The Ethics of Generative AI: Understanding the Principles and Risks
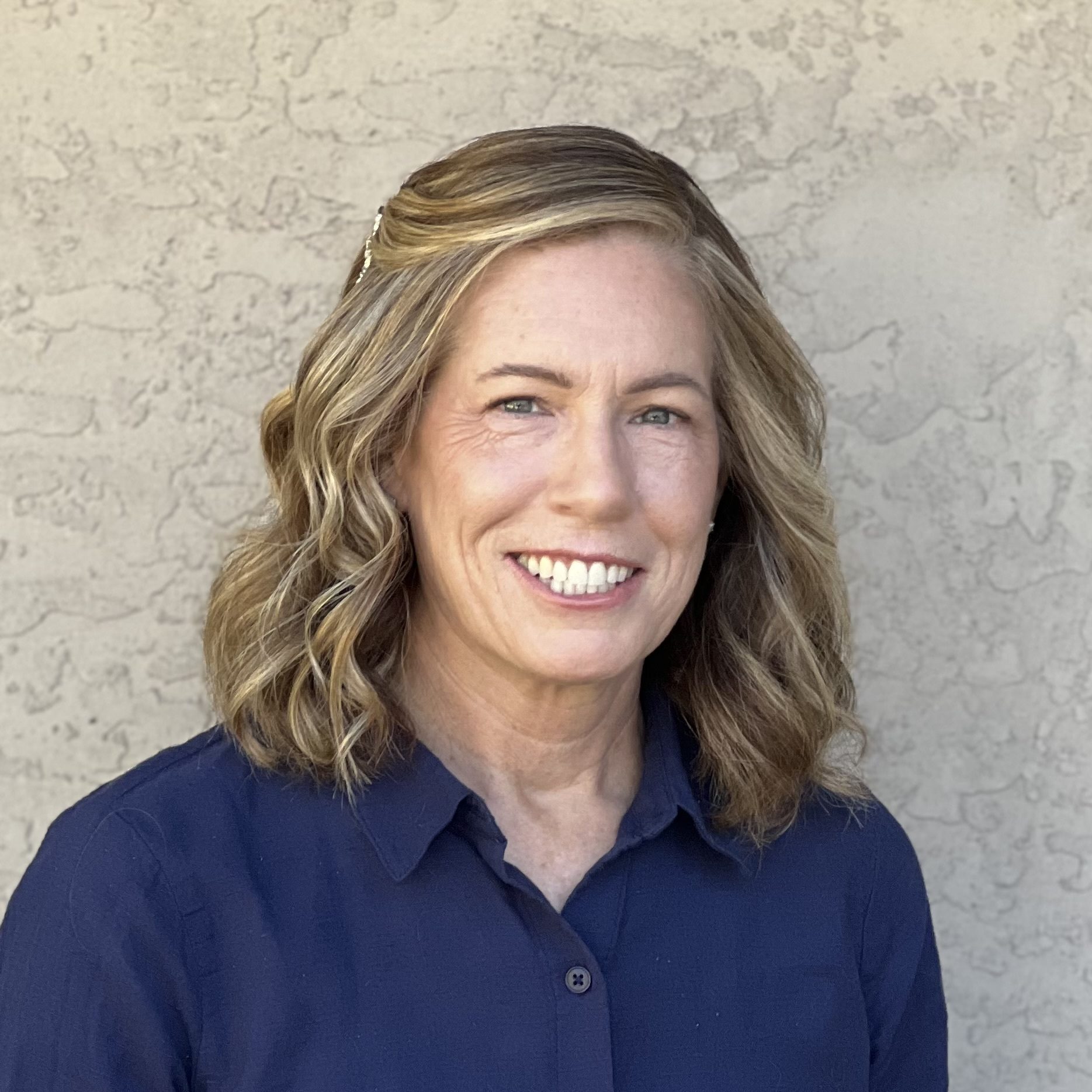
Senior Staff Writer, Neo4j
13 min read
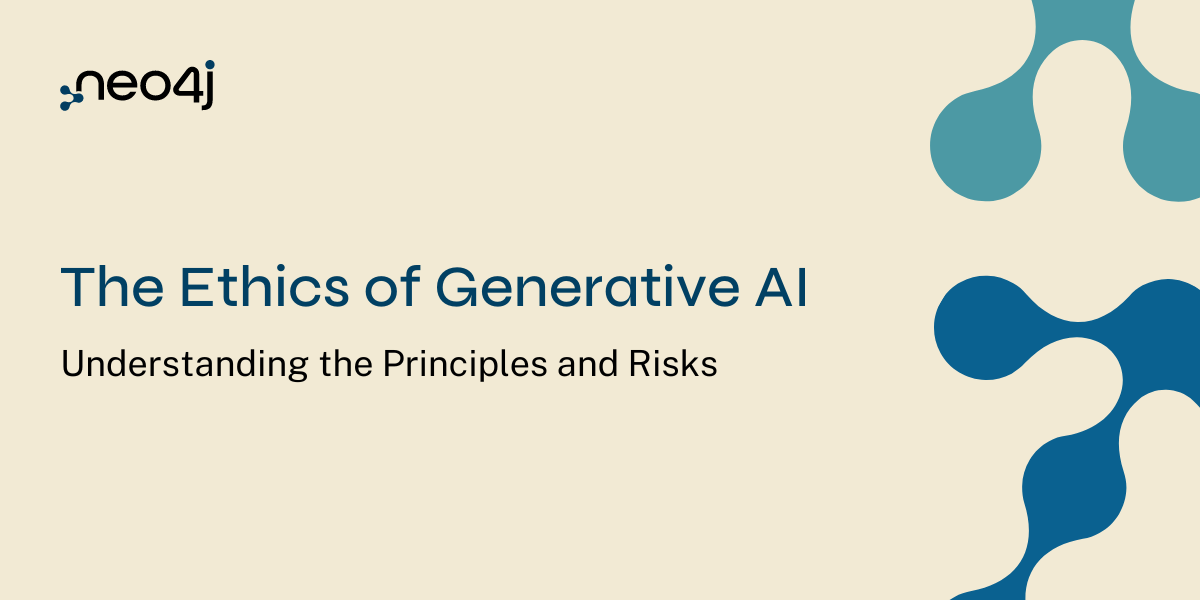
Generative artificial intelligence (GenAI) has taken the business world by storm. According to McKinsey, 65% of organizations are regularly using it. Its advancements are improving use cases in fraud detection, drug discovery, product development, supply chain improvement, customer support, engineering, and more. With its ability to analyze large quantities of data, inform strategic decision-making, streamline processes, and enable innovation, GenAI is transformational for many companies.
Executives, data scientists, and developers all have high expectations for generative AI technology, but a lack of ethical and responsible AI practices can interfere with implementation. As industry practitioners recognize GenAI’s potential to enhance mission-critical systems, they also face the challenge of addressing and managing ethical implications and associated risks.
In this blog post, we look at the current state of generative AI ethics, including the latest guidelines and legislation, along with some concrete ways to incorporate ethical best practices.
- The GenAI Achilles’ Heel
- Generative AI: The Wild West
- Ethically Questionable Opportunism
- Addressing Ethical Concerns
- Principles of Ethical AI
- Help from NIST
- Applying the Principles
- Add Responsible AI to Your Tech Stack
The GenAI Achilles’ Heel
Chief among ethical considerations is GenAI’s tendency to hallucinate, providing an inaccurate or nonsensical answer derived from its large language model (LLM) content.
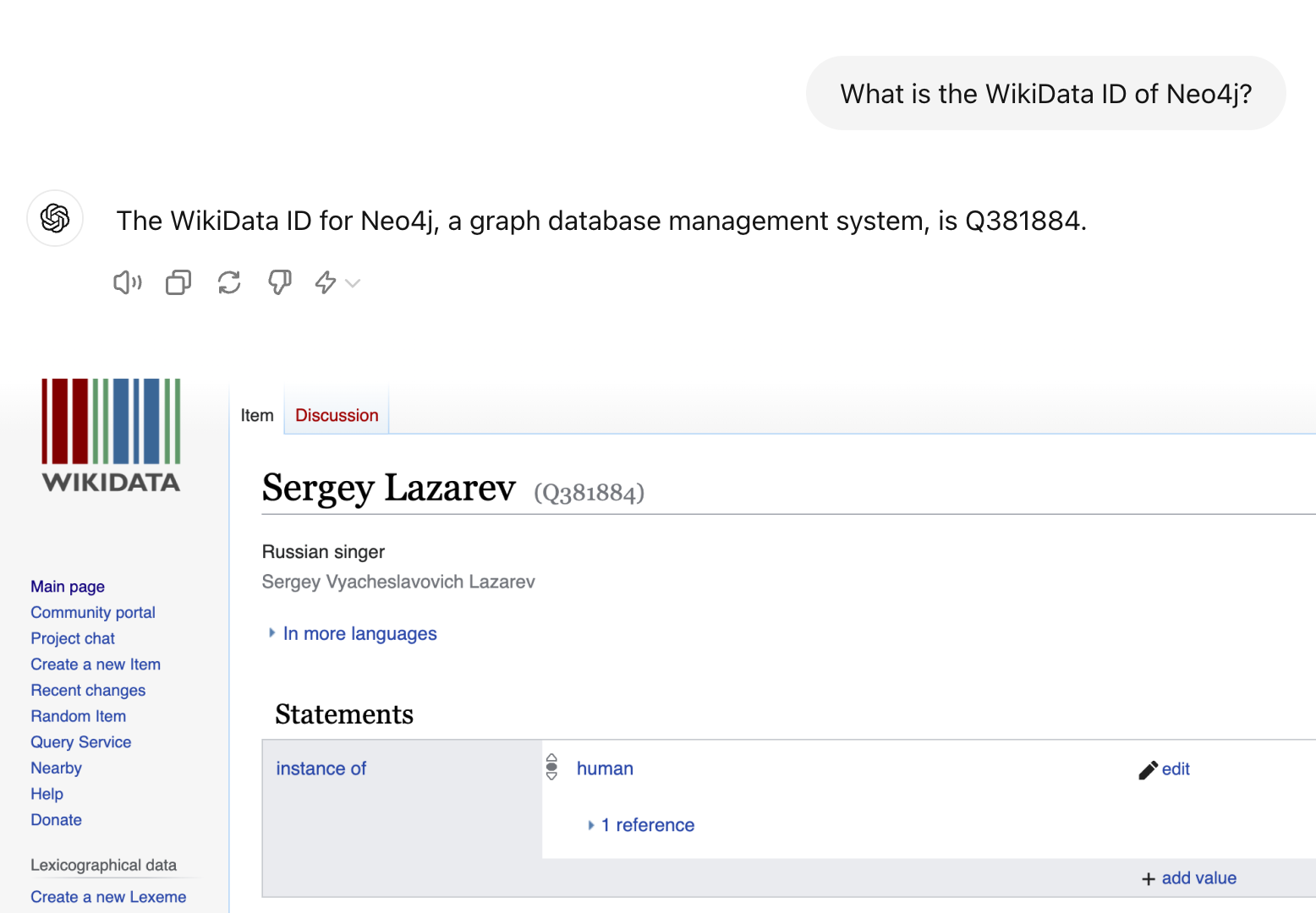
An example of ChatGPT’s hallucination.
Why does GenAI hallucinate? LLMs are trained to prioritize human-like answers, even if they aren’t correct. Hallucinations may also result from poor data quality, model limitations, and sole reliance on pattern recognition, which interferes with full understanding.
Hallucination is an obvious ethical problem for AI because it means generated information isn’t guaranteed to be correct. When a generative AI model hallucinates, and someone makes a real-world decision based on the information, the consequences can be far-reaching. For instance, if an AI-powered chatbot provides an erroneous answer to a consumer’s question, the incident could cause the consumer to accidentally spread misinformation and also damage the company’s reputation. Researchers, data scientists, and developers are, of course, avidly looking for ways to reduce hallucination, but so far, even when using generative AI with high-quality LLM training data, this phenomenon can be challenging to eliminate.
With GenAI, businesses are also concerned about::
- The fact that answers may lack domain-specific knowledge, or “context”
- An inability to trace, verify, and explain how algorithmic responses are derived
- The possibility of biased output that could perpetuate discrimination
- The risk of leaking sensitive data
- Keeping up with changing legal ramifications of using the technology
While GenAI is still considered a breakthrough technology that holds immense promise across industries, some company leaders feel that they can’t yet rely on it, let alone incorporate ethical best practices.
Generative AI: The Wild West
Ushering in a bold new tech era, GenAI draws parallels to the American Wild West. Its pioneers, ranging from startups to established enterprises, are pushing forward with innovative applications, staking claims, and developing swaths of territory in a relatively unchecked environment.
In this land of opportunity, many corporate trailblazers want to do the right thing, such as building in checks and balances when designing GenAI algorithms. CIOs and other stakeholders making assessments about GenAI are keenly aware of the need to mitigate any ethical risks. Company leaders believe they have a duty to guide development and application in ways that incorporate protections and engender public trust, while still fostering innovation and success. AI-focused companies such as Google and Microsoft have drafted best practices for using AI responsibly, and they’re actively proposing and testing prospective solutions.
This abundance of good-faith responses represents a strong start toward creating ethical GenAI frameworks that can help companies succeed while also benefiting society as a whole, but there’s more to be done.
Ethically Questionable Opportunism
Early applications of GenAI have brought attention to some worrisome activity:
- AI creation of “original” writing, music, and artwork. GenAI applications have copied proprietary material found in LLM data sets without providing attributions or obtaining permissions, resulting in copyright infringements of authors’, musicians’, and artists’ work. Intellectual property challenges are progressing.
- Creation of deepfakes, doctored videos and audio clips that pass as authentic. Deepfakes are easy to make and tough to detect. They facilitate identity theft, and, when released on social media, can do things like influence elections. “The ease of creating AI-powered tools might lead to large-scale automated attacks,” warns venture capitalist Alon Hillel-Tuch. Some good news: California is banning deepfakes, and OpenAI has built software to help detect those created by DALL-E.
- Empowerment of scammers through creation of deepfakes and availability of various applications.
These “Wild West” manifestations of GenAI prowess bolster the case for formalizing ethical approaches and establishing regulations as needed.
Addressing Ethical Concerns
At the societal-well-being level, as well as the day-to-day corporate level, can we put guardrails on certain aspects of GenAI and resolve the ethical challenges? World leaders are focused on creating and releasing universal legal frameworks. Some ethics-oriented proposals to date are controversial, but there’s growing momentum to adopt rules.
Most notably, the European Union recently passed the Artificial Intelligence Act, the first comprehensive global regulatory framework for AI. This legislation distinguishes AI from traditional software, defining it as “a machine-based system that is designed to operate with varying levels of autonomy and that may exhibit adaptiveness after deployment, and that, for explicit or implicit objectives, infers, from the input it receives, how to generate outputs such as predictions, content, recommendations, or decisions that can influence physical or virtual environments.”
In addition to Europe, this sweeping law applies to United States companies that have global reach, spanning AI development, use, importation, and distribution in the European market, as well as to American companies that produce output for use in the EU market.
Targeting High-Risk AI Systems
According to CPO, The AI Act is focused on “high-risk AI systems” related to either regulated products or associated safety components, including vehicles, medical devices, aircraft, toys, and machinery.
Applicable use cases include:
- Biometric remote identification, biometric categorization, or emotion recognition
- Critical infrastructure
- Education and vocational training
- Employment, workers management, and access to self-employment
- Essential private services, and essential public services and benefits
- Law enforcement
- Migration, asylum, and border control management
- Administration of justice and democratic processes (e.g., election)
In addition to the AI Act, American companies must comply with broad-level existing regulations that could have implications for using AI, such as equal employment opportunity laws. For example, the EEOC is scrutinizing generative AI tools that could create discrimination in the hiring process, such as résumé scanners that elevate particular keywords and applicant rating systems that assess factors such as personality, aptitude, cognitive abilities, and expected cultural fit.
How can a company that uses GenAI technology for mission-critical projects move forward in an ethically acceptable way? Here are some guiding ethical principles, followed by practical steps executives, data scientists, and developers can take to get on track.
Principles of Ethical AI
A first step for applying ethics to GenAI has been to illuminate human-centered principles of good behavior as they may apply to GenAI. Under that lens, ethics-oriented guidelines include:
Do No Harm
Consumers are reminded by spiritual leaders, psychologists, and businesses to do no harm, be kind and good, go in peace, and live and let live. Company executives hear similar directives for steering their organizations.
When it comes to using GenAI, how can a company strive to be a model corporate citizen? For starters, teams working with GenAI technology can:
- Monitor AI output and avoid releasing misinformation
- Get feedback to assess whether output could be detrimental
- When making decisions that impact people, avoid physical, mental, or emotional harm
- Learn about GraphRAG, a technique that enhances the accuracy and reliability of GenAI models
- Seek ways to build credibility and sustainability of GenAI technology
Be Fair
Fairness is treating everyone equally regardless of race, gender, socioeconomic status, and other factors.
Being fair sounds fairly straightforward, but GenAI processes may inadvertently introduce or perpetuate data bias. For example:
- If a car safety test is conducted with only men drivers — typically larger-framed people — then, regardless of any fairness instructions given to the AI, the resulting data will be biased against women and other small-framed individuals.
- When programming self-driving cars, it’s not easy to make morally perfect decisions.
- An AI model trained on material generated by biased AI can exaggerate stereotypes.
In LLM design, eliminating data bias that favors or disfavors certain groups of people is often easier said than done. A developer can instruct AI to be unbiased, and then follow up to root out any bias that snuck through, but that may not be enough. With the best of intentions, say, by favoring one category of sensitive personal data, a company may still discriminate, as evidenced by Google Gemini. “For SMEs relying on ChatGPT and similar language models for customer interactions, there’s a risk of unintentional bias affecting their brand,” notes industry expert Brad Drysdale.
Companies can promote GenAI fairness by:
- Monitoring and testing AI outcomes for group disparities. The National Institute of Standards and Technology (NIST) supports a “socio-technical” approach to mitigating bias in AI, recognizing the importance of operating in a larger social context and that “purely technically based efforts to solve the problem of bias will come up short.”
- Using diverse, relevant training data to populate an LLM.
- Including a variety of team input during model development.
- Embedding data context in graphs, which can ensure more fair treatment. For example, Qualicorp, a Brazil-based healthcare benefits administrator, has created a graph-based AI application that helps prevent information omission for insurance applicants. In this ethical use case, context provided in a graph database makes it easier to treat people as individuals instead of as a single, homogenous group.
Ensure Data Privacy
With awareness of widespread corporate data breaches, consumers have become advocates for stronger cybersecurity. GenAI models are in the spotlight because they often collect personal information. And consumers’ sensitive data isn’t the only type at risk: 15% of employees put company data in ChatGPT, where it becomes public.
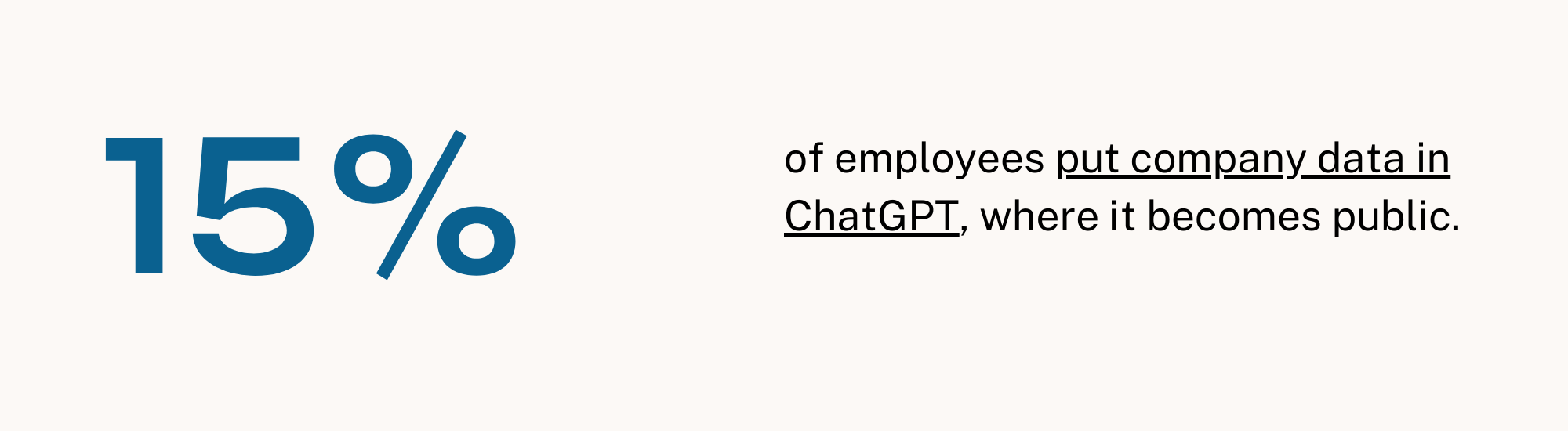
Most company leaders understand that they must do more to reassure their customers that data is used for legitimate purposes. In response to the need for stronger data security, according to Cisco, 63% of organizations have placed limits on which data can be entered digitally, while 61% are limiting the GenAI tools employees are allowed to use.
Executives and developers can protect sensitive data by:
- Setting up strong enterprise defenses
- Using robust encryption for data storage
- Using only zero- or first-party data for GenAI tasks
- Denying LLMs access to sensitive information
- Processing only necessary data (a GDPR principle)
- Anonymizing user data
- Fine-tuning models for particular tasks
Honor Human Autonomy
Applied to AI, what does this lofty directive mean?
In certain contexts, notes Frontiers in Artificial Intelligence, AI technologies threaten human autonomy by “over-optimizing the workflow, hyper-personalization, or by not giving users sufficient choice, control, or decision-making opportunities.” Respecting autonomy alludes to maintaining the natural order of what humans do, such as making choices. For example, with critical decision-making about healthcare, the buck should stop with medical professionals.
When using GenAI, companies can strive to respect human autonomy by:
- Being ethically minded when nurturing talent for developing and using GenAI
Be Accurate
Inaccurate information from LLMs that finds its way into the world is a pressing concern. Inaccurate “facts” end up in everything from search results detailing the history of Mars to legal briefs.
Aside from fact-checking, how can a company ensure the accuracy of its LLM-sourced information? To help catch GenAI hallucinations and improve your LLM responses, you can use a technique called retrieval augmented generation (RAG). Informed by vector search, RAG gathers relevant information from external data sources to provide your GenAI with an authoritative knowledge base. By adding a final key ingredient, knowledge graphs, which “codify” an LLM’s facts, you can provide more context and structure than you could with metadata alone. RAG techniques that can reduce hallucinations are also continuing to evolve. One application is GraphRAG.
Source: Generative AI Benchmark: Increasing the Accuracy of LLMs in the Enterprise with a Knowledge Graph.
“By using a combination of vector search, RAG, and knowledge graph interfaces, we can synthesize the human, rich, contextual understanding of a concept with the more foundational ‘understanding’ a computer (LLM) can achieve,” explains Jim Webber, chief scientist at Neo4j, the preferred data store for RAG over vector databases and other alternatives.
Be Transparent
When a consumer applies for a loan from a bank and is turned down as the result of a GenAI process, does management understand how the decision was made? If a job applicant is rejected based on their resume, is the reason easily identifiable? GenAI models can be “black boxes” in which the algorithmic processing is hidden or obscured.
Being unable to pinpoint what’s behind algorithmic decisions can lead to public distrust of AI, which is why ethical AI proponents are pushing for companies to provide transparency. To identify what data was used in GenAI decision processing, a system can provide context — the peripheral information — which facilitates understanding of the pathways of logic processing. Explicitly incorporating context ensures that the technology doesn’t violate ethical principles. One way for a company to enhance transparency is to incorporate context by using a graph database such as Neo4j.
Be Explainable
With a bank denying a loan based on an algorithm and no way to trace the decision to an origination point, what does the loan officer tell the applicant? Saying “We have no idea why our AI did that, but we’re standing by it” isn’t going to go over well. In addition to damaging trust, not being able to explain how the decision is justified can hinder further application adoption.
In light of this dilemma, the subfield of explainable AI (XAI), the ability to verify, trace, and explain how responses are derived, has emerged.
Explainability has four components:
- Being able to cite sources and provide links in a response to a user prompt
- Understanding the reasoning for using certain information
- Understanding patterns in the “grounding” source data
- Explaining the retrieval logic: how the system selected its source information
How can companies adapt their GenAI processes for this aspect of ethical operation? To build in explainability, tech leaders must identify training provenance. “You need a way to show the decision-owner information about individual inputs in the most detail possible,” says Philip Rathle, chief technology officer at Neo4j.
To that end, a company can use knowledge graphs and metadata tagging to allow for backward tracing to show how generated content was created. By storing connections between data points, linking data directly to sources, and including traceable evidence, knowledge graphs facilitate LLM data governance. For instance, if a company board member were to ask a GenAI chatbot for a summary of an HR policy for a specific geographic region, a model based on a knowledge graph could provide not just a response but the source content consulted.
Be Accountable
With ethical AI, the thinking goes, humans are typically held accountable for their decisions, so logically, generative AI systems should be, too. If there’s an error in an algorithm’s decision process, it’s imperative for the company to step up and be accountable. Acknowledging the issue, determining whether a change must be made, and, if so, making the change helps protect a company’s reputation. Companies can also stand behind their GenAI activity by building in accountability.
Help from NIST
When pairing proprietary data with GenAI, applying these principles may seem relatively straightforward. However, because AI ethics guidelines and regulations are dynamically evolving, executives and developers who want to ensure ethical operation aren’t always sure of the best or most acceptable course of action.
To help with this challenge, NIST has drafted formal AI ethical guidelines. The organization first requested input from stakeholders across the country to create a plan for development of technical standards and related tools to support reliable, trustworthy AI systems.
Among the key contributors, graph database company Neo4j called for recognition of ethical principles as the foundation of AI risk management. Neo4j suggested that for AI to be situationally appropriate and “learn” in a way that leverages adjacency to understand and refine outputs, it must be underpinned by context. The company suggested that these principles be embedded in AI at the design stage, as well as defined in operational terms according to use case and implementation context. For example, fairness might be defined as group fairness (equal representation of groups) or procedural fairness (treating every individual the same way).
NIST followed its input stage by drafting an AI risk-management framework promoting fairness, transparency, and accountability. If you want to design and deploy ethically oriented AI systems, this document is a great place to start.
Applying the Principles
How can executives, data scientists, and developers start applying ethical principles and building generative AI tools on a firm foundation that can expediently adapt to a changing regulatory environment?
For starters, they can:
- Ask whether the use of generative AI truly makes sense for the application: Is it the most appropriate tool?
- When selecting a technology stack, keep your strategic requirements in focus
- Apply ethics to GenAI at the design stage to incorporate transparency
- Establish a risk-based system to review output for bias
- Set up a process to handle any discovered bias
- Teach staff to verify GenAI outputs and report suspect results
- Fact-check to make sure AI-generated information is accurate
- Ensure that private data stays safe
- Use graph databases, which incorporate data context to facilitate ethical outcomes
- Research new techniques that reduce hallucinations and factually ground data
Add Responsible AI to Your Tech Stack
Looking to build ethically oriented generative AI applications but wary of wading into an ethical AI sinkhole?
Neo4j graph database technology is a proven, reliable way forward. Our knowledge graphs enable GenAI to understand complex relationships, enhancing the quality of your output. With our graph retrieval augmented generation (GraphRAG) technology, you can confidently put AI ethical principles into practice.
With Neo4j, you can:
- Make your machine learning model outcomes more trustworthy and robust by giving your data meaningful context
- Provide a comprehensive, accurate view of data created by the connections in a knowledge graph
- Improve your LLM responses by using RAG (retrieval augmented generation) to retrieve relevant source information from external data stores
- Explain your retrieval logic: Trace your sources and understand the connections between knowledge and responses
- Improve data quality, transparency, and explainability as a result of generating more relevant information
- Integrate domain knowledge: Incorporate and connect your organizational data and facts for accurate, tailored responses
- Accelerate your GenAI development: build and deploy quickly with frameworks and flexible architecture
Get the details on how you can build responsible GenAI applications today!