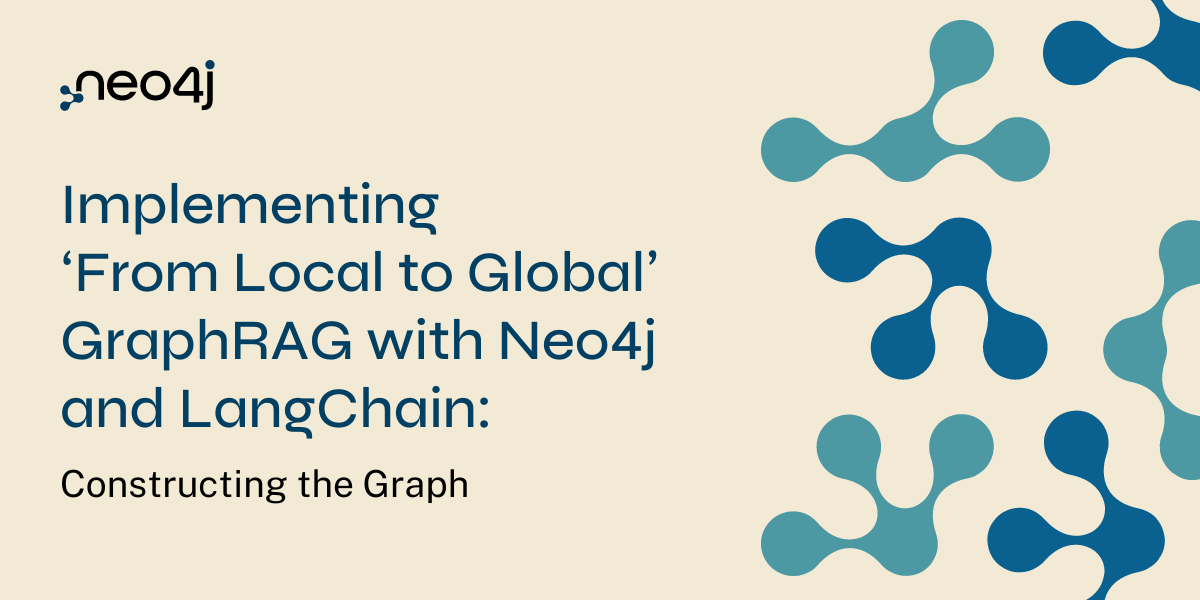
Implementing ‘From Local to Global’ GraphRAG with Neo4j and LangChain: Constructing the Graph
Jul 09 22 mins read
Learn how to combine text extraction, network analysis, and LLM prompting and summarization for improved RAG accuracy. Read more →
Learn how to combine text extraction, network analysis, and LLM prompting and summarization for improved RAG accuracy. Read more →
Learn how to build a GraphRAG application almost entirely in Cypher, using knowledge graphs and vector search in Neo4j. Read more →
Extract and use knowledge graphs in your GenAI applications with the LLM Knowledge Graph Builder in just five minutes. Read more →
The Neo4j GraphRAG Ecosystem Tools make it easy to develop GenAI applications grounded with knowledge graphs. Read more →
Discover how Neo4j and chatbots transform ASVS accessibility, enhancing application security with graphs and AI-driven insights. Read more →
Photo by Growtika on UnsplashThere are so many options when it comes to languages, frameworks, and tools for building generative AI (GenAI) applications. When you are just getting started, these decisions and figuring out how to integrate everything can be overwhelming.My… Read more →
Learn how we build a context-rich chatbot to answer Mahabharata questions using knowledge graphs and retrieval-augmented generation (RAG). Read more →
Learn how graph and vector search systems can work together to improve retrieval-augmented generation (RAG) systems. Read more →
In the 27 episodes of our Going Meta livestream series, Jesús Barrasa and I explored the many aspects of semantics, ontologies, and knowledge graphs. Read more →
Learn how to extract topics from documents with graph data science and use them as the basis for semantic search for better RAG applications. Read more →
Optimizing vector retrieval with advanced graph-based metadata filtering techniques using LangChain and Neo4j. Read more →
A guide to building LLM applications with the Neo4j GenAI Stack on LangChain, from initializing the database to building RAG strategies. Read more →
Learn how to use Neo4j and Senzing to build entity resolved knowledge graphs that remove duplicate data and improve analytics accuracy. Read more →
Learn how to use LangChain and Neo4j vector index to build a simple RAG application that can effectively answer questions. Read more →
Learn when to use graph data models, like parent-child, question-based, and topic-summary, for RAG applications powered by knowledge graphs. Read more →
A practical guide to constructing and retrieving information from knowledge graphs in RAG applications with Neo4j and LangChain. Read more →
Learn how to implement a knowledge graph-based RAG application with LangChain to support your DevOps team. Read more →
In this blog, you will learn how to use the neo4j-advanced-rag template in LangServe Playground to implement advanced RAG strategies. Read more →
Learn how to write graph retrieval queries that supplement or ground the LLM’s answer for your RAG application, using Python and Langchain. Read more →
Learn how to use PDF documents to build a graph and LLM-powered retrieval augmented generation application. Read more →
Exploring the Shortcomings of Text Embedding Retrieval for LLM GenerationLoch Awe in Scotland, photo by author.AbstractExternal knowledge is the key to resolving the problems of LLMs such as hallucination and outdated knowledge, which can make LLMs generate more accurate and reliable… Read more →