Toward AI Standards: Context Makes AI More Reliable and Trustworthy
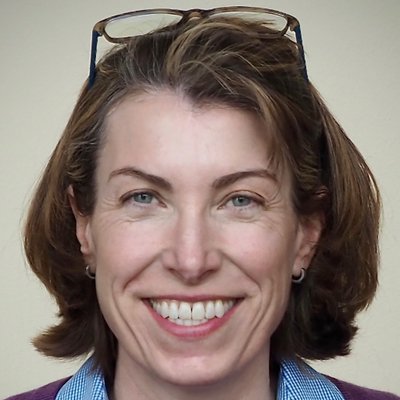
Graph Analytics & AI Program Director
6 min read
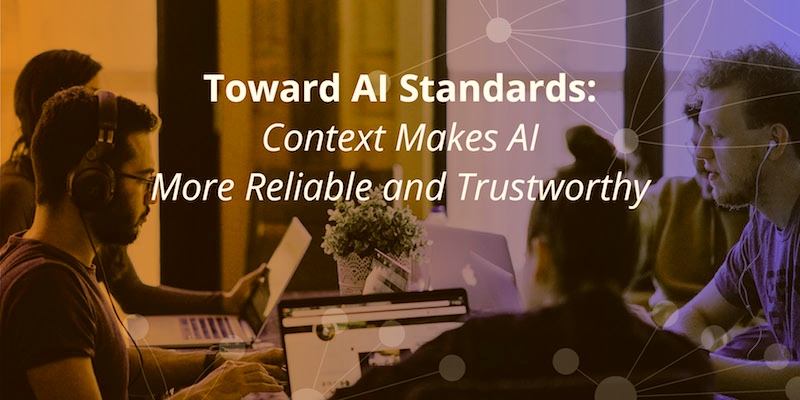
In part three of our series Toward AI Solutions, we explain how and why context makes AI more robust to manage difficult problems and help make the most informed decisions.
Trustworthiness is a critical topic for AI standards efforts and called out in the NIST draft engagement plan for U.S. LEADERSHIP IN AI as a key reason federal engagement in AI standards is needed.
“Increasing trust in AI technologies is a key element in accelerating their adoption for economic growth and future innovations that can benefit society. Today, the ability to understand and analyze the decisions of AI systems and measure their trustworthiness is limited. AI standards and related tools, along with AI risk management strategies, can help to address this limitation and spur innovation.”
This week, the final installment of this series, goes on to look at how adding contextual information enables AI-based systems to be more reliable, fair and trustworthy.
Reliability
In order for AI solutions to be reliable and fair, we need to know what data was used to train our models and why. Unfortunately, this isn’t as straightforward as we might think. If we consider a large cloud service provider or a company like Facebook with an enormous amount of data, it’s difficult to know what exact data was used to inform its algorithms. (Let alone which data may have changed!)
Graph technology adds the required context for this level of explainability.
For example, graph technology is often used for data lineage to meet data compliance regulations such as GDPR or the upcoming California Consumer Privacy Act (CCPA). A data lineage approach is also used on NASA’s knowledge graph to find past “lessons learned” that are applicable to new missions. When we store data as a graph, it’s easier to track how that data is changed, where data is used, and who used what data.
Understanding and monitoring data lineage also guards against the manipulation of input data.
For example, corporate fraud research has shown that when the significance of input data is common knowledge, people will game the system to avoid detection. Imagine a utility system or network infrastructure where we were confident in our monitoring software, but could no longer rely on the input data. The whole system would become immediately untrustworthy.
Finally, context-driven AI systems avoid excessive reliance on any one point of correlated data.
For example, organizations are beginning to apply AI to automate complex business dependencies in areas such as data centers, batch manufacturing, and process operations. With contextual coordination, they avoid the trap of noisy, non-causal information and use root-cause analysis to maximize future efficiency. Contextual information helps us identify the root cause of a problem as opposed to just treating a symptom.
Fairness
Understanding the context of our data also reveals the potential biases inherent in existing data as well as how we collect new data and train our models.
For instance, existing data may be biased by the fact that it was only collected for one gender, which is a known issue in medical studies as noted by Elysium Health in, “Do Clinical Trials Have a Sex Problem?” Or perhaps an AI’s human language interactions were trained on a narrow age or accent range. Graphs ensure situational/contextual fairness by bringing context to the forefront of AI solutions.
Higher arrest rates for some demographic populations become embedded in prosecution data. When historical input data is used for predictive policing, it causes a vicious cycle of increased arrests and policing.
The Royal Statistical Society published an Oakland, CA simulation analysis of a machine learning approach often used for predictive policing and found, “… that rather than correcting for the apparent biases in the police data, the model reinforces these biases.”
Fair-use of our personal data is an important part of AI-based systems. Contextual data can assist in privacy efforts because relationships are extremely predictive of behavior. This means we can learn from peripheral information and collect less information that is less personally identifiable.
In the book, Connected, James Fowler describes studies have shown that even with little or no information about an individual, we can predict a behavior such as smoking based on the behavior of friends, or even friends of friends.
Trust and Explainability
Training a machine learning model is mostly done on existing data, but not all situations can be accounted for ahead of time. This means we’ll never be completely sure of an AI reaction to a novel condition until it occurs.
Consequently, AI deployments need to dynamically integrate contextual information. For example, researchers have developed an application that predicts the legal meaning of “violation” based on past cases. However, legal concepts change over time and need to be applied to new situations. Because graph technologies capture and store relationships naturally, they help AI solutions adjust faster to unexpected outcomes and new situations.
To increase public trustworthiness of AI, predictions must be more easily interpretable by experts and explainable to the public. Without this, people will reject recommendations that are counterintuitive.
Graph technologies offer a more human-friendly way to evaluate connected data, similar to drawing circles to represent entities and lines to show how they are connected on a whiteboard.
There are also new ways to learn based on contextual information. For example, companies like eBay are mapping the potential pathways a person might take from one purchase to another in order to recommend another selection.
In the example of a music download, there’s a lot of context around a person and their selection: the artist, album, publishing decade, music genre, etc.
The paper, “Explainable Reasoning over Knowledge Graphs for Recommendation” details how to use graph technology and machine learning to predict the path (from song to artist, album, genre, or decade, etc.) that a person would likely take to get to their next song purchase.
We can use graphs to relay the complex data of people, songs, albums and their relationships into practical machine learning measures while retaining the various potential paths from one purchase to another. When we combine the likelihood of different paths with context, we better understand such AI-powered recommendations and decision making.
Oversight opportunities also benefit from increased AI explainability. When homeowners insurance increases for an individual, it’s frustrating; when rates increase for an entire demographic, it’s discrimination. Without efficient AI explainability, it will take regulators considerable more effort to determine the root cause of such discrimination.
We’re excited by recent work that appears to offer a leap forward for machine learning explainability. Particularly promising is the idea of graph native learning which involves computing machine learning tasks within a native graph structure itself to make use of its natural context.
Implementing artificial intelligence in a way that is underpinned by connected data as suggested above, results in AI solutions that are much more generally applicable.
However, perhaps even more significant will be the extreme transparency afforded by this approach: Graph native learning enables us to inputconnected data, learn while keeping data connected, and then output AI outcomes in the same graph format, which helps those accountable to accurately interpret results.
In addition, the intermediate states of learning become uniquely observable in that same connected format, which means experts track and validate an AI’s decision paths.
With the advancement of native graph learning, we can interpret and explain how an AI comes to a particular conclusion – which is a far cry from the black box approach used today.
Conclusion
Although any new standards endeavor is difficult and imperfect, NIST is making the necessary first steps in encouraging reliable, robust, and trustworthy systems that use AI technologies.
Furthermore, when considering such a broad and evolving area of technology, widely applicable standards and tools – such as integrating context – should be part of any AI foundation.
The federal government does have a role in creating guidelines that promote AI aligned to key American values of accountability, fairness and trust. Specifically, to advise that AI systems incorporate contextual data and process for better flexibility and situational appropriateness, explainability and transparency, protection against data manipulation and verifiability.
Finally, graph technologies are unarguably the state-of-the-art method for adding and leveraging context. These technologies are used worldwide across a diverse array of industries.
Please contact us if we can be of assistance in gathering or understanding how connected data influences artificial intelligence.
Read the white paper, Artificial Intelligence & Graph Technology: Enhancing AI with Context & Connections
Catch up with the rest of the Toward AI Standards series: