Fujitsu Laboratories of America Partners with Larus to Provide Enhanced Graph AI Technologies to Neo4j Customers
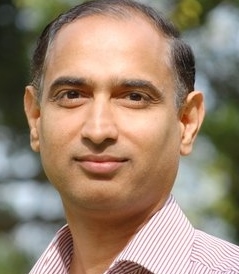
Marketing Director at Fujitsu Laboratories
3 min read
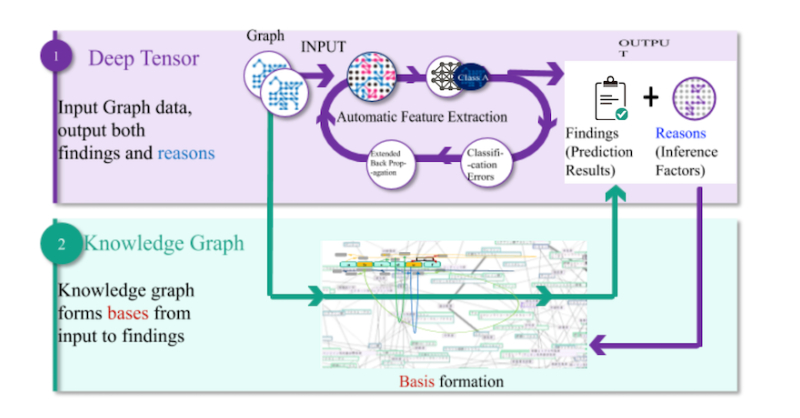
From social media data to financial transactions and web log data, graphs are ubiquitous in today’s interconnected world. Likewise, the size and scale of graph databases is increasing exponentially with modern applications.
To classify and understand relationships in graph databases – and drive insightful decisions for business advantage – enterprises are racing to adopt graph AI technologies to gain a competitive advantage.
Graph technology incorporates context and connections that make AI more broadly applicable – and that’s crucial for valuable AI applications.
In effect, making a decision requires having the right contextual, relevant information and ability to automate or streamline that process in some way. There are several areas where graphs provide context for AI:
- Context for decision support with knowledge graphs
- Context for efficiency of processing with graph accelerated ML
- Context for accuracy with connected feature extraction
- Context for transparency into the way AI makes decisions
Applications of graph AI technologies include understanding diseases and cures based on genetic mutations, identifying fraudulent activities from banking transactions and extracting insights from social media data.
An example is the area of network security, where breaches at corporations have resulted in billions of dollars of losses and erosion of company reputation. The prevention of these attacks and securing corporate networks from intruders is a business critical priority for CEOs and CIOs.
By storing the underlying network topology in a graph database and applying graph AI technologies, companies are able stop brute force or denial of service attacks even when the underlying network patterns, which can be represented as graphs, are changing dynamically.
Fujitsu Laboratories, the R&D arm of Fujitsu Ltd., developed a new AI technology that uses a novel tensor decomposition method called “Deep Tensor.” Deep Tensor leverages new tensor processing techniques and a deep neural network to automatically extract relevant features of graph data.
Fujitsu Laboratories has collaborated with customers across industry verticals to demonstrate that the Deep Tensor decomposition method results in high accuracy analytics for various types of graph data.
Additionally, Fujitsu Laboratories has integrated Deep Tensor technology with knowledge graph to provide additional explanation for the reasons and basis behind AI-generated findings.
Larus, a Neo4j top solution partner based in Italy with over 15 years of business critical project expertise, helps companies around the world design large-scale, data-driven systems based on the Neo4j’s Graph Platform.
Thanks to a graph-based approach to artificial intelligence and by empowering AI technology with related context, Larus helps organizations enhance the efficiency of their business operations by extracting actionable insights from connected data.
By partnering with Larus, Fujitsu Laboratories plans to build on Larus’s deep expertise in Neo4j customer requirements and application domain knowledge and complement it with advanced graph AI technologies.
This will enable both companies to further increase the footprint of Deep Tensor in the marketplace by making it available to additional customers of Neo4j database platform.
For the additional information on the joint offerings please contact: fla_deeptensor@us.fujitsu.com or deeptensor@larus-ba.it.
Read the white paper, Artificial Intelligence & Graph Technology: Enhancing AI with Context & Connections