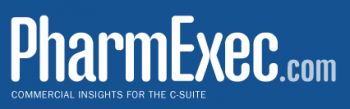
Emil Eifrem examines how life science researchers at Munich’s German Centre for Diabetes Research are uncovering insights with a new way of working with complex data
Diabetes is one of the most widespread diseases worldwide. Increasingly both type 1 and 2 forms of the condition in our ageing population will present major healthcare challenges in the coming years, with type 2 diabetes in children having risen 40% in three years amid Britain’s obesity epidemic
Clearly, investigating its causes and, through new scientific findings, developing effective prevention and treatment measures to halt the emergence or progression of diabetes is a priority for policymakers, citizens and the research community in all advanced economies.
Many researchers wonder if graph databases (technology that powered the Paradise Papers investigation as well as other examples of cracking big data problems) could provide the pharma industry with the opportunity for valuable, previously unobtainable insight that has the potential to improve our lives. That’s because, not only is graph technology ideally suited to depicting hidden relationships and discovering “known” and “unknown” unknowns at big data scale, it is also able to handle dynamic and constantly evolving data – something that is vital with scientific or bioinformatics analysis research.
That’s useful because real world data comes in different and highly unstructured formats. This means that big data life science research must go beyond simplistic managing, analysis and storage of data that fits neatly into a specific discipline and find new ways to achieve its objectives. This realisation has resulted in re-visiting the tools historically utilized for the purpose, including SQL and relational database technology. Unfortunately, traditional relational database methods can’t cope with the volume, as well as the inconsistent data, we need to use for impactful large-scale diabetes research of the kind we really need to start doing; medical data by its nature is very heterogeneous, running from cell-level to detailed data to macro-scale disease network tracking – all in the same research.
Further work to build graph-based data structures for research will enable ever more highly trained specialists to have access to data in a form they can work with earlier in their research. And ultimately, having the power to dive deeper and make the unknowns known in order to uncover the potentials in real-world data is a compelling tool in life science research.
Keywords: Diabetes Research German Center for Diabetes Research neo4j