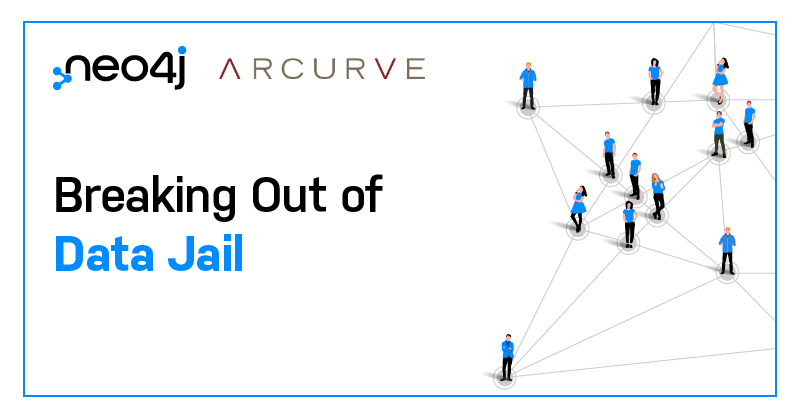
One of North America’s leading technology services companies, Arcurve, works tirelessly to help clients solve their most challenging problems across industries like energy, technology, finance, and government. Arcurve prioritizes cutting-edge data science and machine learning advancements – including Neo4j Graph Data Science – to support their clients, from startups to Fortune 500 companies.
With a focus on data relationships, two of Arcurve’s bright minds – Mike Morley, Director of AI and ML Technology, and Pete Tunkis, Senior Data Scientist with the Arcurve Advanced Analytics team – use the power of Graph Data Science to combine structured and unstructured data.
They find that it offers a more natural, connected way to answer their most critical data science questions like: what’s important? (prioritization), what’s unusual? (anomalies), and what’s next? (predictions). Plus, with a native Python client and access to more than 65 pretuned graph algorithms, it was easy for them to get started quickly using the data science skills they already had.
The Knowledge Asset
The Challenge
Across industries, many of Arcurve’s clients face challenges accessing the correct documentation and information in the right context. This is particularly difficult for teams trying to analyze disparate and complex datasets in legal and administrative contexts.
As Mike Morley explains, “Unstructured data – like files stored as legal documents, contracts, environmental reports, or work tickets – are trapped in ‘data jail’ because it’s hard to analyze using traditional data and analytics methods. Potentially useful information is completely left out of analysis because it’s unstructured.”
The Graph Data Science Solution
Using Neo4j Graph Data Science, Arcurve data scientists Mike and Pete are able to break complex files into data to find patterns in the resulting structure. Consequently, the datasets derived from structured data then create a comprehensive “knowledge asset.”
Knowledge assets have several applications. For example, many companies file similar types of legal documents with courts. Using a knowledge asset to identify similarities between the language and arguments used in comparable court cases can help speed up the legal process, lead to more successful filings, and reduce legal costs.
“We helped organizations reduce the time of triaging legal documents by approximately 70 percent by identifying which sections of the documents were most relevant to legal or regulatory use cases,” Mike elaborates.
In another example, Mike and Pete created a knowledge graph of a mining system that blended structured data about the system’s performance with unstructured data from maintenance reports and work tickets. By unifying these two types of data into a single analysis, they were able to improve the accuracy of the financial impact measure of unplanned downtime by 40 percent.
Mitigation, Mining, and Safety
The Challenge
Arcurve is headquartered in Calgary, Alberta, and works with many clients within the energy and mining industries. When mining or energy companies cease production, mines – often referred to as abandoned or orphan wells – get returned to the local government.
“Because remediation and clean-up are very expensive, local governments and their partners need to prioritize abandoned well sites for remediation based on public safety and environmental risk,” Pete clarifies.
The Graph Data Science Solution
With Graph Data Science, Pete and Mike connected disparate datasets to find areas of overlap to build out a holistic and comprehensive replica of a mine.
“Using Neo4j Graph Data Science, we were able to combine analytical and semi-structured geological data shared by the Alberta Energy Regulator and other industry partners that contained partial keys to build a complete picture of a well site. These well sites were then used to characterize the sites from a geological, incident, and production standpoint,” Pete shared.
Using the digital replica, Mike and Pete identified the top 15 wells for remediation for the local governments to prioritize citizen safety and minimize environmental degradation. This means cleaner water and fewer harmful incidents, an invaluable benefit to the people and communities of Alberta, Canada.
The applications – and benefits – of Graph Data Science are limitless.
Ready to learn more about how Graph Data Science can serve and benefit your own business challenges? Download our Use Case Selection Guide for Free.