Engaging in a conversation with a company’s AI assistant can be frustrating. Chatbots give themselves away by returning generic responses that often don’t answer the question.
This doesn’t have to be the case. Imagine a different scenario: interacting with a chatbot that provides detailed, precise responses. This chatbot sounds like a human with deep institutional knowledge about the company and its products and policies. This chatbot is actually helpful.
The second scenario is possible through a machine-learning approach called Retrieval-Augmented Generation (RAG).
RAG is a technique that enhances Large Language Model (LLM) responses by retrieving source information from external data stores to augment generated responses.
These data stores, including databases, documents, or websites, may contain domain-specific, proprietary data that enable the LLM to locate and summarize specific, contextual information beyond the data the LLM was trained on.
RAG applications are becoming the industry standard for organizations that want smarter generative AI applications. This blog post explores RAG architecture and how RAG works, key benefits of using RAG applications, and some use cases across different industries.
Why RAG Matters
Large language models (LLMs), like OpenAI’s GPT models, excel at general language tasks but have trouble answering specific questions for several reasons:
- LLMs have a broad knowledge base but often lack in-depth industry- or organization-specific context.
- LLMs may generate responses that are incorrect, known as hallucinations.
- LLMs lack explainability, as they can’t verify, trace, or cite sources.
- An LLM’s knowledge is based on static training data that doesn’t update with real-time information.
To address these limitations, businesses turn to LLM-enhancing techniques like fine-tuning and RAG. Fine-tuning further trains your LLM’s underlying data set while RAG applications allow you to connect to other data sources and retrieve only the most relevant information in response to each query. With RAG, you can reduce hallucination, provide explainability, draw upon the most recent data, and expand the range of what your LLM can answer. As you improve the quality and specificity of its response, you also create a better user experience.
How Does RAG Work?
At a high level, the RAG architecture involves three key processes: understanding queries, retrieving information, and generating responses.
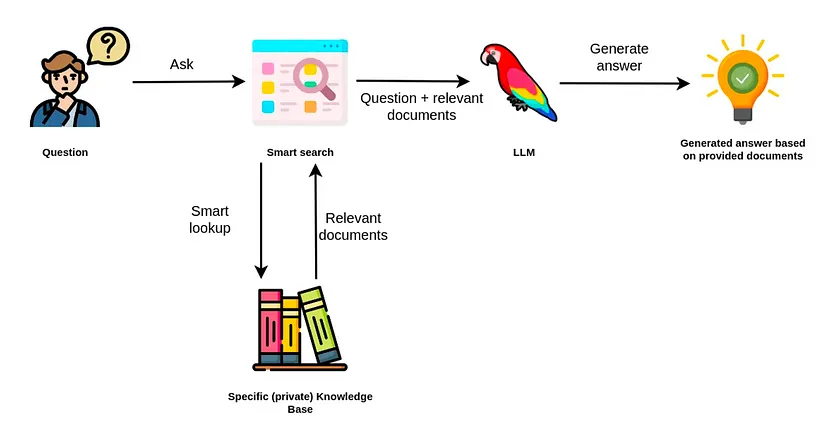
Before implementing an RAG application, it’s important to clean up your data to make it easy for the RAG application to quickly search and retrieve relevant information. This process is called data indexing.
Frameworks like LangChain make it easy to build RAG applications by providing a unified interface to connect LLMs to external databases via APIs. Neo4j vector index on the LangChain library helps simplify the indexing process.
1. Understanding User Queries
The process begins when a user asks a question. The query goes through the LLM API to the RAG application, which analyzes it to understand the user’s intent and determine what information to look for.
2. Information Retrieval
The application uses advanced algorithms like vector similarity search to find the most relevant pieces of information in the company’s database. These algorithms match vector embeddings based on semantic similarity to identify the information that can best answer the user’s question.
3. Response Generation
The application combines the retrieved information with the user’s original prompt to create a more detailed and context-rich prompt. It then uses the new prompt to generate a response tailored to the organization’s internal data.
What Are the Benefits of RAG?
Out-of-the-box generative AI models are well-equipped to perform any number of tasks and answer a wide variety of questions because they are trained on public data. The primary benefit of using a RAG application with an LLM is that you can train your AI to use your data—and this data can change based on what’s most relevant and current. It’s just a matter of which data stores are accessed and how often the data within them is refreshed. RAG also allows you to access and use custom data without making it public. Overall, RAG allows you to provide a generative AI experience that is personalized to your industry and individual business while solving for the limitations of a standalone LLM:
- Increased Accuracy: RAG applications provide domain-specific knowledge and enhanced reasoning, significantly reducing the risk of hallucinations.
- Contextual Understanding: RAG applications provide contextual responses based on proprietary, internal data across your organization, from customer info to product details to sales history.
- Explainability: By grounding responses in a source of truth, RAG applications can trace and cite information sources, increasing transparency and user trust.
- Up-to-date Information: As long as you keep your Graph Database or other document stores updated, RAG applications access the latest data in real time, allowing for continuous improvement.
What Are Common RAG Use Cases?
RAG enhances GenAI applications to interpret context, provide accurate information, and adapt to user needs. This enables a wide range of use cases:
- Customer Support Chatbots: With product catalogs, company data, and customer information at its fingertips, RAG chatbots can provide helpful, personalized answers to customer questions. They can resolve issues, complete tasks, gather feedback, and improve customer satisfaction.
- Business Intelligence and Analysis: RAG applications can provide businesses with insights, reports, and actionable recommendations by incorporating the latest market data, trends, and news. This can inform strategic decision-making and help you stay ahead of the competition.
- Healthcare Assistance: Healthcare professionals can use RAG to make informed decisions using relevant patient data, medical literature, and clinical guidelines. For instance, when a physician considers a treatment plan, the app can surface potential drug interactions based on the patient’s current medications and suggest alternative therapies based on the latest research. RAG can also summarize the patient’s relevant medical history to help guide decisions.
- Legal Research: RAG applications can quickly retrieve relevant case law, statutes, and regulations from legal databases and summarize key points or answer specific legal questions, saving time while ensuring accuracy.
As enterprises continue to generate ever-increasing amounts of data, RAG puts the data to work to deliver well-informed responses.
If you’re assessing your tech stack for generative AI models, the 2024 analyst report from Enterprise Strategy Group, Selecting a Database for Generative AI in the Enterprise, is a valuable resource. Learn what to look for in a database for enterprise-ready RAG applications and why combining knowledge graph and vector search is key to achieving enterprise-grade performance.