Why Modern Fraud Detection Needs Graph Database Technology
3 min read
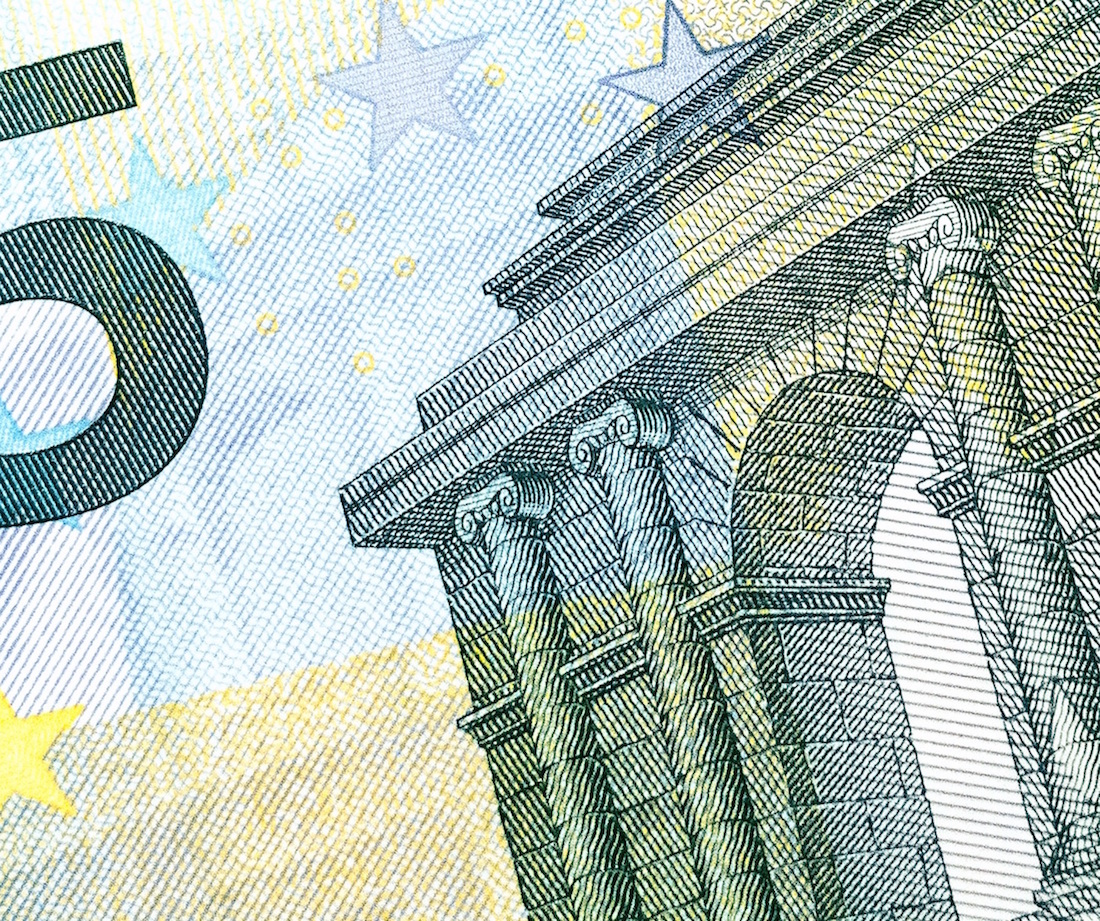
Banks and insurance companies lose billions of dollars every year to fraud.
Traditional methods of fraud detection play an important role in minimizing these losses. However increasingly sophisticated fraudsters have developed a variety of ways to elude discovery – both by working together and by leveraging various other means of constructing false identities.
Graph databases offer new methods of uncovering fraud rings and other sophisticated scams with a high-level of accuracy, and are capable of stopping advanced fraud scenarios in real-time.
In this series on fraud detection, we’re going to take a closer look at how graph databases help detect and mitigate three types of fraud:
This week, we’ll take a broader look at how graph databases detect fraud in multiple scenarios.
The Value of Graph Databases for Advanced Fraud Detection
While no fraud prevention measures can ever be perfect, you can achieve a significant opportunity for improvement by looking beyond individual data points, to the connections that link them. Oftentimes, fraudulent data connections go unnoticed until it is too late – something that is unfortunate, as these connections oftentimes hold the best clues to detecting a fraud scenario.
Understanding the connections between data – and deriving meaning from these links – doesn’t necessarily mean gathering new data. Significant insights can be drawn from your existing data, simply by reframing the problem and looking at it in a new way: as a graph.
Unlike most other ways of looking at data, graphs are designed to express relatedness. Graph databases can uncover patterns that are difficult to detect using traditional representations such as tables.
An increasing number of companies are using graph databases to solve a variety of connected data problems, including fraud detection.
While first-party bank fraud, insurance fraud and e-commerce fraud are all entirely different types of fraud, they all hold one very important thing in common: the deception relies upon layers of indirection that can be uncovered through connected data analysis.
In each of these examples, graph databases offer a significant opportunity to augment one’s existing methods of fraud detection, making evasion substantially more difficult. In the coming weeks, we’ll be taking a closer look at each of these fraud scenarios and how graph technology is being used to detect and deter fraudulent activity.
The Importance of Real-Time Fraud Detection & Connected Data Analysis
Whether it is bank fraud, insurance fraud, e-commerce fraud or another type of fraud, two points are very clear:
First is the importance of detecting fraud as quickly as possible so that criminals can be stopped before they have an opportunity to do too much damage. As business processes become faster and more automated, the time margins for detecting fraud are becoming narrower and narrower, increasing the need for real-time solutions.
Second is the value of connected data analysis. Sophisticated criminals have learned to attack systems where they are weak. Traditional technologies – while still suitable and necessary for certain types of prevention – are not designed to detect elaborate fraud rings. This is where graph databases add value.
Graph databases are the ideal enabler for efficient and manageable fraud detection solutions. From fraud rings and collusive groups, to educated criminals operating on their own, graph databases provide a unique ability to uncover a variety of important fraud patterns, in real time.
Collusions that were previously hidden become obvious when looking at them with a system designed to manage connected data, using real-time graph queries as a powerful tool for detecting a variety of highly-impactful fraud scenarios.
Learn how to harness the power of graph databases for your enterprise fraud detection efforts with this white paper, Fraud Detection: Discovering Connections with Graph Databases.
Catch up with the rest of the fraud detection series: