Text2Cypher: The Impact of Difficult Example Selection
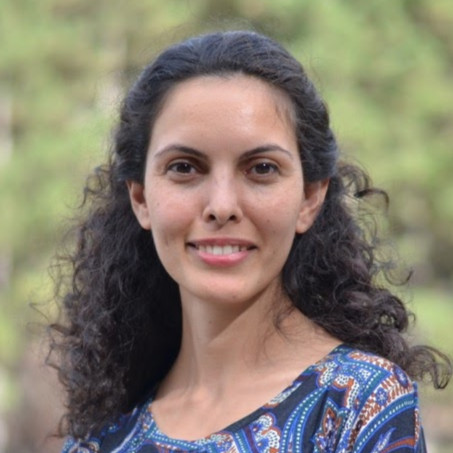
Machine Learning Engineer, Neo4j
6 min read
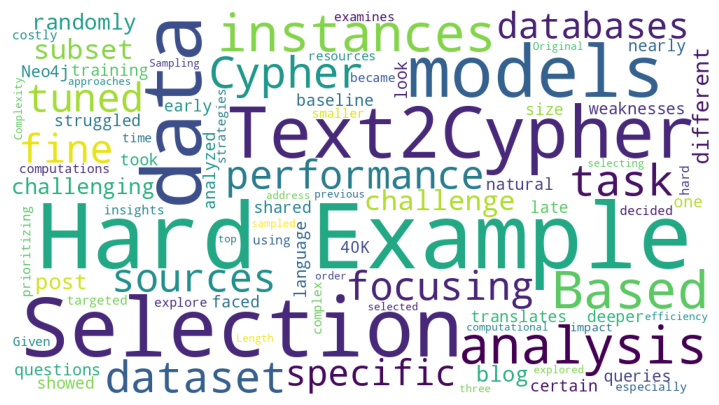
The Text2Cypher task translates natural language questions into Cypher queries. In late 2024, we shared our Neo4j Text2Cypher (2024) dataset, along with our analysis of the performance of both baseline and fine-tuned models.
In early 2025, we took a deeper look at fine-tuned models, focusing on their weaknesses. Our analysis showed that these models struggled more with certain data sources and specific databases. During this analysis, one challenge we faced was the size of the training dataset — with nearly 40K entries, computations became costly in both time and resources.
Given these insights, we decided to explore using a smaller, more targeted subset of data, prioritizing more complex or challenging (hard) instances. This blog post examines how different subset selection strategies — especially focusing on more difficult, hard examples — impact both performance and computational efficiency in the Text2Cypher task.
Hard-Example Selection Techniques
In order to address these challenges, we explored three different approaches for selecting hard examples.
Complexity-Based Hard-Example Selection
Based on the analysis from a previous blog post, we selected instances from the top-k most challenging databases and data sources. The chosen databases are [‘neo4jlabs_demo_db_recommendations’, ‘neo4jlabs_demo_db_companies’, ‘neo4jlabs_demo_db_neoflix’]
, and the selected data sources are [‘neo4jLabs_functional_cypher’, ‘neo4jLabs_synthetic_gemini’, ‘neo4j_text2cypher2023_train’]
.

Focusing only on complex data led to an imbalanced dataset, with too many instances from a single data source group. To address this, we performed further sampling, limiting each group to a maximum of 4,000 instances, which is the average size of a data source group. This process resulted in a total of 16,173 instances, less than half of the original training data.

Length-Based Hard-Example Selection
This heuristic-based approach assumes that longer ground-truth Cypher queries are more challenging for a language model to generate due to their increased complexity. Longer queries often involve multiple clauses, making them harder to replicate accurately. As a result, this approach selects instances with the longest “cypher” field. To ensure fairness with other hard-instance selection methods, we kept the sampled dataset size at 16,173 instances.

Cypher-Specific Hard-Example Selection
This heuristic-based approach assumes that ground-truth queries containing more Cypher-specific terms (such as MATCH
, WHERE
, RETURN
) are more challenging for the model. Unlike the length-based approach, which focused on the length of the query, this method focuses on the presence of Cypher terms (i.e., amount of them and selecting queries likely to be more complex, which is those containing multiple clauses). To maintain consistency with other hard-instance selection methods, we kept the sampled dataset size at 16,173 instances.

Others — Combined Approaches and Baselines
Furthermore, we combined these ideas:
- Complexity-based and length-based selection — After selecting hard examples using the complexity-based approach, we further sorted them in descending order based on Cypher query length, following the length-based approach.
- Complexity-based and Cypher-specific selection — After selecting hard examples using the complexity-based approach, we then ranked them by the number of Cypher-specific terms in descending order, aligning with the Cypher-specific approach.
In addition, we included two types of baselines for the analysis:
- Original data — This baseline uses the training data with no modifications.
- Sampling randomly — We randomly sampled from the original data while ensuring a balanced selection for fairness with the complexity-based approach. First, we sampled each group (based on the data-source field) with a size of 2,755, representing the 75th percentile of data source group sizes. Then we refined the sample to 16,173 instances to maintain consistency with the previously discussed hard-instance selection methods.

Experimental Setup and Results
For our experiments, we used the Neo4j Text2Cypher (2024) dataset, specifically the test set. As detailed in previous blog posts on Neo4j’s Text2Cypher efforts (including results from baseline and fine-tuned models), we used multiple evaluation metrics to assess performance. Following the same approach, we conducted two types of evaluations: Translation (Lexical) Evaluation, which compares generated Cypher queries with ground-truth queries textually; and Execution-Based Evaluation, which executes generated and ground-truth Cypher queries on the target database and compares outputs. Note that Execution-Based Evaluation only works if there is an active target database, so it only assesses a subset of the data. In this post, we report the Google BLEU score and the Exact-Match score as the key metrics.
We fine-tuned the unsloth/Meta-Llama-3.1–8B-Instruct-bnb-4bit foundational model using the sampled datasets to analyze the impact of hard-example selection. We examined the results in three key areas.
Impact of Training Data Reduction
We compared the original dataset with sampled subsets in terms of performance and training time.

Comparing the sampled and original datasets shows that reducing dataset size cuts training time by more than half, as fewer training steps are required. Analyzing the fine-tuning process reveals that both datasets follow a similar loss function trend. While training with randomly sampled data results in a loss value of 0.0569, using the original dataset achieves a lower loss of 0.0387, indicating better convergence.
The evaluation results follow a similar pattern. Since translation-based evaluation measures token prediction accuracy, its results align closely with the loss function. The original dataset achieves a Google BLEU score of 0.75 and an Exact-Match score of 0.36, whereas the random sampling approach scores lower at 0.69 and 0.20, respectively. Execution-based evaluation follows the same trend but with a smaller performance drop. The original dataset achieves 0.25 (Google BLEU) and 0.27 (Exact-Match), while the sampled dataset scores 0.21 and 0.25, respectively. In short, using a subset of data significantly reduces training time and cost — by more than half — but at the expense of performance.
Impact of Using More Challenging (Hard) Instances
We compared random sampling and hard-example selection approaches.

When fine-tuning with datasets prepared using random sampling or hard-example selection approaches, training times remain similar, as the dataset sizes were kept equal. All methods achieve comparable loss values, ranging between 0.05 and 0.06. However, a closer look reveals a ranking from highest (worst) to lowest (best) loss: length-based → random-sampled → Cypher-specific → complexity-based. The same trend is observed in translation-based evaluation, where the complexity-based approach performs best, achieving 0.71 Google BLEU and 0.25 Exact-Match, bringing it closer to the performance of the original dataset. Interestingly, execution-based evaluation follows a different pattern. Here, the Cypher-specific approach yields the best results, with 0.23 Google BLEU and 0.26 Exact-Match scores.
Impact of Combining Hard-Example Selection Approaches
We compared individual methods with their combined versions.

Combining the complexity-based approach with either length-based or Cypher-specific approaches did not lead to significantly different loss values. As expected, translation-based evaluation, which measures token prediction accuracy, followed a similar trend. All approaches performed similarly, with Google BLEU and Exact-Match scores around 0.71 and 0.25, respectively. However, execution-based evaluation showed some variation. The complexity-based and length-based approach achieved the highest Google BLEU score (0.24), while the complexity-based and Cypher-specific approach obtained the best Exact-Match score (0.25).
These findings suggest that while combining approaches doesn’t drastically impact performance, certain combinations may offer slight advantages depending on the evaluation method.
Summary and Next Steps
Although the highest Google BLEU and Exact-Match scores — both for translation- and execution-based evaluation — remain below the performance achieved with the full dataset, hard-example selection outperforms random sampling. Moreover, hard-example selection reduces resource usage (both time and cost) by more than half — compared to the original — improving efficiency without a drastic drop in performance.

One key observation is the convergence of the fine-tuned models. Even if we use a relatively large dataset with around 16,000 instances, it looks like they can still use more data or better-tuned hyperparameters. We plan to explore increasing data diversity and fine-tuning hyperparameters to further improve performance.
Another important takeaway is the behavior of evaluation methods. Since translation-based evaluation measures token prediction accuracy, its results closely align with the loss function. However, execution-based evaluation doesn’t always follow the same pattern. In the future, we’ll analyze how different data subsets impact the model’s ability to generate accurate Cypher queries during execution-based evaluation.
Stay tuned as we continue to uncover insights for optimizing Cypher generation models!
Text2Cypher: The Impact of Hard Example Selection was originally published in Neo4j Developer Blog on Medium, where people are continuing the conversation by highlighting and responding to this story.