Customer Success Stories
1,700+
global organizations rely on Neo4j
84
of the Fortune 100 are Neo4j customers
58%
of the Fortune 500 power their business with Neo4j
Featured Stories
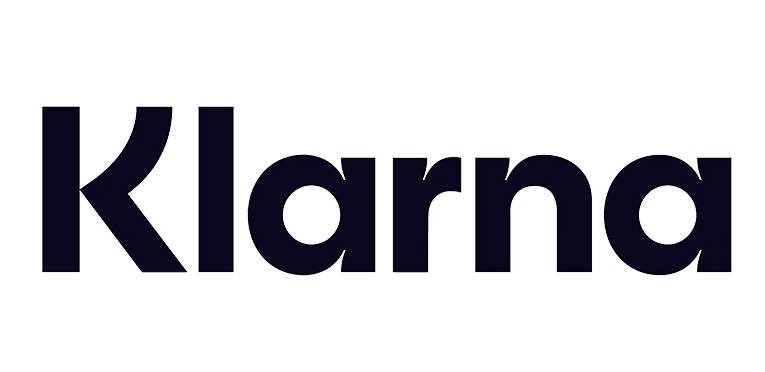
“At Klarna, we’re transforming the way we collaborate with our GenAI chatbot Kiki, powered by Neo4j’s knowledge graph”
Read Case StudyWestJet Takes Flight with Booking Experience Powered by Neo4j
Read Case Study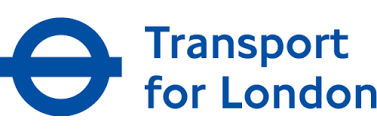
Transport For London Cuts Congestion by 10% with a Digital Twin Powered by Neo4j
Read Case Study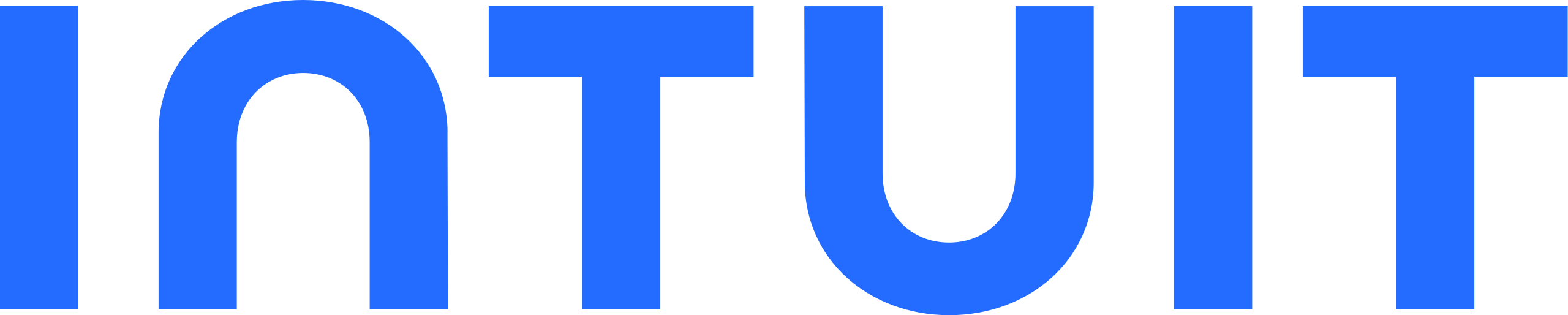
Intuit Safeguards the Data of 100 Million Customers with Neo4j
Read Case Study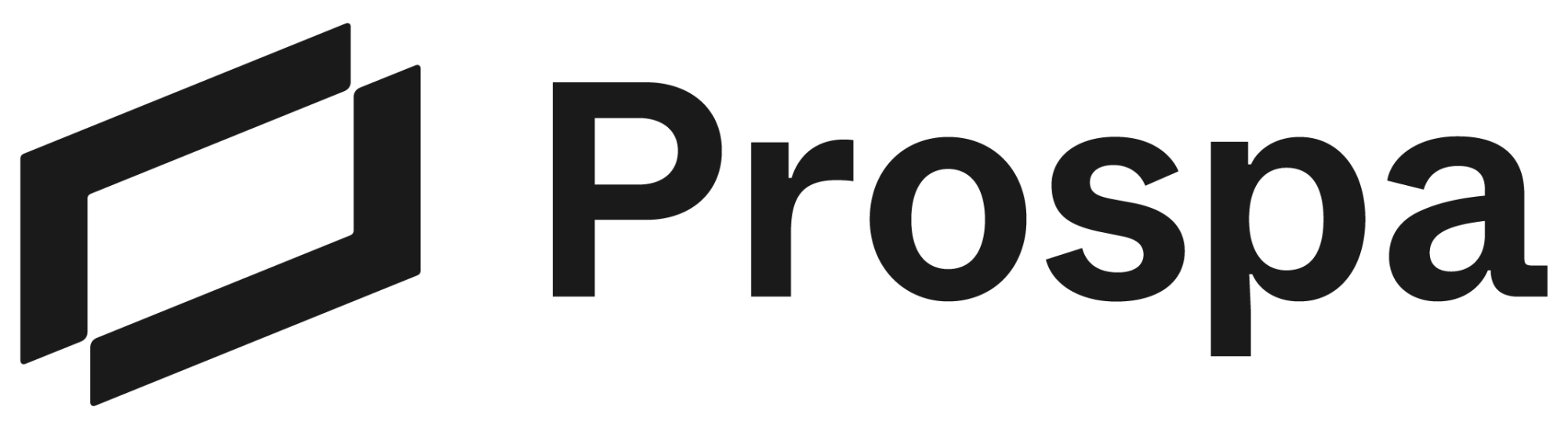
Prospa Propels Australia’s Small Businesses with Neo4j Graph Technology
Read Case Study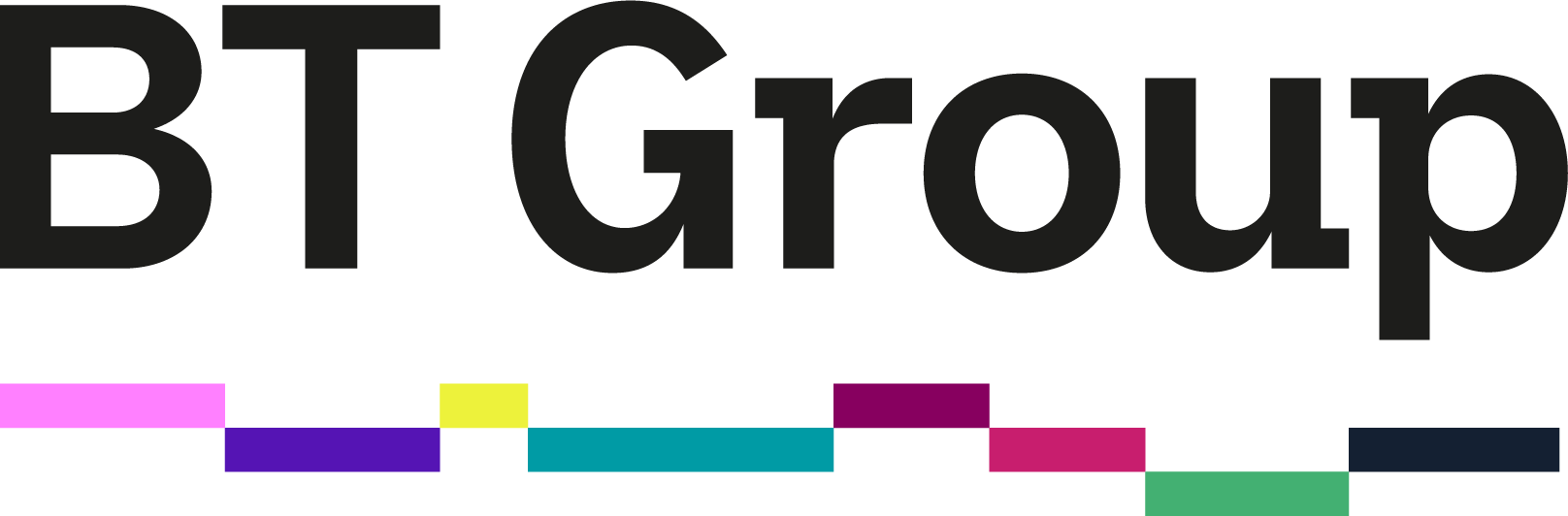
BT Group Keeps Customers Connected with Lightning-Fast Inventory Management
Read Case StudyEnabling Global Companies of All Sizes
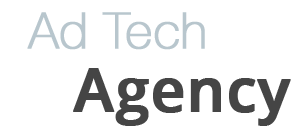
Identity Graph Analysis at Scale Provides Ad Tech Agency Customers with Greater ROI
Building the Largest Knowledge Graph of Life on Earth
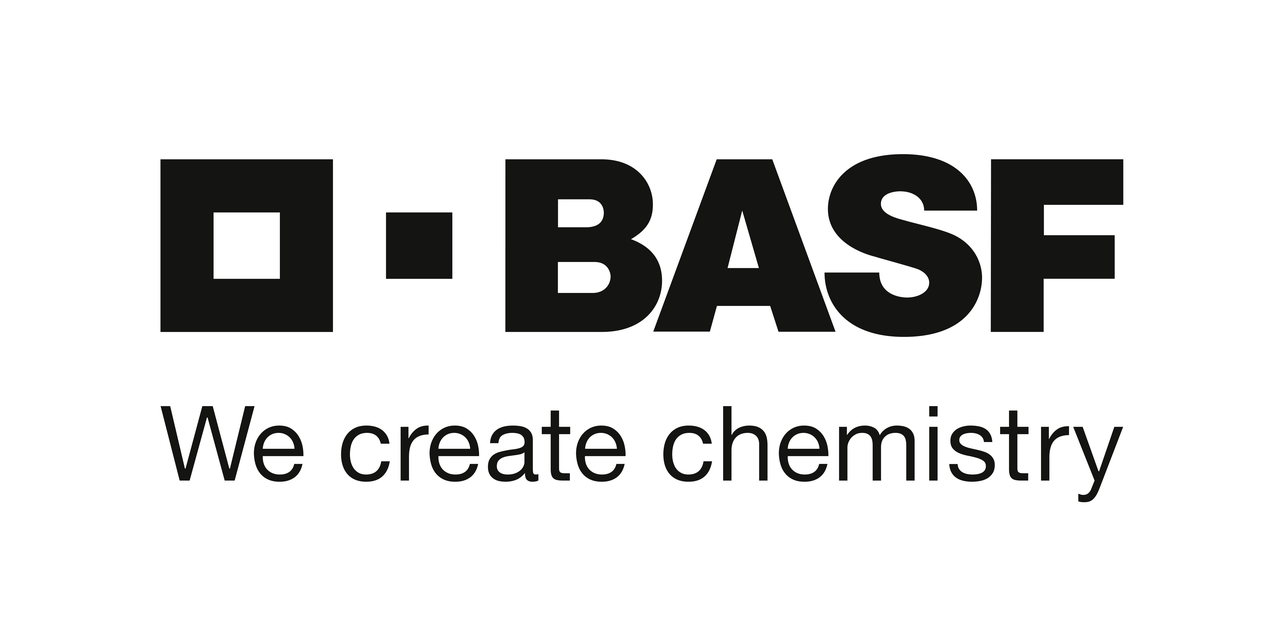
BASF SE Transforms Global Value Chain Analysis with Neo4j
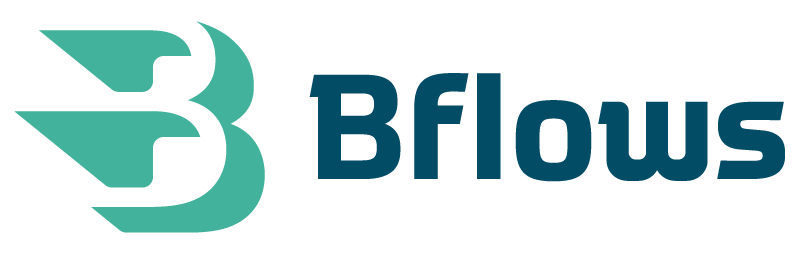
Driving Faster Cash Flow Management with Neo4j

BNP Paribas Personal Finance Reduces Fraud by 20% with Neo4j
Graph Data Science Streamlines Complex Medical Supply Chain Analysis
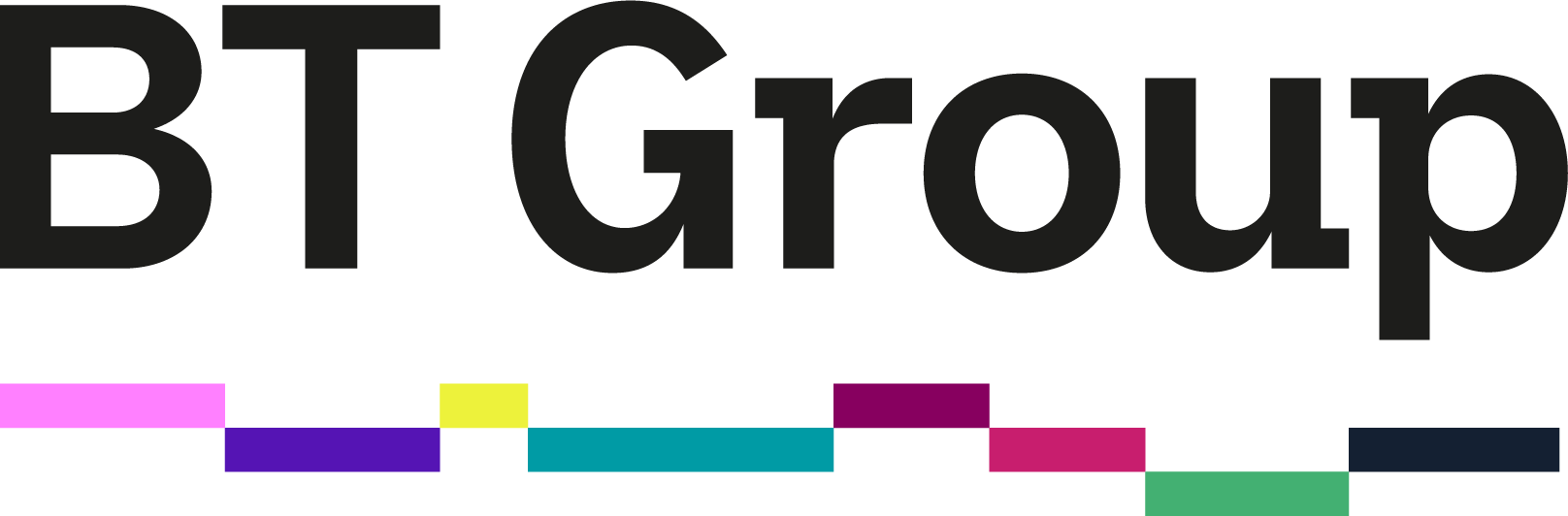
BT Group Keeps Customers Connected with Lightning-Fast Inventory Management
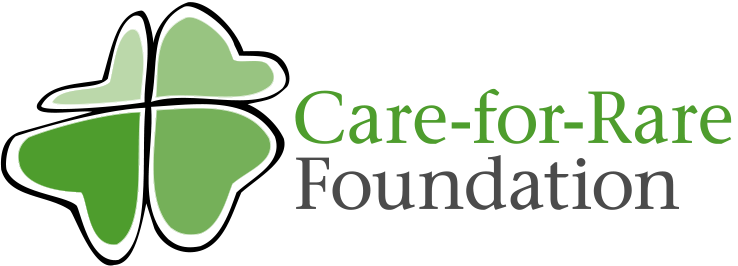
Care-for-Rare Identifies Rare Childhood Diseases with Neo4j
Graph Technology Fuels Rapid Development of IT Architecture Visibility Solution
Neo4j Provides Natural Language Processing at Scale, Making Equipment Repair More Efficient
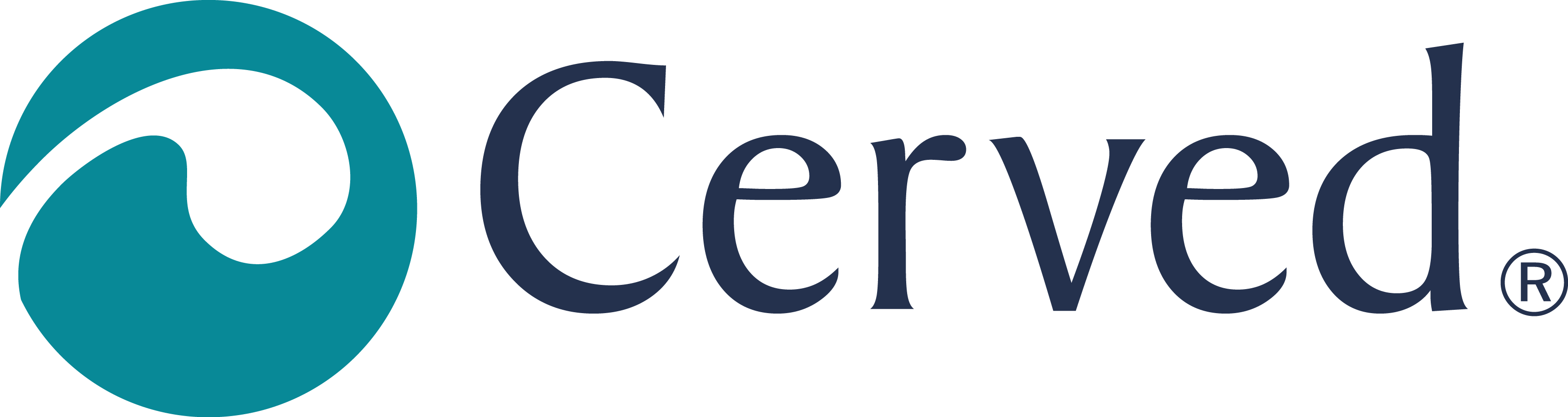
Companies and Their Economic Connections Are More Transparent Thanks to Big Data Intelligence
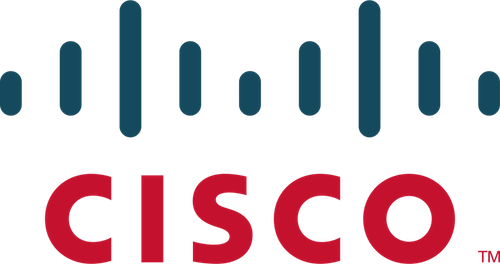
Real-Time Graph Analysis of Documents Saves Company Over 4 Million Employee Hours
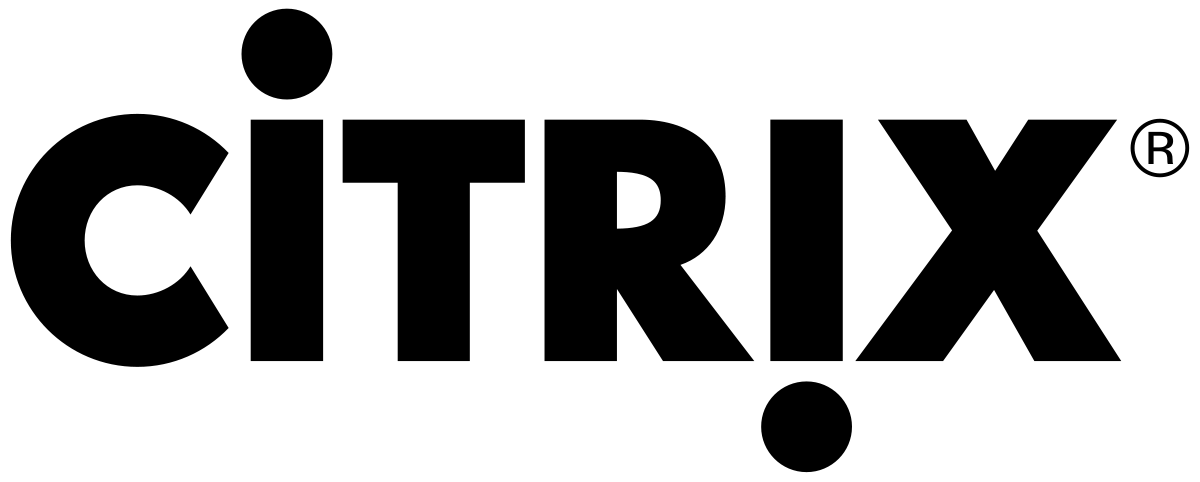
Neo4j Keeps Millions of Remote Workers on Citrix Safe, Productive, and Secure
COVID-19 Contact Tracing with Neo4j
Graph Technology Helps Xfinity Create Personalized, Smart Homes
Building a Digital Twin of the Largest Railroad in the Eastern U.S. with Neo4j
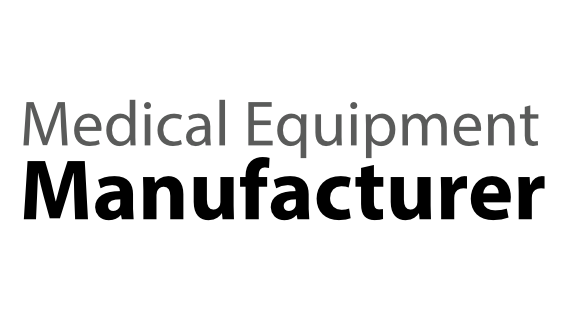
Detailed Visibility into Complex Workflows
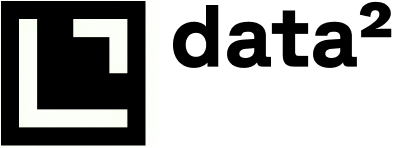
Data² Builds Leading GenAI Analytics Platform with Neo4j
Cloud-Based Graph Technology Drives Efficiencies
E-Health Company Creates a Knowledge Graph Solution to Help Patients with Chronic Pain
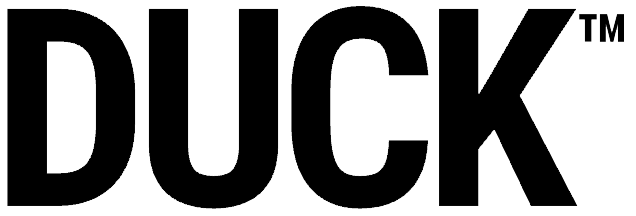
DUCK Transforms Customer Analysis with Neo4j and LLM-Powered Netnography Platform
Dun & Bradstreet Chooses Neo4j to Accelerate Clients’ Compliance with Complex Company Ownership Checks
DXC Technology Upskills its Talent Pool With Neo4j
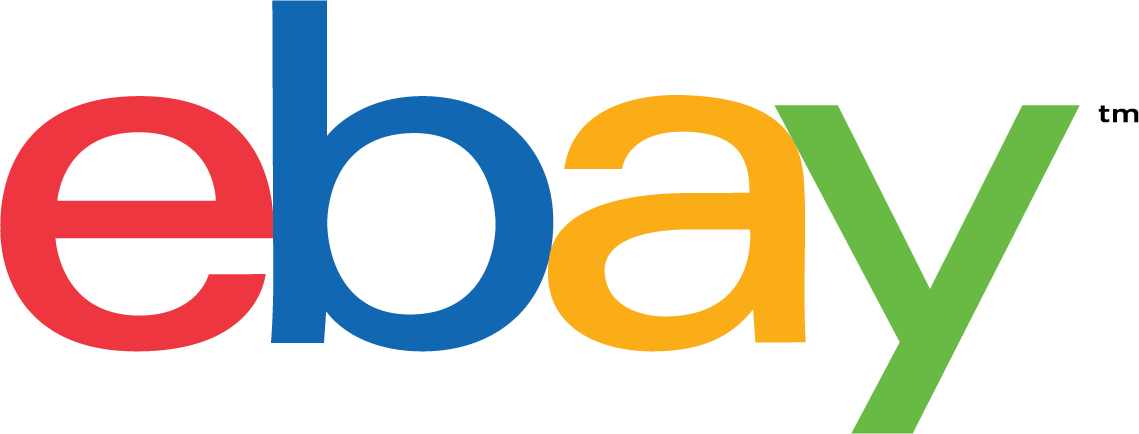