Top 10 Graph Database Use Cases: Fraud Detection
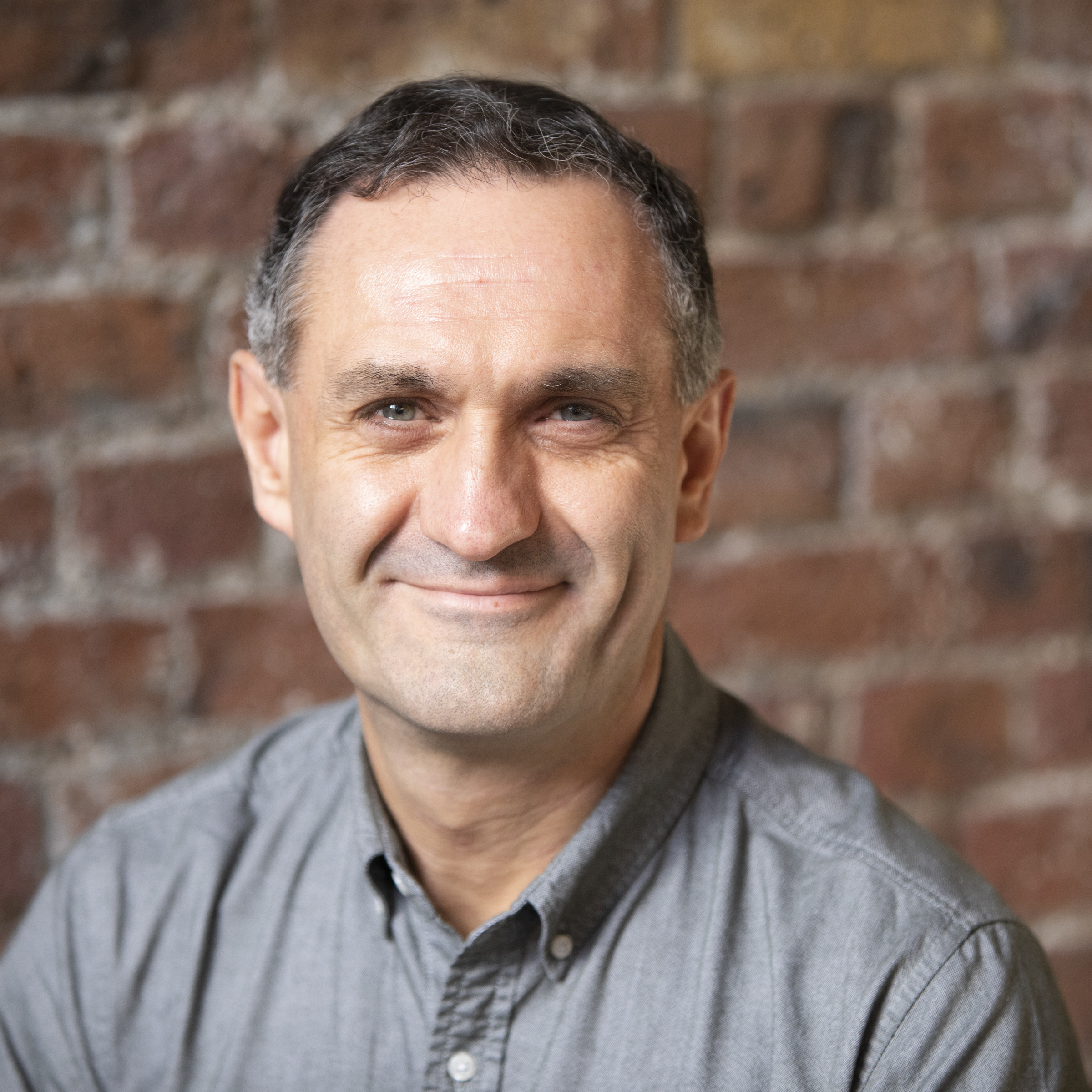
Chief Scientist, Neo4j
1 min read
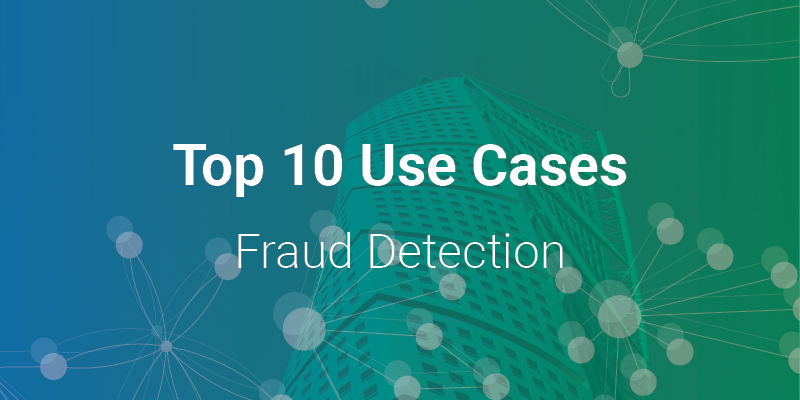
Graph technology is the future. Not only do graph databases effectively store relationships between data points, but they’re also flexible in adding new kinds of
relationships or adapting a data model to new business requirements.
But how do companies today use graph databases to solve tough problems? In this blog series, we’ll cover the top 10 use cases for graph technology and for each we include a real-world example. This blog series starts off with a difficult connected data problem for many businesses, especially in financial services: uncovering fraud.
Use Case #1: Fraud Detection
Banks and insurance companies lose billions of dollars every year to fraud. Traditional methods of fraud detection often fail to minimize these losses since they perform discrete analyses that are susceptible to false positives and negatives. Knowing this, increasingly sophisticated fraudsters develop a variety of ways to exploit the weaknesses of discrete analysis.
Graph technology offers new methods of uncovering fraud rings and other complex scams
with a high level of accuracy through advanced contextual link analysis. As a
result, fraud detection graph databases are capable of stopping advanced fraud scenarios in real time.
Unlike other ways of looking at data, graphs are designed to express relatedness. Graph technology uncovers patterns that are difficult to detect using traditional representations such as tables. An increasing number of companies use graph data technology to solve a variety of connected data problems, including fraud detection.
Example: Fortune 500 Financial Services Company
A Fortune 500 financial services company collects a huge amount of data that needs to be analyzed in real time before a transaction can be approved. While the majority of these requests are instantly approved or denied through an automated fraud detection system, potentially fraudulent requests are submitted to an analyst for manual review.
The analyst has a dedicated transaction review tool encompassing all relevant third-party data. Prior to using Neo4j, analysts had to query a Microsoft SQL Server database to review customer history for fraudulent activity.
“It was taking five minutes or more to run a query,” said a product manager for fraud
detection solutions at the company. “And since our analysts were having to review 10,000
daily transactions, this wasn’t sustainable. Also, a relational database wasn’t the right solution
to perform link analysis queries so it placed a huge burden on our database.”
The company needed to find a more efficient way to analyze the data and save time for both
their waiting customers and analysts. Specifically, they needed to decrease the time it took to
process fraud detection queries and provide analysts with simple data visualizations.
That’s when they found Neo4j, which provided real-time results with connected data and
data visualization that enabled analysts to make faster, more accurate decisions. This opened
the door for more extensive searches, which the company hopes to expand from four to 10
degrees of separation.
Company analysts also began noticing clusters and relationships between their data, thereby
uncovering new, previously unnoticed potential fraud connections. This introduced the
possibility of more accurate, real-time fraud ring detection.
Data visualization made possible by Neo4j cut the manual analyst review time in half,
allowing them to stop fraudulent transactions sooner and reduce wait times for non-fraudulent
customers. In addition, they began to see possibilities far beyond their original
data visualization use case.
The company is now integrating Neo4j with their real-time decision platform to instantly stop
fraudulent transactions and save the company thousands of dollars per day.
Conclusion
When it comes to graph-based fraud detection, you need to augment your fraud-detection
capability with link analysis. That being said, two points are clear:
- As business processes become faster and more automated, the time margins for
detecting fraud are narrowing, increasing the need for a real-time solution. - Traditional technologies are not designed to detect elaborate fraud rings. Graph
databases add value through analysis of connected data points.
Graph technology is the ideal enabler for efficient and manageable fraud detection solutions.
From fraud rings and collusive groups to educated criminals operating on their own, graph
database technology uncovers a variety of important fraud patterns – and all in real time.
Next week, in blog two of our series on the top 10 use cases, we will cover real-time recommendations.