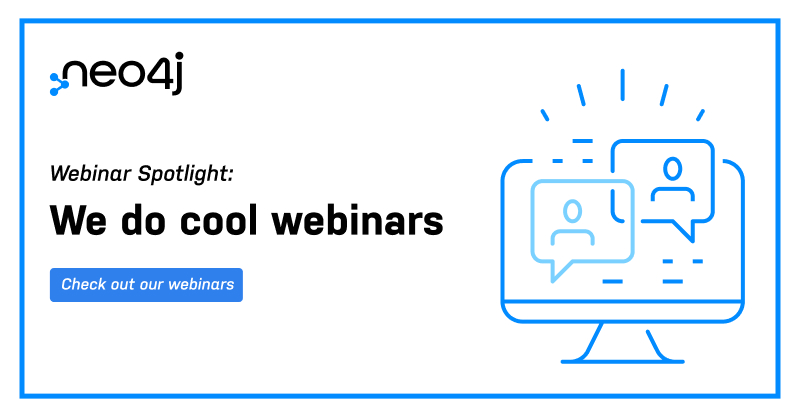
Crystal balls, tarot cards, fortune tellers, Groundhog Day – all things used to make predictions. The problem is, they’re not very reliable. But you know what is reliable? Graph data science.
Graph data science is a science-driven approach to gain knowledge from the relationships and structures in data, typically to power predictions. It describes a toolbox of techniques that help data scientists answer questions and explain outcomes using graph data.
Because today’s businesses are faced with extremely complex challenges and opportunities that require more flexible, intelligent approaches, Neo4j created the first enterprise graph framework for data scientists to improve predictions that drive better decisions and innovation.
Neo4j for Graph Data Science™ incorporates the predictive power of relationships and network structures in existing data to answer previously intractable questions and increase prediction accuracy.
To help you learn more about this, I’ve included replays to two webinars that focus on the key components of graph data science: relationships and link predictions.
The first webinar, Relationships Matter: Using Connected Data for Better Machine Learning, with Dr. Alicia Frame and Stuart Laurie, covers:
- Different approaches to graph feature engineering, from queries and algorithms to embeddings
- How ML techniques leverage everything from classical network science to deep learning and graph convolutional neural networks
- How to generate representations of your graph using graph embeddings, create ML models for link prediction or node classification, and apply these models to add missing information to an existing graph/incoming data
- Why no-code visualization and prototyping is important