Combating Money Laundering: Graph Tech Fights Serious Crimes
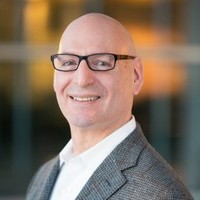
Enterprise Account Manager
1 min read
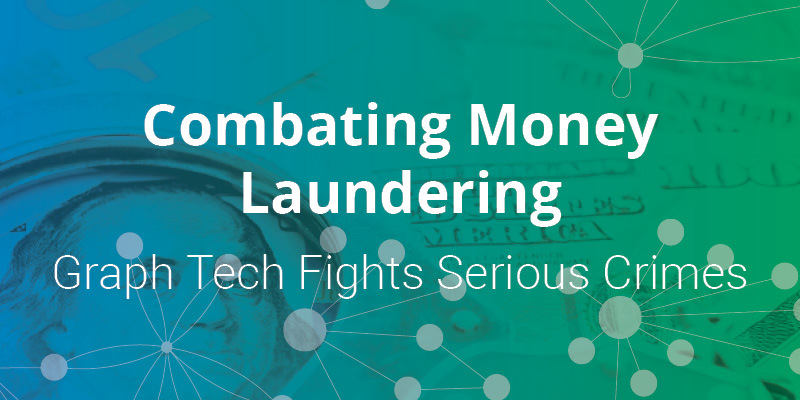
Money laundering is among the hardest activities to detect in the world of financial crime. Funds move in plain sight through standard financial instruments, transactions, intermediaries, legal entities and institutions – avoiding detection by banks and law enforcement. The costs in regulatory fines and damaged reputation for financial institutions are all too real. Neo4j provides an advanced, extensible foundation for fighting money laundering, reducing compliance costs and protecting brand value.
In this first blog in our six-part series on using graph technology to fight money laundering, we explain how money laundering occurs and the high price of failing to detect it.
Graph Technology Fights Serious Crimes
What Is Money Laundering?
Money laundering conceals the origins of illegally obtained money. Insider trading, drug trafficking, kickbacks and extortion are examples of crimes that require laundering large sums of money by principals through their agents. Agents might include individuals, corporations, financial institutions and law firms.
For example, at the height of its operations, the Medellín Cartel brought in more than US$70 million per day – more than $25 billion annually.
How Money Laundering Occurs
The money laundering process begins when illegal sums are placed in a bank, which in turn triggers a complex sequence of banking transfers or commercial transactions (layering) that return the money to the launderer in an obscure and indirect way (integration).
Money laundering is hard to detect because of the sophisticated layering techniques used to mask parties and layered transactions. Investigators often start with one or more bread crumbs and then follow the money trail through a series of structured transactions involving small deposits, cash and equivalents. The transactions move the funds through layers of agents, companies and financial institutions, making their owners difficult to identify.
The Panama Papers are 11.5 million leaked documents that detail financials and private information for more than 200,000 wealthy individuals, public officials and offshore agencies. The International Consortium of Investigative Journalists (ICIJ) used Neo4j to
discover payment chains and shell corporations used for fraud, tax evasion, evading international sanctions, and other illegal purposes. Based on the data and analysis, the result has been hundreds of ongoing investigations, $1.2 billion in fines and back taxes, and law enforcement has begun prosecuting.
The Role of Anti-Money Laundering Regulations
Governments have tightened laws and strengthened regulations around money laundering while investing more in enforcement – producing new revenue from non-compliance fines. Since 2015, annual AML penalty revenue has risen steadily each year. According to Encompass, in 2019 alone, regulators doled out more than $8 billion in fines. Negative consequences to banks also include damaged client relationships and reduced market competitiveness. One bank suffered a 30% decline in brand value due to negative media associated with money laundering.
Why Use Neo4j to Fight Money Laundering?
Fighting money laundering is an expensive undertaking. Large global banks process millions of transactions daily that involve tens of millions of parties. Different languages, disparate transaction formats, and unstructured data also make fighting money laundering more difficult.
Over the years, technology has automated the process, but data science techniques have not kept pace with the creative minds of money launderers. Natural language processing (NLP) has made it easier to identify potential criminal entities from unstructured media such as news feeds and documents. Artificial intelligence and machine learning (AI/ML) have helped to accelerate the evaluation of those entities. However, the high number of false positives
and negatives makes investigation expensive while real money laundering goes undetected.
These techniques fail to account for relationships in the underlying networks of parties and payment chains across transactions. The fundamental problem with these technologies is that they store data as rows and columns. As a result, their backend platforms are rigid, brittle and slow.
The Neo4j graph database fills this gap and enables what was once impossible. Neo4j unlocks the wealth of insights found by pattern matching on connected people, companies, financial institutions, places and times in a financial network. Neo4j treats relationships like first-class citizens, making it possible to match complex and changing patterns of connected money laundering data in real time and at scale.
Conclusion
In the following weeks, we will highlight various aspects of using graph technology to root out money laundering, diving into the money laundering detection process and exploring how an enterprise graph platform supports your anti-money laundering efforts.
Stop money laundering in its tracks.
Click below to get your copy of
How to Combat Money Laundering Using Graph Technology.