Financial Services: The Next Evolutionary Step Is Graph Technology
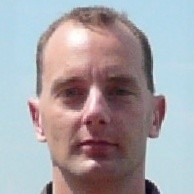
IT Architect & Consulting Engineer, Neo4j
5 min read
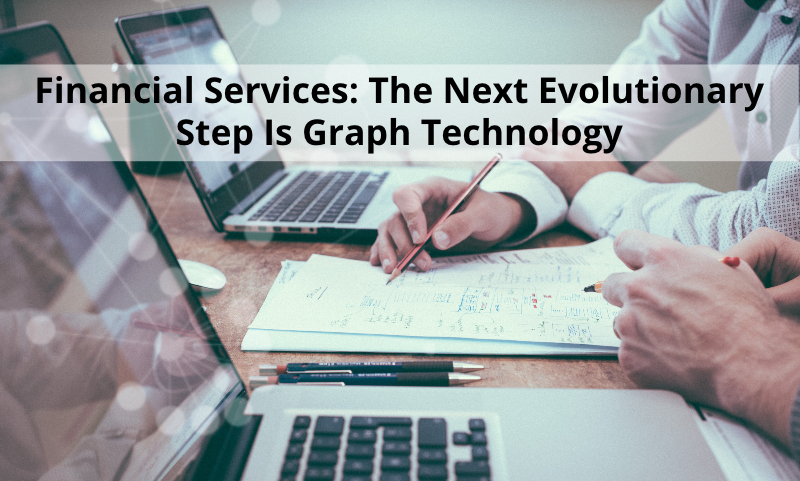
It is common amongst solution vendors to take new technologies and extend their existing financial services solutions with them. Often, these new technologies do not or only partially close existing functionality gaps.
Financial institutions are still required to patch these gaps through alternative means, usually, cost-intensive human manual efforts resulting in higher OPEX spent than necessary.
Neo4j looked at the root causes of those still unresolved gaps first and designed its graph technology to resolve them for good. Neo4j is an evolution born out of real-world need, not an invention. This means Neo4j graph technology does not extend or replace existing solutions – it complements them.
Most financial services issues have their origin either in the payments or transaction monitoring space. Patching these causes an unnecessarily high level of operational expenditure.
Similarly, a reduction of OPEX spent leads to many risk suspicious payments being released unintentionally or a high number of stopped payments, which are not suspicious at all.
Until today, the financial industry has not achieved a synchronised positive effect for all three operational spaces. To achieve this positive effect synchronicity, it is required to fix a single issue in the payments space and a single issue in the transaction monitoring space.
Payments
The payment space – especially the correspondent system – is by its very nature a graph theory operational space, which requires a graph calculation capable solution.
Historically, due to the lack of such an IT solution until the last few years, financial institutions tried to automatically calculate the optimal payment path for a fund transfer from an ordering to a beneficiary financial institution with solutions using relational databases.
Relational databases have never been designed to solve such problems and can’t help, therefore. So it’s up to humans to manually set static payment paths for the majority of payments, even though better fee, compliance and customer service paths are available.
Finding the optimal path for every transaction automatically and lowering the OPEX spent on manual payment message repairs, requires the evaluation of every entity’s condition along each possible path. The combination of entities, which fulfill the transaction service quality requirements and comply with fee, settlement and risk & legal obligations, is the optimal path.
The example shows three available paths to transfer customer funds from an account in France to an account in China within 7 days and a customer service charge of USD 40. Neither path is optimal as each one violates profitability, settlement time, or exposes the financial institution to possible fines from U.S. financial authorities.
A Neo4j graph database enables such complex calculations at scale and in near real time. It has been designed specifically for such a scenario.
Even a small OPEX saving of 1 USD per transaction results in large transaction profitability increases, due to the high number of daily payments. Large financial institutions process double-digit millions of transactions every day.

Transaction Monitoring
The detection of potentially risk suspicious transactions, like fraud, AML, scam, tax evasion, etc.., is performed by matching the entities and their details contained in the transaction’s payment message, e.g. SWIFT MT 103, against entries on watchlists, PEP databases and Negative News.
The challenge is that not each KYC detail contained in the payment message, like sender and beneficiary information, is necessarily complete, correctly spelt or even existent. This makes matching those details against reference lists difficult and is resolved by the “Entity Resolution” capabilities of existing transaction monitoring systems.
However, those systems can only match what they receive by payment messages, and these do not contain the details of entities related to the sender or the beneficiary. In other words, the ultimates. If such an ultimate represents a financial crime risk to the financial institution, then this entity slips through undetected. A false negative.
The usual approach to combat false negatives is to create many and widely scoped matching rules to catch even the slightest possible false negative, which increases the number of transactions filtered out for investigation that are not suspicious at all. False positives.
All filtered out transactions are investigated by Transaction Monitoring Analysts (TMA) who decide to stop or release payments and create a report containing the evidence used for their decision. A TMA needs 20 to 30 minutes to review a case, spending 80% of that time manually searching, connecting and evaluating involved ultimates and their social backgrounds, which link them to the senders and beneficiaries.
This means that each TMA reviews about 20 cases per workday. The lack of an automated ultimates’ detail collection system, known as knowledge graph relating all details to each other and exposing suspicious patterns to the transaction monitoring system for filtering and the TMA for evaluation and reporting purposes, is the main OPEX spent driver, which shows its effects in the high number of required TMA (on average 83) in compliance departments to barely cope with the load of non-investigative review work.
An automated data collection, so the removal of that 80% time spent on manual data collection, reduces the time needed for each case to about 10 minutes.
It also ensures a significantly higher number of truly suspicious, less false-positive cases.
OPEX
Neo4j’s graph database makes a huge operational expenditure difference by resolving two previously unresolved operational areas. Their combined positive effects mean a reduction of up to 0.7% of your total OPEX spent on compliance.
Conclusion
It is not beneficial to acquire the latest and greatest technology first and then without understanding what causes existing gaps trying to figure out how it resolves them. Neo4j developed an operational graph model to complement your existing core banking solutions, which is in live operation for some years now and has proven its effectiveness in resolving those gaps.
Engage with Neo4j’s Professional Services and book your “Graph Financial Services Architecture Workshop” to receive your custom architectural design, explaining how you are going to achieve the same positive results on payments, Transaction Monitoring and OPEX simultaneously.