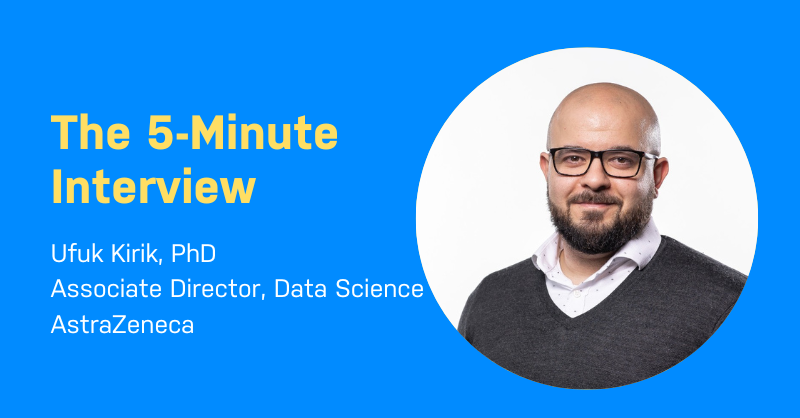
We had the chance to chat with Ufuk for a 5-Minute Interview, which we’re excited to share with you now.
Enjoy learning more about how this bright mind has harnessed the power of connected data for advancements in the pharmaceutical space, and hear his thoughts on what the future has in store for graph technology.
Please introduce yourself.
Ufuk Kirik: My name is Ufuk Kirik, I work at AstraZeneca Pharmaceuticals. We work with the data science team underneath pharmaceuticals R&D. Our big task is to identify new drug targets using graph data science.
Why did you choose Neo4j?
Ufuk Kirik: Well, it’s one of the most mature products out there, but it’s also the product that was used by our external stakeholders, our external collaborators. So it was both a natural choice and a happy coincidence as well.
Do you have any advice for someone getting started with Neo4j?
Ufuk Kirik: Absolutely, sure. First of all, don’t be afraid. Don’t panic in the name of “Hitchhiker’s Guide to the Galaxy.” Dip your toes in the water. Pay attention to graph model, but don’t be a perfectionist. There’s a lot of really good documentation out there. There’s a lot of experts who are willing to share their expertise. Use them and don’t be shy and try it out.
What are some surprising results you’ve seen from using Neo4j?
Ufuk Kirik: What I really, really like about Neo4j, as opposed to similar technologies elsewhere, is that Cypher makes translating difficult biological questions into queries very, very simple – it’s almost trivial.
So you can actually, while you’re writing a query, you almost see the path that your query is going to take. And that allows you to then sort of associate different types of entities with types of relationships fairly easily, as opposed to writing JOINs and all of these different things. So it’s quite intuitive both in terms of designing and writing the queries and also while troubleshooting.
What do you think is in store for the future of graph technology?
Ufuk Kirik: I think as the technologies mature, we’ll see more and more examples and libraries building on top of Neo4j and on top of similar technologies out there. We’ll be able to hook in different things, bring in all of the processing power and exclusivity that comes from Python, from other high level programming languages. We’ll be able to do a lot of things, which quite frankly was not possible before, as well as hooking data from all sorts of different places.
So ideally in a world – I mean, this is an expression that’s thrown out a lot – but a much more agile environment in which you can work, not only in terms, but also in effect, where you can adapt your data model really quickly, and you can sort of develop things very, very quickly.
Discover More Life Science Use Cases
Life Sciences