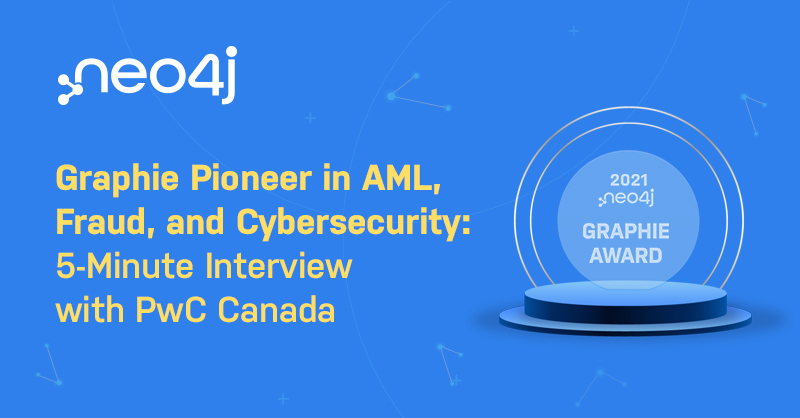
We were delighted to honor PwC Canada with a Graphie Award for being a Pioneer in AML, Fraud, and Cybersecurity, and had the pleasure of sitting down with Sam Samod, Partner, Financial Crime Technology at PwC, to discuss his work and the win.
Sam revealed some of his on-the-ground insights into graph technology, spoke to some of the existing challenges and solutions they’ve encountered in the fraud detection space, and hinted to some exciting things he sees on the horizon for connected data.
Enjoy hearing from a leading expert in the Neo4j community!
How are you using Neo4j?
Sam Samod: So we use Aura currently to prepare our data. Then we use Bloom for visualizations. Given Aura is the software of service platforms, we were able to get on it very quickly. Our technology team didn’t have to spend time installing or onboarding or procuring the software itself. Our initial use case was an application fraud where we developed an approach to detect synthetic ID.
There are a lot of fake profiles. Through our experiments, we know for a fact that it’s relatively easy to create fake profiles. You go to an online banking website and apply for a credit card, as an example. So from there, we know for sure that a lot of financial institutions have not implemented any effective controls to monitor any synthetic ID coming through.
How were you solving that problem before Neo4j?
Sam Samod: In the past, we’ve been using a relational database and legacy system. It’s very difficult to correlate data from multiple sources. So when you think of relational database joins, it is very time consuming to join information from one table to another table and then correlate data. It’s very heavy on internal system resources so we cannot oftentimes do things in real time – or the best we can do is perhaps near real time.
But with the acceleration of digital banking, I think more and more of our processes will become real time. And that’s where we need to have the ability to correlate data effectively and quickly. That’s where we find a graph database is a lot more effective in that sense. When we introduce a new piece of information, the system automatically establishes additional context of the information that we already have, which enhances insights for what we’re trying to do.
What made you choose Neo4j?
Sam Samod: When I first got to learn Neo4j, I found the way it works is very similar to how our brains work. Every piece of information provides additional context. So in the past, if you played those games where, every time you open up our little tile, the picture behind the tiles becomes clearer. I think that’s the analogy that I use for Neo4j, so every piece of information provides additional context and details inside that we can draw upon.
So when you think of our brain, every piece of information that we learn, we gain more knowledge from there. So this is where it’s not like the structured database, where for every piece of information you have to look at the entire role to understand what the information is trying to say to us.
Trying to correlate that information with another piece of information is very time consuming. But with Neo4j, every piece of information that we introduce into the graph itself automatically creates that additional context and contextual layer.
What have been some surprising results you’ve seen?
Sam Samod: I talk a lot about pictures. I think a picture is worth a thousand words. That is definitely true when we use a graph database and graph analytics. Oftentimes, the answer is found right in front of our eyes. We can’t see it because it’s all text and numbers, and it’s very hard for our brain to process and understand that information.
Since we use Bloom, we generate insights in a very short amount of time. This is where, from our own estimate, when we apply these to a financial crime investigation, as an example, we can cut down investigation time by 80 to 90 percent. The system goes out with fresh information, puts a graphical representation of that information, and the investigator is able to see it and make decisions quickly.
In the past, that process took a very long time. Oftentimes investigators spend four to five hours just going and gathering information from multiple sources and then trying to put all that together. With Bloom, the system automatically does that for them.
What is your favorite part about working with Neo4j?
Sam Samod: I think data discovery, because we let data tell us stories. We don’t start off something with a preconceived notion or prejudice. When we build models oftentime there’s a lot of bias that is built into it as well. Talking about models in the past – we used to model to generate alerts and red flags, especially for financial crime. Hence, everything looks the same.
I think that’s the root cause of why most banks and financial institutions are struggling with the false positives. We were bombarded with so many alerts and then those alerts all looked the same because they come from the same model. We talk about machine learning, we talk about AI, but all of them at the end of the day are model based with Neo4j. It’s a data-driven approach because you have to have a piece of information in order to connect it to something else. It is factual, so rather than make a hunch or a judgment on something, we have facts to back it up.
What do you think the future holds for graph technology?
Sam Samod: I think it’s huge. I think the opportunity is tremendous. We are data people. We have opportunities wherever we have data, or whenever we have data. What I do in financial crime can be applied to credit risk, to CRM, customer relationship management. My background is in credit risk and CRM. So I know for a fact that what we learn from, what we built for financial crime, would apply there as well.
You can imagine if we can detect bad people with high accuracy, we are even better at detecting good people. So when you think of consumers, they don’t change their behavior to avoid detections, unlike criminals, where they have to change their approach, change their methodologies, to avoid detection.
With consumers, we can move away from probability and high confidence to very high probability and very high confidence to target the good customers. That’s where I think there are a lot of opportunities, rather than pigeonhole ourselves into a competency like financial crime, CRM, or credit risk. Really, just go back to basics and look at data for what it is.
How do you think graph technology can serve cybersecurity and fraud detection?
Sam Samod: I think as I mentioned before, it’s factual, it’s unbiased. It’s real time. Right? So those are, I think, the three-pronged approach. We want to take an approach where we look at real data, we look at facts. We want to take away any bias or preconceived notions when we look at a case or investigate something, and of course real time, I think, is going to be the key for our survival going forward. We really need to move away from batch processing, into real time. Giving digital banking is coming, a real time payment is coming,
How did you feel when you found out you were being honored with a Graphie?
Sam Samod: I was happy, not for me, but for my team, because really it’s a team approach. I learned about Neo4j from an associate who came from my university and he came to me and said, “Hey, have you heard of Neo4j?” I’m like, “no.” That’s when we started looking at Neo4j.
When I heard about the technology, I actually went to your website. I put in the information and your team contacted me right away. That’s how our relationship with Neo4j started. From there we got training from you guys. Then more and more of our team members became certified in using the technology.
We have been using the tool for over a year now, and I’d like to use this opportunity to urge the industry to rethink our approach to financial crime prevention. I think with the recent news of the Taliban back in office, the Pandora Papers – clearly what we have been doing over the past 10 years isn’t working too well. So let me give you a simple example. So if we look at SWIFT wire and then if we apply Neo4j or a graph database on top of that data, we’ll gain so much insight in terms of the web of money flow from senders to receivers.
The challenge we have is that each financial institution only looks at data from their silo, right? In their own organizations. I think a consortium like SWIFT would be able to see the web of information, how the data or the money is flowing from one to the next. I think that will be the power where we leverage technology. Leveraging the consortium model on the wire transfer of KYC is another example where I think, KYC – rather than to “know your customer” should be “know your citizen.”
You know, this is where our consortium at the country level should be overseeing KYC profiles, because let’s say I bank at five different banks. Then my KYC will have three, five different profiles. I’m sure I have five different scores, but if I put it at the consortium level, they would be able to see that Sam has five different accounts or five different banks, and these are his profiles, and this is his behavior throughout.
So I think what we’ve been doing – and spending a lot of money on – is detecting wire fraud and detecting fake profiles in KYC, and it has not been working very well. And I think we need to revisit how we tackle this problem.
What is one main takeaway you have from introducing graph technology to your company?
Sam Samod: We want to be a data-driven organization, factual data. You know, we want to move away from hunch, from sentiment, and we want to have everything that’s factual and something to back it up. I think that’s where we build trust within our clients, and then for the services that we perform. I am part of forensic and what we do has always been very factual and data driven, but I want to put that even more so, in terms of applying technology as much as possible in everything that we do.
What is one graph trend you’re following in 2022?
Sam Samod: Open banking initiative. I think this is where graph technology would play a big role from credit application and adjudication to customer servicing and collections, right? So the gamut of the life cycle from bringing the customer into the organization – how do we service them and how do we collect money? When I think about graph technology, I think the day that we fill out the credit application, or online banking, that will go away.
We can literally approve credit on a customer without even seeing anything that customer provides. All they have to do is click consent. We have the ability to go out and fetch information from those clients using the open banking initiative, as an example, to go out and fetch data. We can approve them without even looking at the credit score, because I think credit score has a lot of bias.
For consumers who don’t borrow a lot of money, they tend not to have a very good credit score. This is where we can tap into the untapped market, where we use Neo4j, look at the cash flow, and then make a decision based on factual transaction data, rather than the information that consumers or customers provide.
Nominate a Graph Superstar for 2022