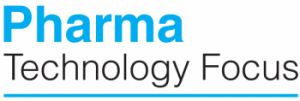
German centre for diabetes research changes its approach to handling data with Neo4j
The emergence of big data and other advances in technological innovation has provided medical researchers, among others, with the opportunity to attain valuable, previously unobtainable insight with the potential to improve all of our lives. The problem: real-world data comes in multiple, highly unstructured formats.
Why is that a problem? To really start exploiting the power of things like big data, researchers must go well beyond what they’re doing now – simplistic managing, analysis and storage of data. This means taking a cold and objective look at the tools they’ve historically used in research, especially spreadsheets and relational database technology.
That’s because traditional methods can’t cope with the volume, as well as the inconsistent nature, of the kind of data we want to study in-depth now. By its nature, medical data is heterogeneous: it can run from cell-level to detailed data to macro-scale disease network tracking across different diseases areas, with scientists often wanting to link both ends of that spectrum. Realistically, the breakthroughs we want to find are likely to come from working with many different data sets, as that is where the interesting results can lie, but it is a real challenge to model this.
DZD’s research network accumulates a huge amount of data that is in turn distributed across various locations.
As a result, Jarasch has built a new internal tool, DZDconnect, built in Neo4j that sits as a layer over the centre’s various relational databases, finally joining up different DZD systems and data silos. When fully implemented the expectation at the centre is that, using DZDconnect, its teams can access metadata from clinical studies and exploit the visualisation and the easy querying it has made possible.
Graph technology’s innate ability to discover relationships between data points allows us to understand them.
Graph technology’s innate ability to discover relationships between data points allows us to understand them, which could have an enormous role to play in medicine and healthcare in the future. And there’s also the promise that making these data connections is the beginning of a journey that could lead to real breakthroughs that older ways of working with data just can’t offer. Further input from developers to build graph-based data structures for research will enable ever more highly trained specialists to have access to data in a form they can work with much earlier in their research.
Read the full article →
Keywords: Diabetes Research German Center for Diabetes Research neo4j