Neo4j Blog
Neo4j Named a 2025 Gartner® Peer Insights™ Customers’ Choice for Cloud Database Management Systems
We are proud to be named a 2025 Gartner® Peer Insights™ Customers’ Choice for Cloud Database Management Systems.
5 min read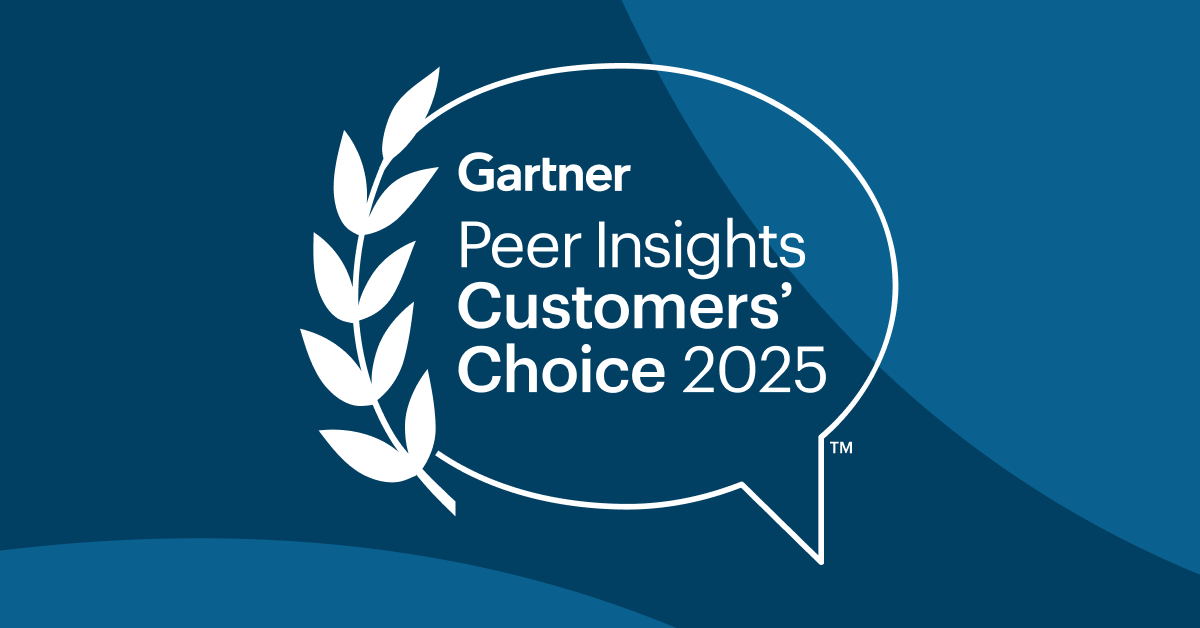
Featured Voices
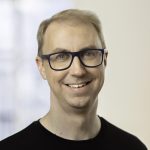
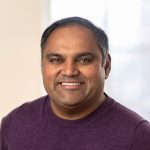
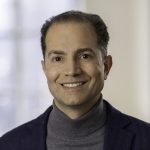
Most Recent
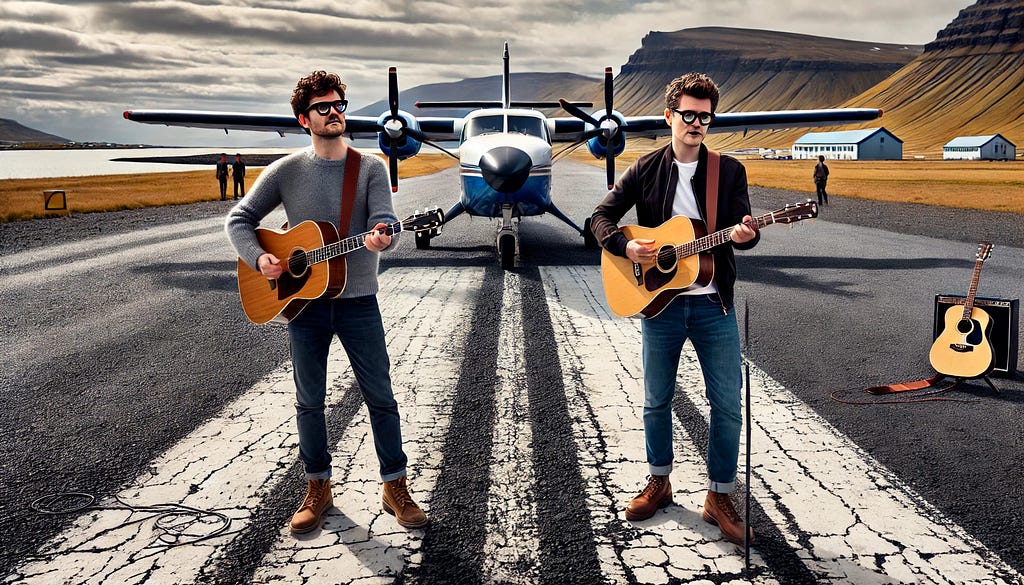
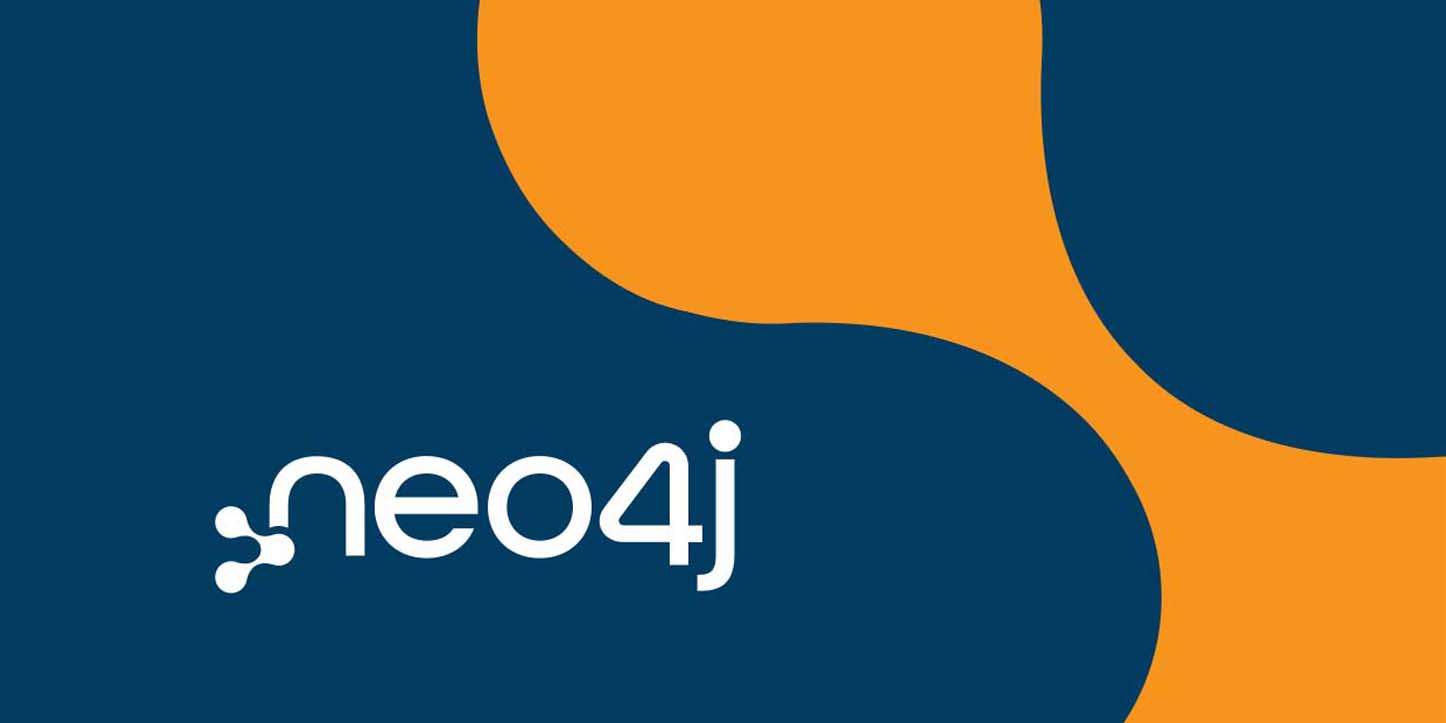
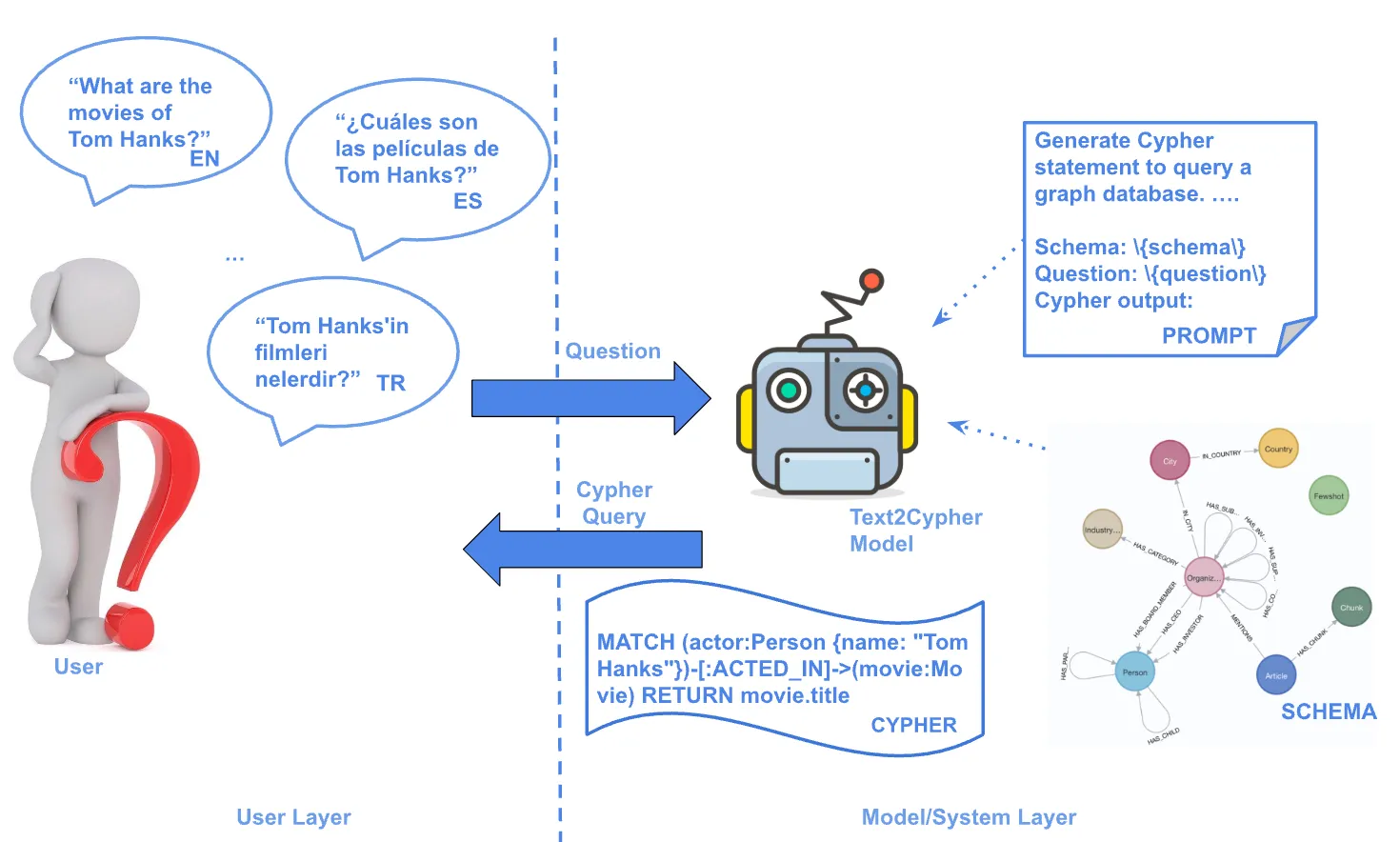
Text2Cypher Across Languages: Evaluating Foundational Models Beyond English
5 min read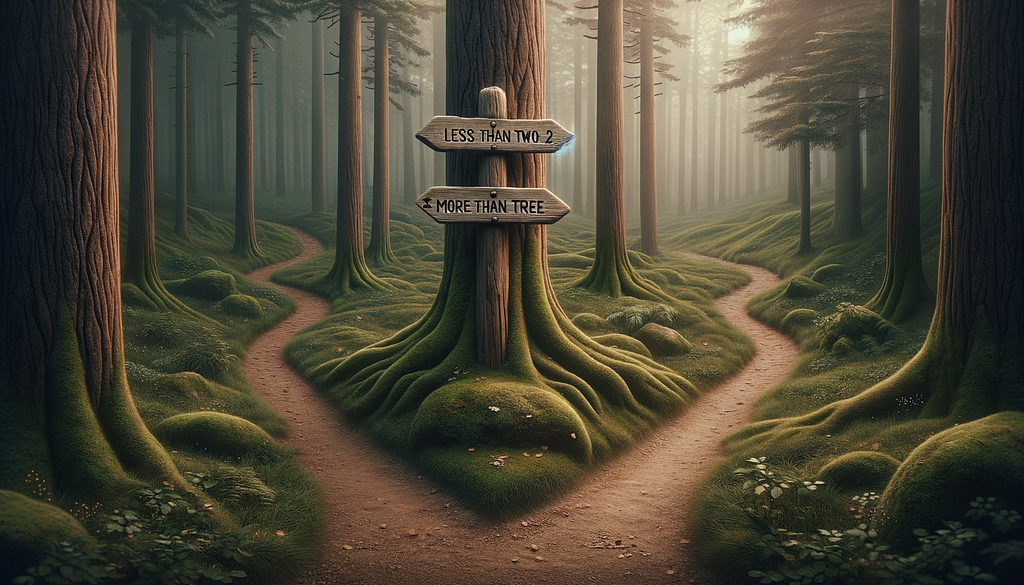
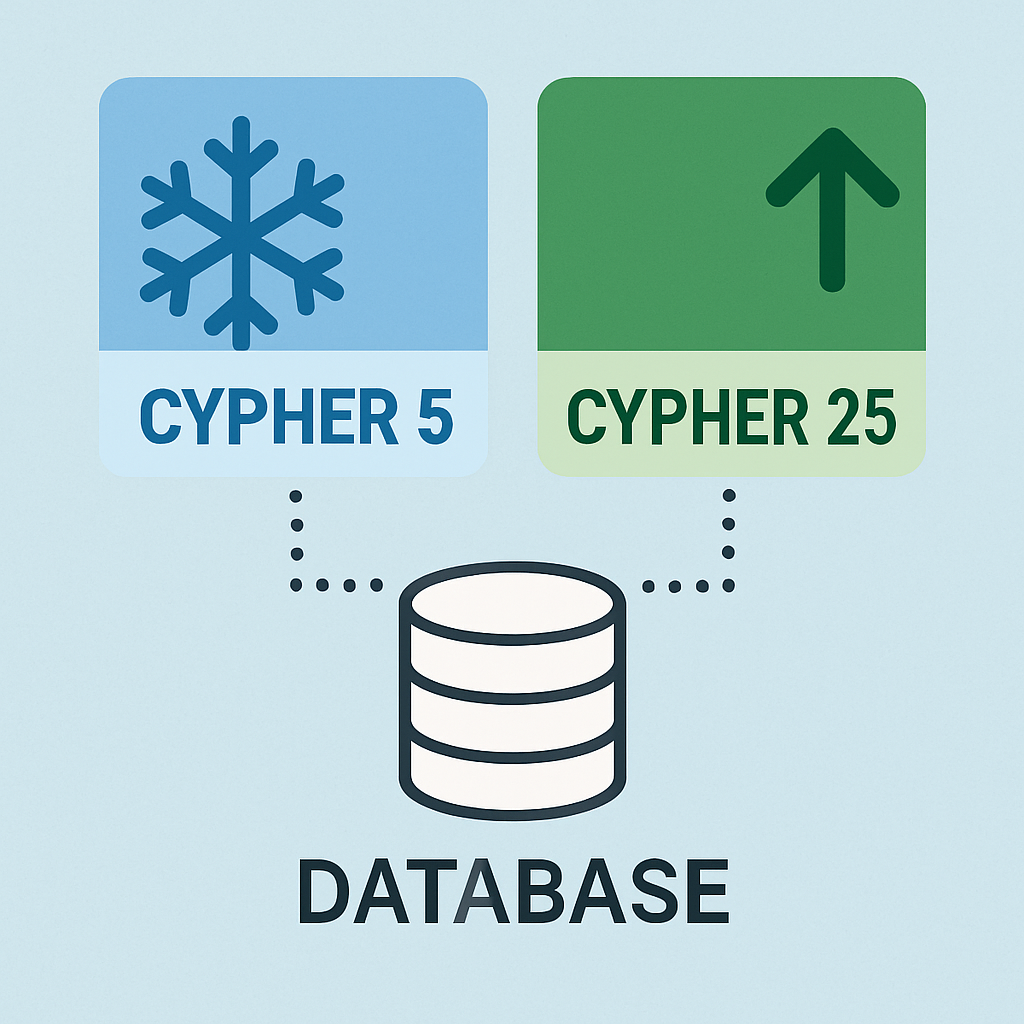
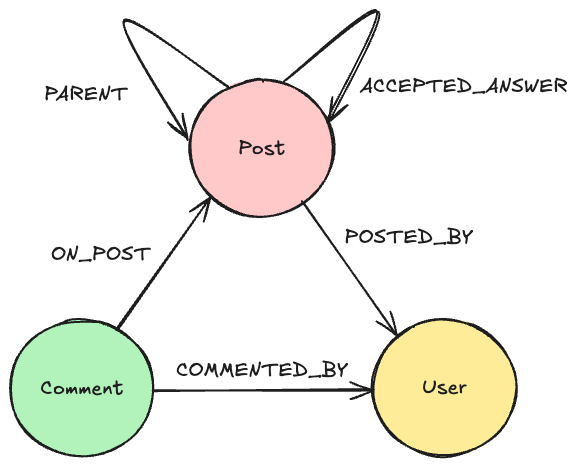
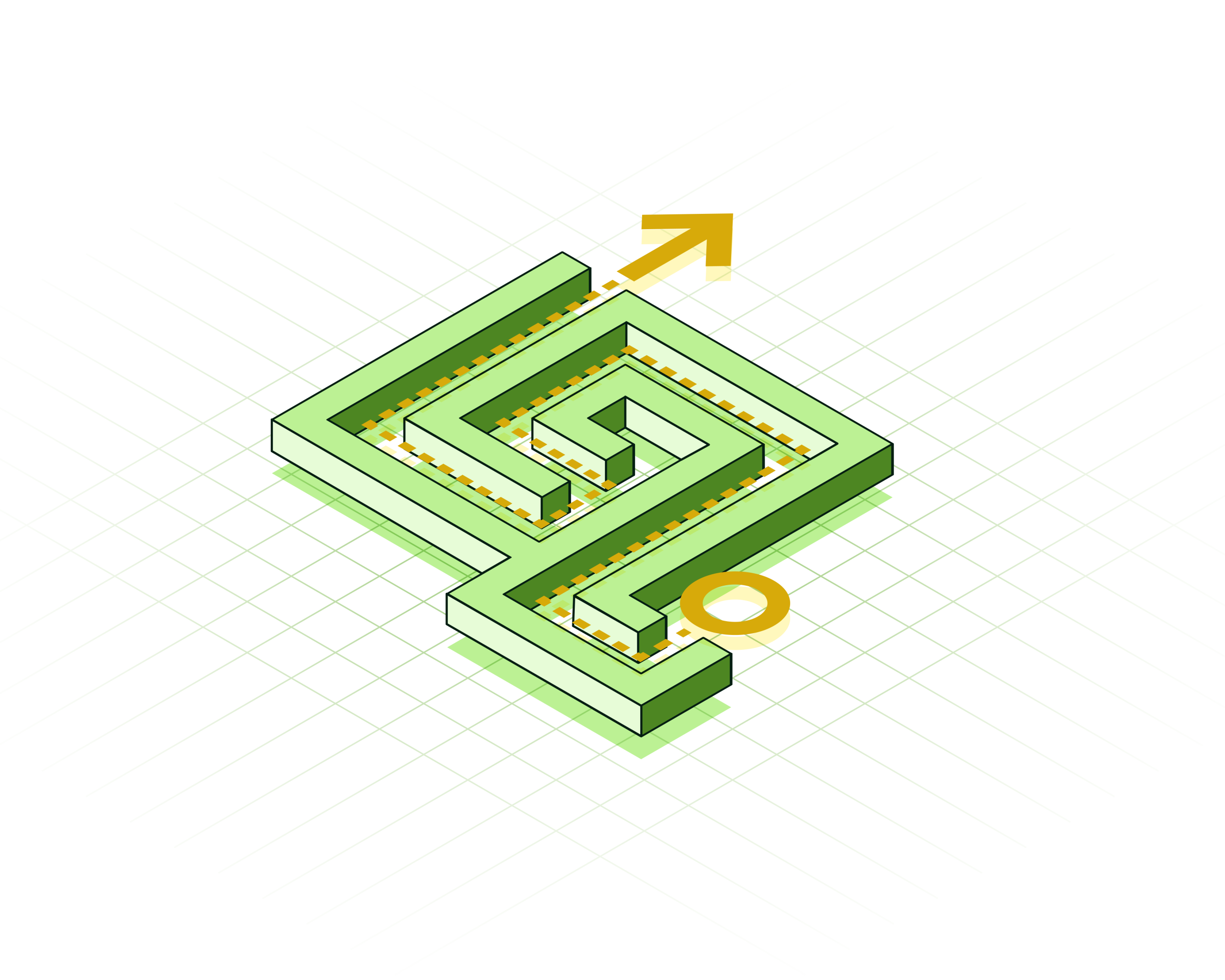
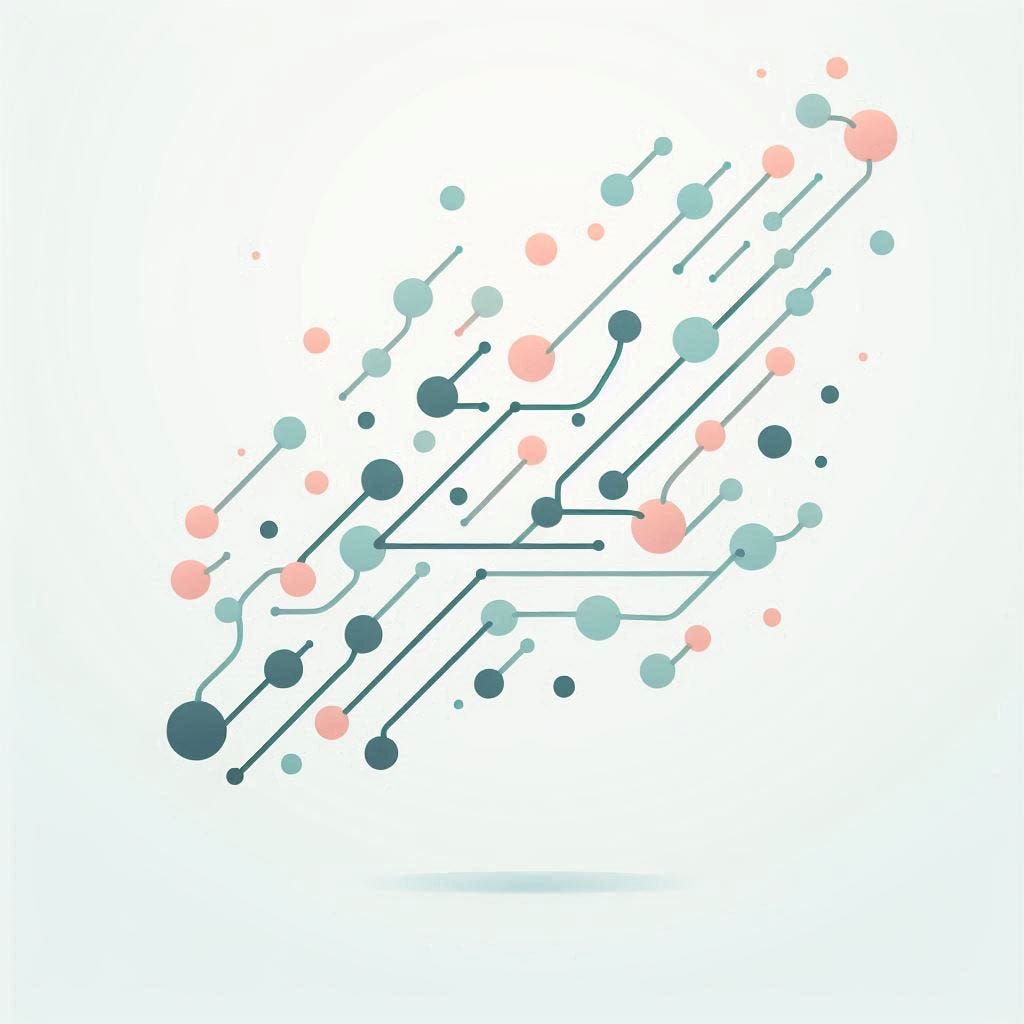
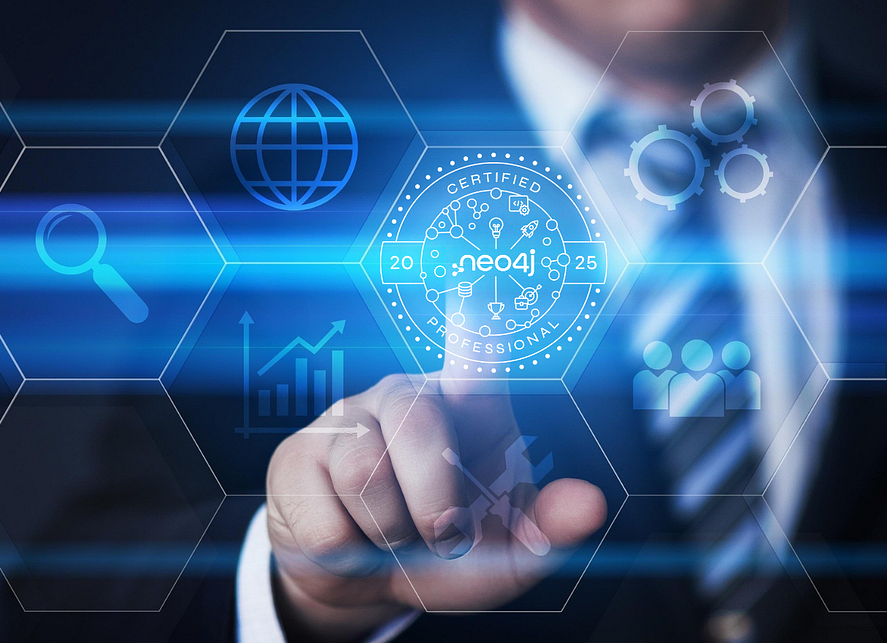
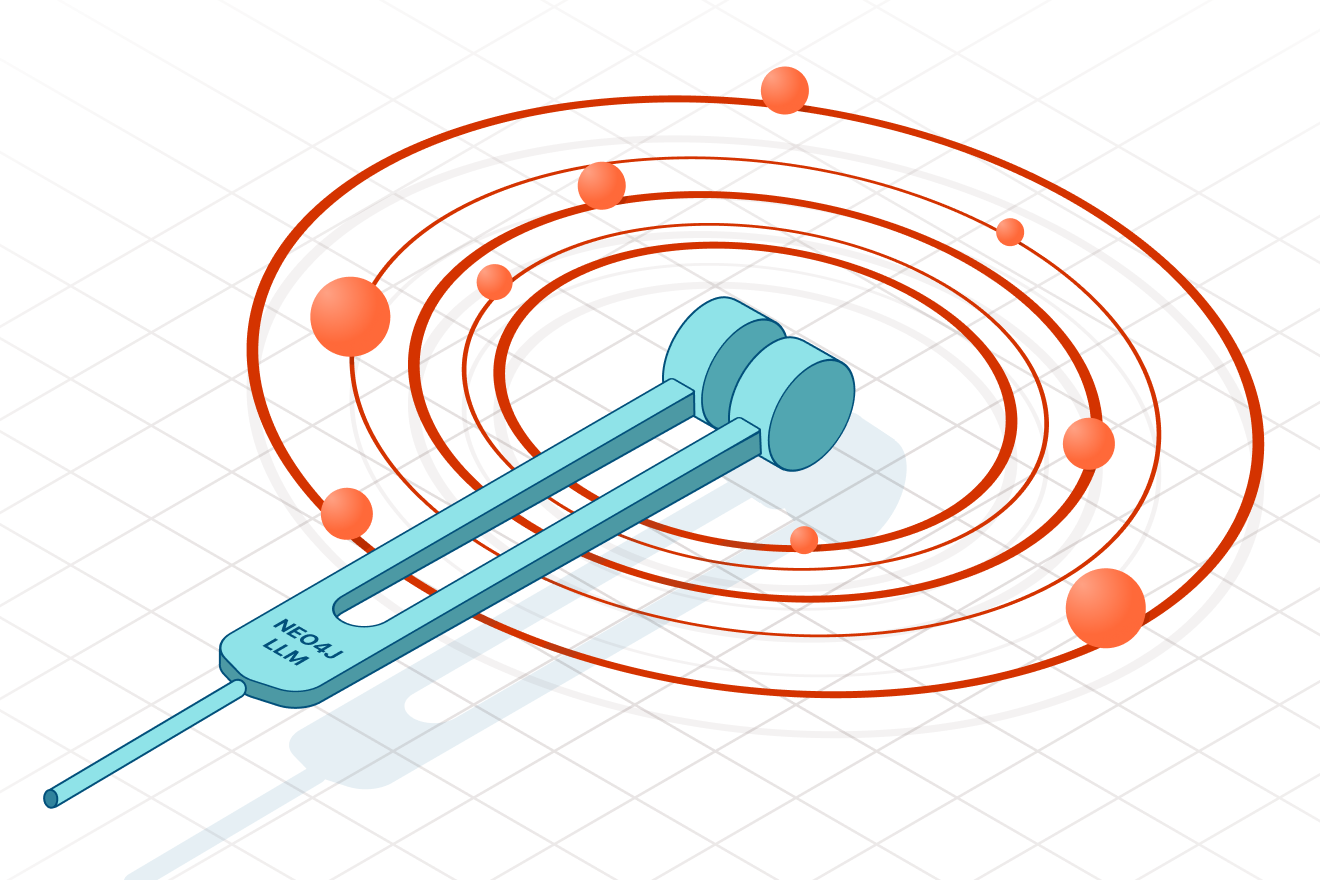
How to Improve Multi-Hop Reasoning With Knowledge Graphs and LLMs
9 min read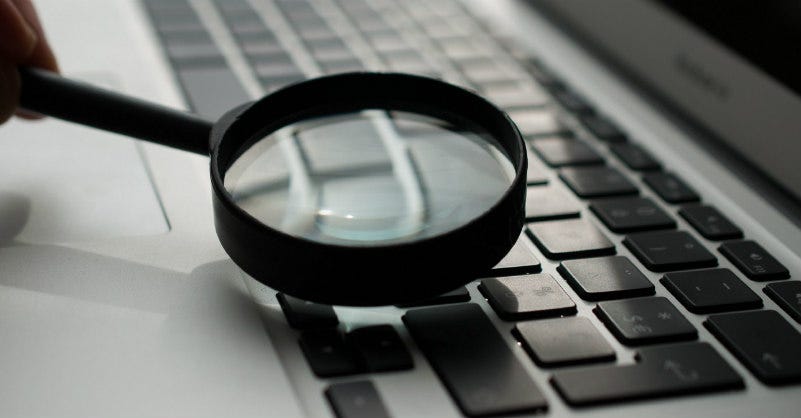
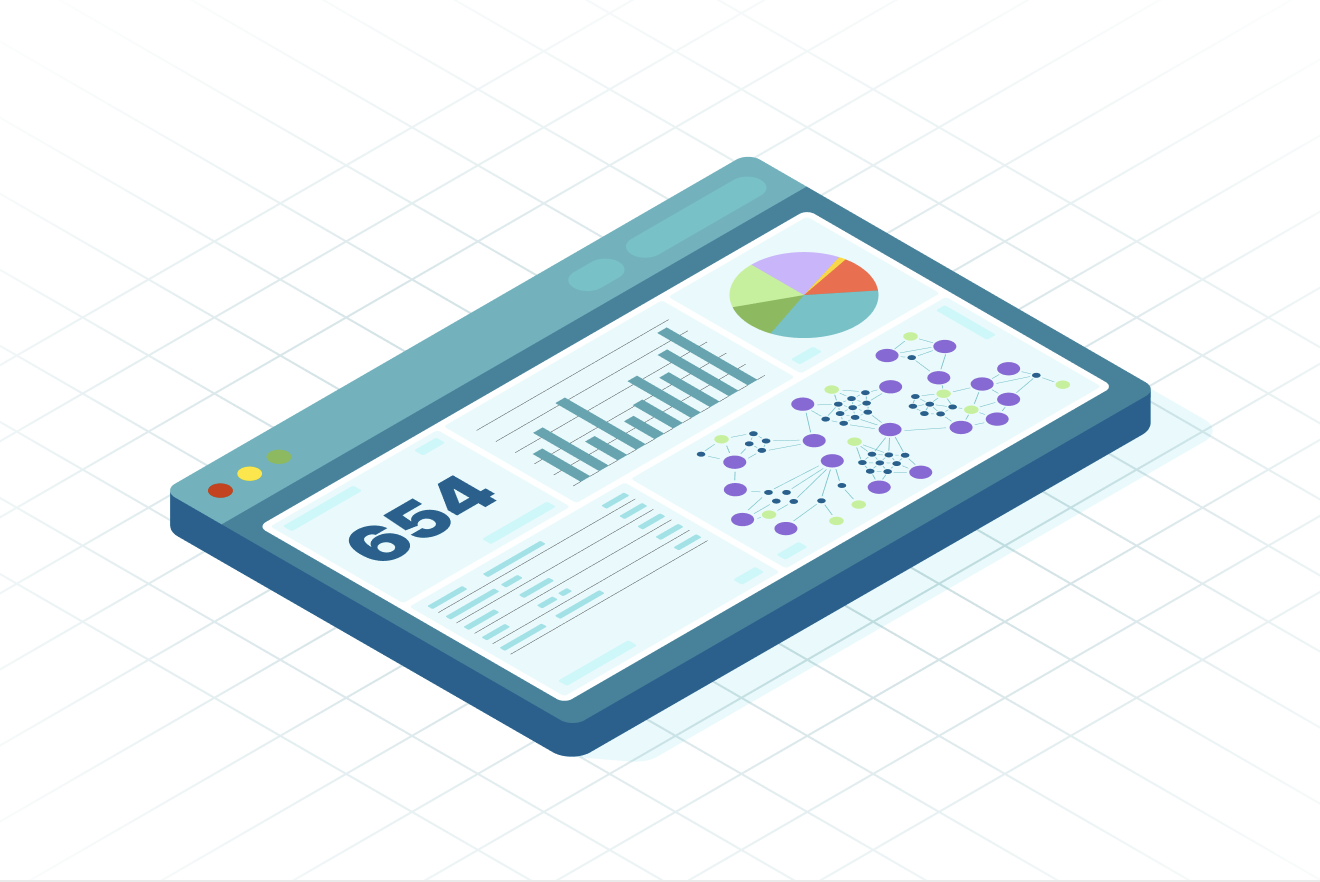