Knowledge Graphs & Navigating the Future of AI: An Interview with Charlie Beveridge of Accenture
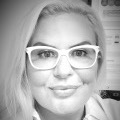
Partner Marketing Lead for the Solution Partners, Neo4j
7 min read
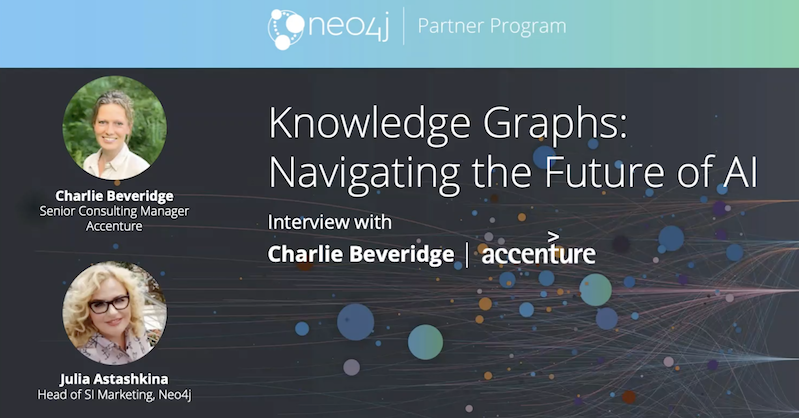
I’m thrilled to have Charlie Beveridge with us for a 5-minute interview on the future of knowledge graphs. Charlie is a Senior Consulting Manager at Accenture and leads the company’s AI propositions for clients in the Telco and Media industries.
Carlie works with client teams in both the front and back office spaces to help them take advantage of the things new technologies are enabling them to do. And, knowledge graphs are one of those technologies she’s seeing have the greatest impact for Accenture’s clients.
Julia Astashkina: So, what is a knowledge graph?
Charlie Beveridge: So normally a graph means a couple of X and Y axes, which measures certain things like volume or time. But the sort of graphs we’re talking about here is 3D, and its 3D axes don’t even necessarily measure anything. They’re just a way to give dimensions to or draw out the space you’re about to fill.
And if you can imagine a number of points or dots representing, for example, LinkedIn profiles, and they’re laid out anywhere within that 3D space, and each point or profile has lines coming from it that connects it to one or more other points or profiles, which in turn have their own lines connecting to other profiles and so on… Really the only things that are important are what each of those points on the graph represents, which other points they’re connected to and then, how close or far apart those connections are. That’s a knowledge graph. Social or professional networks like LinkedIn are a pretty obvious network example.
But basically everything in your life is a part of a network. You take a network of roads when you drive. Your household products went through a network of some supply logistics to get there. Even our knowledge is a network of concepts that we’ve built up over time. Um, once you set up any network on a graph, there are so many more things you can do with that data, and AI in particular can have a much, much greater impact when it’s using data organized into graph format.
Julia: What excites you most about graphs?
Charlie: Well, in a single sentence: Graphs are doing for AI what electricity did for the world. And that might be really strong, but they’re opening up entirely new use cases for AI and they’re radically shifting what’s possible.
There’s a major difference between big data and knowledge. You can have a ton of data points in a big data sea of information. For example, all the people who visited a theme park in a year, which you could aggregate up into things like age or gender, to know how many of your visitors were, say, male thirty-somethings. But it’s another level entirely if you can have connected data points, which provide real insight, because they pull together the relevant context. For example, knowing who came together to that theme park, or who came after their friend who recently visited recommended they should go, or who expanded to another person in the group, the second time they came.
Connections between data points can give you the context behind data, like knowing who is actively increasing your visitor base by bringing their friends along because they just love your theme park so much. And drawing connections is the essence of what a graph is doing to the data points you give it. It’s creating connecting relationships so that everything hangs together into meaningful context or a storyline. And the data that means something is data you can do something much more useful with.
The exciting part is the graph means that AI can use data that is all already connected together with all of its context and meaningful storylines. This means it can make much more useful decisions. For example, AI can learn to target you with the best kinds of offers to reward you for advocating that theme park to your friends, which is a much better strategy than the theme park just blanket targeting you with comms they believe are right for your age and gender.
Julia: Right, very interesting. Do you have any more examples of why it is important to use the whole context for AI?
Charlie: Absolutely, there are so many.
Imagine an AI that’s reading what you type and responding to you – maybe a customer service chatbot on your bank’s website. The English language has evolved to use the same words, and even the same phrases, to mean entirely different things. And when we speak, we can clarify the meaning we intend through the use of tone or gesture. But when we type, there are far fewer clues for the recipient to use to decipher the meaning. It’s like when you read a WhatsApp for a second time and you realize it meant something entirely different than how you originally read it. Where on both reads your brain is using all of the context available to it at the time in order to determine what to understand from the facts. It’s linking words and sentences and previous words and sentences in the threads, as well as knowledge of who the sender is, and even things like time of day, and it’s getting to a conclusion on what the latest text means.
Now, for your bank’s chatbot, if it’s built only to read your question at face value and provide an answer, then it’s likely gonna miss the whole context and therefore misinterpret the question, which wouldn’t make it very helpful. But if a graph can tie together all of the clues, like time of day, and links between words and sentences and information about the sender and so on, then the chatbot has a much more brain-like way of understanding what you’re asking and then responding appropriately.
Another good example is getting to the root cause of an issue. So Toyota actually created the “Five Whys” mantra, as part of its manufacturing methodology, where, asking why five times focused them on fixing the cause and not just putting a temporary plaster over any symptoms. Why is the car not starting? The battery is dead. Why is the battery dead? The alternator isn’t working. Why? It’s belt is broken. Why? The belt is at the end of its life but it wasn’t replaced. Why wasn’t it replaced? The car wasn’t serviced when it was supposed to be.
Okay so, we don’t need to investigate a line of cars for faulty batteries then. If you’ve got a relatively new car, then it’s probably got some kind of onboard diagnostic system, which is constantly monitoring how the car is performing and whether any part of it is broken. And the newer the car, the more likely it’s using AI in those diagnostics. But without the ability for the computer to link together symptoms, and chains of connected parts, and the root cause or root causes plural, it’s never going to be able to accurately present to you what really needs fixing.
So in this sense, graphs would enable AI to ask the Five Whys and that example focused on car faults, but really this deep analysis technique is important for any chain of events that leads to a bolt in anything. You could have burst pipes in an energy network, or a mast issue in a telco network or even faulty machines in the supply chain network. Graphs are helping AI determine the real root cause.
Julia: And what does this mean for AI in the future?
Charlie: The thing with AI is that, so far, we’ve not seen it do much, if any, of the scary stuff we see it doing in films. We don’t have friends who are robots, at least not yet. And that’s because the narrow forms of AI we’ve developed are using specific datasets. Often big data datasets to complete specific tasks, albeit learning over time, how to do those tasks better.
AI is really great at what it does, but what it does has been limited by the lack of context and the data that fuels it. And the thing that we have as humans is the innate ability to draw all the connections we need to interpret, to understand, to learn new things, and even to invent things. Like using one language to learn another, or like learning about history through watching theater productions, or even like, doing things like studying bird wing shapes as the Wright Brothers did to invent aircraft flights.
This ability to draw connections and comparisons make our brains the most powerful on earth. And that sort of creativity has been key to our survival and evolution as a species. So, we talked about how graphs are the glue that connects data in a way that creates meaning out of it. We’ve seen how that connected context, in turn, enables AI to achieve much more powerful outcomes for us. Like identifying root causes, and like targeting relevant rewards, or answering your questions more effectively.
The ability to make connections like humans do naturally is gonna really continue to change the game, in terms of what AI is able to achieve. I almost hate to say it but, it’s going to mean AI could be creative.
Julia: Wow. Well, thank you very much. This has been very insightful!