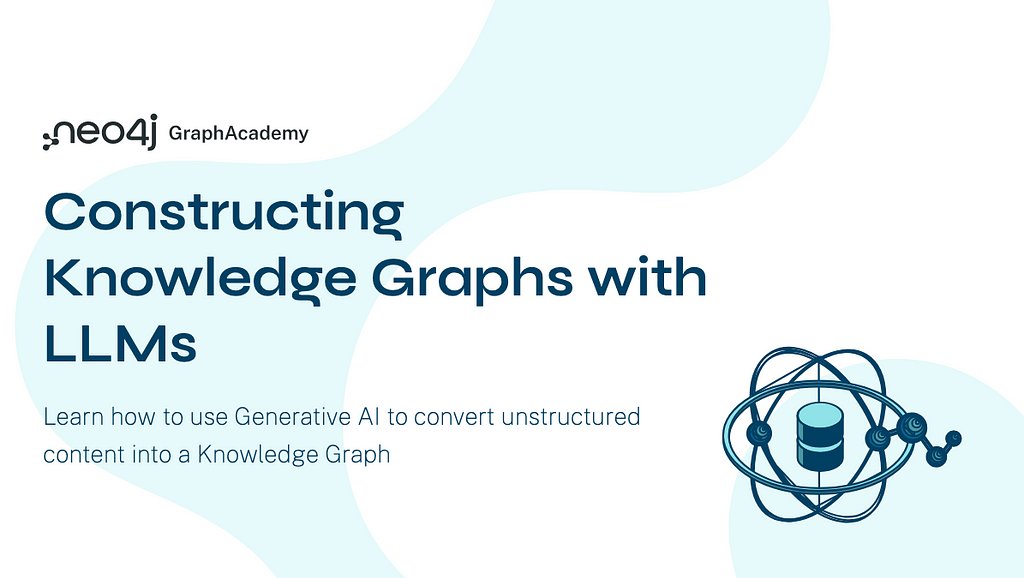
New GraphAcademy Course: Building Knowledge Graphs With LLMs
Oct 10 2 mins read
Learn how to use GenAI, LLMs, and Python to convert unstructured data into graphs. Read more →
Learn how to use GenAI, LLMs, and Python to convert unstructured data into graphs. Read more →
Learn to build accurate, explainable recommendation systems with minimal code using Neo4j graph database and Keymaker framework. Read more →
Learn how to overcome the challenges of structured data operations in text embeddings in RAG applications using knowledge graphs. Read more →
Knowledge graphs can be the secret ingredient to better supplier relationships and cost efficiency. Read more →
Learn how combing vectors and graphs boosts AI’s ability to reason, helping uncover deeper insights and create smarter queries for complex data. Read more →
Create a Neo4j GraphRAG workflow using LangChain and LangGraph, combining graph queries, vector search, and dynamic prompting for advanced RAG. Read more →
Integrate Microsoft's GraphRAG with Neo4j, using LangChain and LlamaIndex for advanced retrieval in just a few steps. Explore detailed code examples. Read more →
Learn how to use unstructured.io for PDF document parsing, extracting, and ingestion into the Neo4j graph database for GenAI applications. Read more →
Learn how to combine text extraction, network analysis, and LLM prompting and summarization for improved RAG accuracy. Read more →
Learn how to build a GraphRAG application almost entirely in Cypher, using knowledge graphs and vector search in Neo4j. Read more →
Learn how to perform entity deduplication and custom retrieval methods using LlamaIndex to increase GraphRAG accuracy. Read more →
Extract and use knowledge graphs in your GenAI applications with the LLM Knowledge Graph Builder in just five minutes. Read more →
The Neo4j GraphRAG Ecosystem Tools make it easy to develop GenAI applications grounded with knowledge graphs. Read more →
Neo4j, LLM creators, RAG orchestrators, knowledge graph designers, researchers, and deep thinkers gathered in San Francisco Presidio to explore GenAI. Read more →
Learn how we build a context-rich chatbot to answer Mahabharata questions using knowledge graphs and retrieval-augmented generation (RAG). Read more →
Learn how graph and vector search systems can work together to improve retrieval-augmented generation (RAG) systems. Read more →
Introducing the Neo4j LangChain Starter Kit for Python developers, which generates GenAI answers backed by data stored in a Neo4j Graph Database. Read more →
Part 2 of analyzing the Mahabharata's connections with knowledge graphs and building a chatbot using Google Gemini to answer questions. Read more →
In the 27 episodes of our Going Meta livestream series, Jesús Barrasa and I explored the many aspects of semantics, ontologies, and knowledge graphs. Read more →
Learn how to create knowledge graphs easily by turning PDF documents into graph models using LlamaParse for better RAG applications. Read more →
Learn how to extract topics from documents with graph data science and use them as the basis for semantic search for better RAG applications. Read more →
Optimizing vector retrieval with advanced graph-based metadata filtering techniques using LangChain and Neo4j. Read more →
A guide to building LLM applications with the Neo4j GenAI Stack on LangChain, from initializing the database to building RAG strategies. Read more →
What LLM & Graph May Bring to the Future of Knowledge GraphsNight light at Federation Square in Melbourne, photo by AuthorAbstractIn the last several decades, when people consider building a knowledge-related solution, they tend to look into two distinct directions based… Read more →
In this blog post, we will explore extracting information from unstructured data to construct a knowledge graph. Read more →