BNP Paribas Personal Finance Reduces Fraud by 20% with Neo4j
BNP Paribas Personal Finance, a subsidiary of banking giant BNP Paribas Group, specializes in retail financing through consumer credit and installment payment services where sniffing out fraudsters is a critical part of their mission.
800,000
applications processed per year
20%
reduction in fraud with Neo4j graph-powered fraud detection
2s
maximal query latency with Neo4j
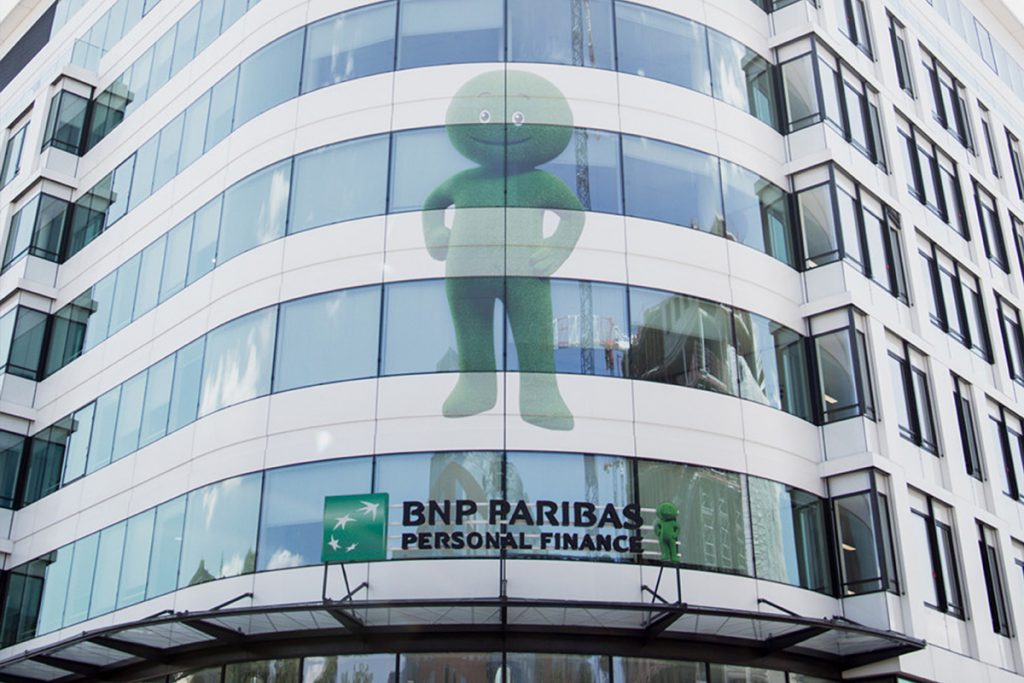
Installment payment services enable consumers to split payments over three or four future installments. Unfortunately, these types of payments tend to attract a swarm of fraudsters — individuals who deceitfully apply for credit, manipulating or concealing personal details to mask their true identities and intentions of never paying the balance.
“Fraudsters and fraud rings reuse information across many different credit applications,” explains Mehdi Barchouchi, Head of Innovation Data & Tools, Risk Division at BNP Paribas Personal Finance France. “They also change this information frequently on many different applications in order to circumvent current rules and blacklists.”
With over 800,000 applications and 85 retail merchants relying on secure payments and Transactions, robust anti-fraud tools are paramount for BNP Paribas Personal Finance’s extensive customer base. BNP Paribas Personal Finance chose Neo4j’s graph database to reinforce its fraud framework after an initial proof of concept (POC) to enable the development of an additional fraud detection system that could overcome specific information combination tactics used by savvy fraudsters.
“Our collaboration with Neo4j helped us develop a successful fraud detection model that met our expectations. It’s a win-win fruitful partnership.”
Close Collaboration with Neo4j Paves the Way for Success
A relational database could support blacklist checks and velocity rules to identify users and evaluate their behavior, but it faltered in uncovering vital relationships within vast volumes of connected data in real time.
“To create an effective fraud detection system, we needed to establish links between consumers’ credit applications and diverse data, even if these connections didn’t share common information,” says Barchouchi. “Real-time scoring decisions — approving or referring applications — were crucial for immediate customer responses.”
Traditional relational databases simply couldn’t meet these demands. Well-suited for traditional SQL queries, they lacked the capability for traversing deeper relationships in the data. Multiple joins would be needed to detect these relationships, resulting in performance bottlenecks and cost-prohibitive processing and networking overhead.
“The overall complexity and query times would rise significantly as the total size of the database grew,” explains Barchouchi. “Especially with exponentially more connection points and the depths of the relationships we needed to explore.”
Developing a Successful Fraud Detection Model Through Real-Time Scoring Decisions
BNP Paribas Personal Finance selected Neo4j and its Neo4j Enterprise graph database system. Both parties collaborated closely on an initial proof of concept (POC) to demonstrate the viability of the new fraud detection system.
“When we first started the pilot, we didn’t know exactly how we would transition from a traditional relational database to a graph database,” recalls Barchouchi. “The Neo4j team played a pivotal role, deeply involved in crafting our inaugural data model and developing new queries to calculate optimal fraud indicators for future deployment across our entire database.”
BNP Paribas Personal Finance Reduces Fraud by 20% with Graph-Powered Fraud Detection
The result? A remarkably potent detection model that could defend against nearly all fraudster networks. With Neo4j, BNP Paribas Personal Finance strengthened its automated fraud detection process with a maximal latency of 2s.
Now, new data from credit applications integrates directly into the graph database and quickly compares all historical requests in milliseconds. As clusters and relationships emerge, the system identifies potential fraud signals using similarity links in Neo4j.
BNP Paribas Personal Finance has designed a fraud detection scoring model to assess all applications and refer the riskiest ones: “We created a machine learning model which takes as inputs various embeddings derived from Neo4j graph database,” says Julie Cavarroc, data scientist in Scoring Center, Central Risk in BNPP PF.
“With Neo4j, we have a much better view of each consumer’s application,” explains Julie. “The expansive data context enables us to discern intricate patterns, even uncovering links to known fraudsters. All of this gives us a powerful advantage in fraud detection and prevention.”
This approach rejected just a very small part of the total applications yet yielded a substantial 20% reduction in total fraud. This victory underscores BNP Paribas Personal Finance’s ability to thwart a high degree of fraud — that may have gone undetected in the past — without filtering out valid applications to maintain the highest loan volumes possible.
The Future with Neo4j
In the ongoing journey, BNP Paribas Personal Finance remains dedicated to refining and improving both its graph data model and its fraud detection score to keep up with fraudulent patterns evolution.
Julie Cavarroc, Mehdi Barchouchi, and the entire team are confident in their partnership with Neo4j. “Our past database could not have delivered these outcomes,” he says. “Our entire experience with Neo4j helped us develop components of a successful fraud detection system that met our expectations.”
Get in Touch
Curious about what insights you could unlock for your business with graph-powered solutions? Let’s talk — reach out, and we’ll get in touch.